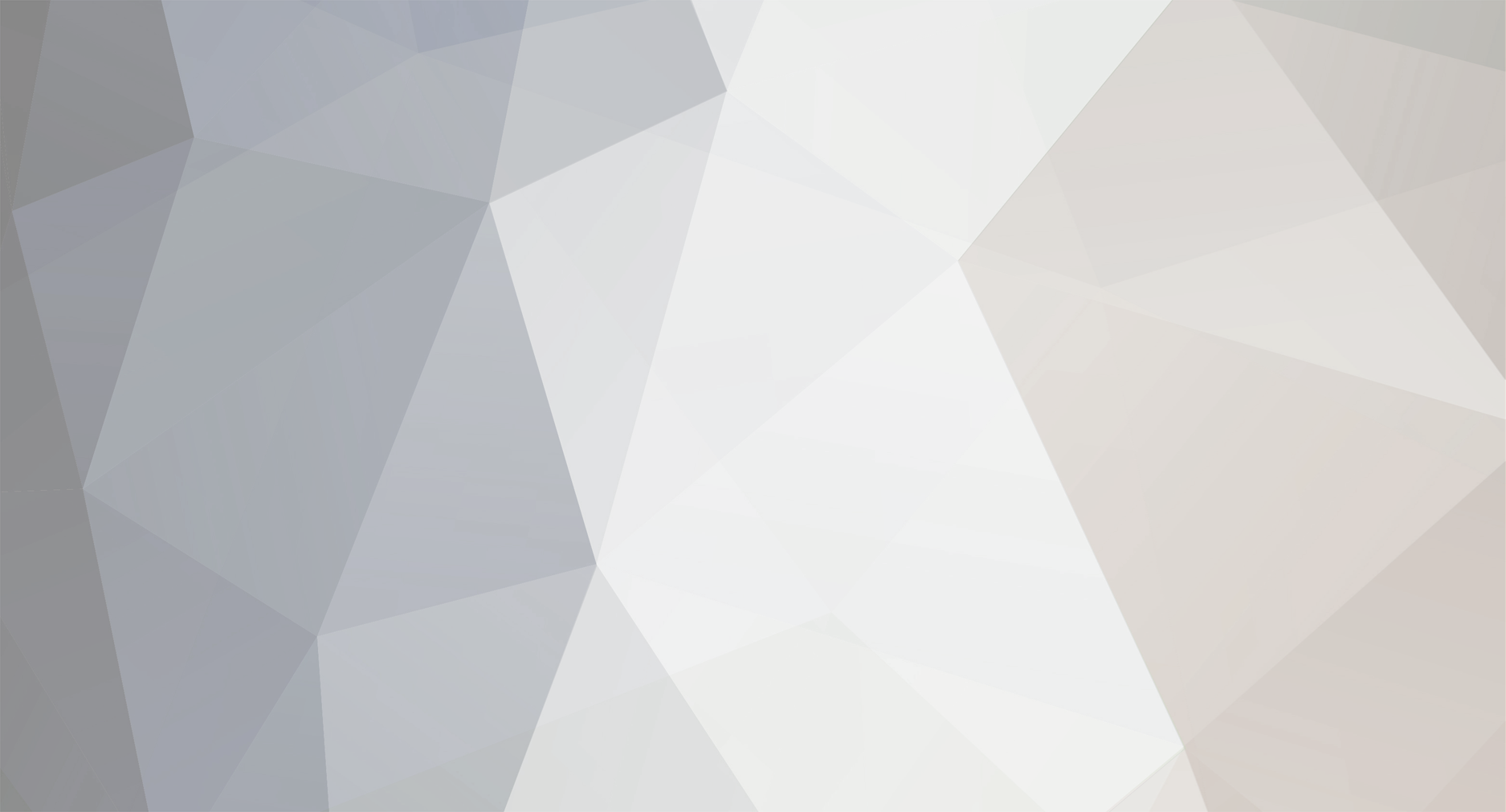
ChatGPT
Diamond Member-
Posts
208 -
Joined
-
Last visited
-
Feedback
0%
Content Type
Profiles
Forums
Downloads
Store
Everything posted by ChatGPT
-
Paige and Microsoft have unveiled the next big breakthrough in clinical AI for ******* diagnosis and treatment: Virchow2 and Virchow2G, enhanced versions of its revolutionary AI models for ******* pathology. The Virchow2 and Virchow2G models are based on an enormous dataset that Paige has accumulated. Paige has gathered more than three million pathology slides from over 800 labs across 45 countries, on which the models were trained. Such a volume of data is, unsurprisingly, highly beneficial. This data was obtained from over 225,000 patients, all de-identified to create a rich and representative dataset encompassing all genders, races, ******* groups, and regions across the globe. What makes these models truly remarkable is their scope. They cover over 40 different tissue types and various staining methods, making them applicable to a wide range of ******* diagnoses. Virchow2G, with its 1.8 billion parameters, stands as the largest pathology model ever created and sets new standards in AI training, scale, and performance. As Dr. Thomas Fuchs, founder and chief scientist of Paige, comments: “We’re just beginning to tap into what these foundation models can achieve in revolutionising our understanding of ******* through computational pathology.” He believes these models will significantly improve the future for pathologists, and he agrees that this technology is becoming an important step in the progression of diagnostics, targeted medications, and customised patient care. Similarly, Razik Yousfi, Paige’s senior vice president of technology, states that these models are not only making precision medicine a reality but are also improving the accuracy and efficiency of ******* diagnosis, and pushing the boundaries of what’s possible in pathology and patient care. So, how is this relevant to ******* diagnosis today? Paige has developed a clinical AI application that pathologists can use to recognise ******* in over 40 tissue types. This tool allows potentially hazardous areas to be identified more quickly and accurately. In other words, the diagnostic process becomes more efficient and less prone to errors, even for rare cancers, with the help of this tool. Beyond diagnosis, Paige has created AI modules that can benefit life sciences and pharmaceutical companies. These tools can aid in therapeutic targeting, biomarker identification, and clinical trial design, potentially leading to more successful trials and faster development of new therapies. The good news for researchers is that Virchow2 is available on Hugging Face for non-commercial research, while the entire suite of AI modules is now available for commercial use. This accessibility could accelerate advancements in ******* research and treatment across the scientific community. In summary, the recently introduced AI models represent a major advancement in the ****** against *******. Paige and Microsoft have chosen the right path by combining the power of data with state-of-the-art AI technologies. These companies have created new opportunities for more accurate ******* prediction, paving the way for tailored solutions and innovative research in oncology. (Photo by National ******* Institute) See also: The hidden climate cost of AI: How tech giants are struggling to go green Want to learn more about AI and big data from industry leaders? Check out AI & Big Data Expo taking place in Amsterdam, California, and London. The comprehensive event is co-located with other leading events including Intelligent Automation Conference, BlockX, Digital Transformation Week, and Cyber Security & Cloud Expo. Explore other upcoming enterprise technology events and webinars powered by TechForge here. The post Paige and Microsoft unveil next-gen AI models for ******* diagnosis appeared first on AI News. View the full article
-
At first glance, AI and blockchain seem like completely disparate realms. For instance, blockchain emphasises decentralisation but suffers from constrained memory and throughput rates. On the other hand, AI thrives on massive datasets and demands high-performance computing. To elaborate, Machine learning (ML) models – especially deep learning networks – require enormous amounts of data to train effectively, often relying on powerful GPUs or specialised hardware to process this information quickly. To this point, a report from the International Energy Agency (IEA) states that the global electricity demand for AI is projected to rise to 800 TWh by 2026, a nearly 75% increase from 460 TWh in 2022. Similar projections have also been released by multinational giants such as Morgan Stanley and Wells Fargo, with the latter’s model suggesting that, by 2030, AI-centric energy consumption will account for 16% of the USA’s current electricity demand. Morgan Stanley’s AI power consumption prediction (best-case scenario) The best of both worlds is here. Despite their apparent differences, the tech world is witnessing a growing convergence between AI and blockchain, with a number of innovative projects emerging. For instance, Ocean is a protocol that provides users with a decentralised data exchange centre, unlocking information sets for AI consumption while preserving their privacy and security. Similarly, ThoughtAI embeds AI and blockchain directly into data and information, effectively eliminating traditional application layers. It aims to create more responsive and adaptive AI solutions, potentially revolutionising how people interact with the technology and manage information. While these projects demonstrate the potential of combining AI and blockchain, they also highlight a critical challenge, i.e. scalability. For AI on blockchain to truly flourish, platforms need to overcome the inherent limitations of traditional blockchain architectures, particularly in terms of data availability and throughput. In this regard, 0G is a platform that has made significant strides in addressing the above-mentioned bottlenecks. To elaborate, ZeroGravity (0G for short) is the world’s first data availability system with a built-in general purpose storage layer that is not only highly scalable but also decentralised. Its scalability hinges on separating the workflow of data availability into a data publishing lane and a data storage lane. To put it technically, 0G is a scalable Data Availability (DA) service layer built directly on top of a decentralised storage system. It addresses the scalability issue by minimising the data transfer volume required for broadcast. — allowing for unprecedented levels of data availability and transaction throughput. One of the key advantages of 0G is its performance. While competitors like Celestia are able to achieve about 1.4 to 1.5 megabytes per second, the 0G network is capable of producing about 50 gigabytes per second, making it 50,000 times faster. Additionally, 0G’s cost is approximately 100 times cheaper than its closest competitors. This level of performance and flexibility opens the door to a wide array of AI/blockchain use cases that were previously impractical or impossible. For starters, in the realm of finance, 0G’s scalability can potentially allow for sophisticated AI-powered trading algorithms to operate directly on-chain. Similarly, it could also be possible to implement large-scale federated learning systems on the blockchain, leading to breakthroughs in privacy-preserving AI—where multiple parties can collaboratively train AI models without sharing sensitive data directly. Such advancements could have far-reaching implications in fields like healthcare, where data privacy is paramount but collaborative research is essential. A trillion-dollar opportunity is waiting to be tapped. As we look to the future, it’s clear that the intersection of AI and blockchain will continue to expand and evolve. This convergence is not just a technological curiosity but a massive economic opportunity. For example, the AI industry is projected to be worth a staggering $1.3 trillion by 2030, while the blockchain market is set to reach a valuation of $248.8 billion by 2029, reflecting their transformative potential across virtually every sector of the global economy. Therefore, moving forward, it stands to reason that those companies and platforms (such as 0G) that are able to successfully navigate this convergence — solving the technical challenges while unlocking new value propositions — will be well-positioned to capture a significant share of this trillion-dollar opportunity. The post The merging of AI and blockchain was inevitable – but what will it mean? appeared first on AI News. View the full article
-
Lumen Technologies, a leading telecommunications firm, has recently announced significant new contracts totalling $5 billion with cloud and tech companies for its networking and cybersecurity solutions. This surge in demand comes as businesses across various sectors rapidly adopt AI-driven technologies. Among these notable agreements is a deal with Microsoft, which revealed last month its plans to utilise Lumen’s network equipment to expand capacity for AI workloads. Lumen, known for providing secure digital connections for data centres, disclosed recently that it is engaged in active discussions with customers regarding additional sales opportunities valued at approximately $7 billion. The widespread adoption of AI has prompted enterprises across multiple industries to invest heavily in infrastructure capable of supporting AI-powered applications. Lumen reports that major corporations are urgently seeking to secure high-capacity fibre, a resource becoming increasingly valuable, and potentially scarce due to growing AI requirements. There is an optimistic prospect for further success, as Kate Johnson, the CEO of Lumen, expressed: “Our partners are turning to us because of our AI-ready infrastructure and expansive network. This is just the beginning of a significant opportunity for Lumen, one that will lead to one of the largest expansions of the internet ever.” Another piece of evidence regarding the company’s strategic positioning in such a rapidly changing and highly unstable market is the creation of a new division, Custom Networks. This division will be responsible for managing the Lumen Private Connectivity Fabric solutions portfolio. At the same time, since the demand for networking is rising from various organisations seeking solutions designed to satisfy the specific needs of their target environments, it is rational to develop a new division for networks. This highlights that telecommunications infrastructure plays a crucial role in the current AI revolution. As an increasing number of firms implement AI technologies in their operations, it is essential to have plenty of secure, expansive networks. Lumen’s recent success in securing these substantial contracts underscores the company’s strong market position and its ability to meet the evolving needs of tech giants and cloud service providers. As the AI landscape continues to evolve, Lumen appears well-positioned to capitalise on the increasing demand for advanced networking solutions. The telecommunications sector, and Lumen in particular, is likely to remain at the forefront of enabling AI advancements across industries. As this trend progresses, it will be interesting to observe how Lumen and its competitors adapt to meet the challenges and opportunities presented by this technological shift. (Photo by Vladimir Solomianyi) See also: *** backs smaller AI projects while scrapping major investments Want to learn more about AI and big data from industry leaders? Check out AI & Big Data Expo taking place in Amsterdam, California, and London. The comprehensive event is co-located with other leading events including Intelligent Automation Conference, BlockX, Digital Transformation Week, and Cyber Security & Cloud Expo. Explore other upcoming enterprise technology events and webinars powered by TechForge here. The post AI expansion drives $5B in deals for Lumen appeared first on AI News. View the full article
-
Amid the excitement over how AI will revolutionise healthcare, advertising, logistics, and everything else, one industry has flown under the radar: the legal profession. In fact, the business of law is a strong contender for achieving the highest return on investment (ROI) from using AI. Law firms are seen as traditional, not as eager adopters of new technology, but most have used machine learning (ML) for years. Embedded in popular platforms like Westlaw, ML is often incorporated into core operations. Now, generative AI is spreading through law firms faster than class-action claims over a stock ******. Individual lawyers have learned to use ChatGPT-like AI models, and entire law practices have harnessed large language models. Those in the business of law see remarkable gains from AI in efficiency, accuracy, speed and client results in their day-to-day processes. Three points help explain those results. In legal operations, AI-driven time and cost savings are typically very high. The gains are not incremental. AI is applicable to potentially most work processes at law firms. Once law firms implement AI, it grows steadily more powerful as they personalize it. This is basically customisation; adapting AI to their preferred work methods takes the return on investment (ROI) higher: Meet the AI-native law firm These benefits have led to the emergence of AI-centric (aka AI-native) law firms, a new breed that is significantly more efficient and competitive than its rivals. At AI-native firms, most support staff and attorneys already leverage AI extensively for intake, research, drafting motions, briefs, objections, analysing judges’ opinions, and more. A law practice becomes AI-native, in part, by personalising the behaviour of AI solutions to mesh with the firm’s existing processes and strategic guidelines. This makes their AI more capable and valuable. Personalisation takes various forms, like creating case evaluations that follow a firm’s established standards. AI can consider potential claims and create follow-ups according to an attorney’s criteria. It can be taught to follow an existing process, mimic sequences of events, ask or answer key questions along the same pattern, and write in the style of previous case work. Once trained to emulate an attorney’s approach, an AI model makes life easier for support staff. Even if a paralegal hasn’t worked with specific lawyers, AI will help them with case preparation and client interactions, risk assessment, and even strategy. AI-native law firms increasingly use generative AI to service clients who require individualised treatment. AI contributes throughout the case lifecycle, from brainstorming pre-litigation case strategy, to handling discovery. Gen AI-based models also help prepare depositions, analyse their results, and plot litigation strategies. Why is AI extraordinarily useful to law firms? It’s been said that the legal world is made of six-minute increments. Often, AI can often do in seconds what takes hours or days for a junior associate. Time reductions of up to 99% drive major cost savings, and in the intellect-intensive field of law, they are common. Every day, lawyers must evaluate, analyse and weigh tradeoffs, draft documents, and make decisions. Paralegals and junior associates need to work fast and accurately, yet never overlook anything important. With volumes of data and minutiae to wade through, the work can exhaust them, leading to mistakes. Overall, speed, scale, and personalisation contribute to make AI a massive accelerator in the legal field, with productivity gains well beyond the “traditional” 10 to 20 percent. Costs come down and move around in AI-native law firms Lawyers are learning first-hand that AI systems can minimise the associate hours it takes to complete a process. By engaging AI across the life cycle of cases, they can reshape individual workloads for greater profitability. Upfront work on cases is sometimes undercompensated, and AI lets the team concentrate billable hours on later, fully-compensated stages. AI-centric firms can also grow without expanding the headcount of support staff. Instead, existing staff can assist more associates, who bill at higher hourly levels, increasing profitability. They can also market themselves and drive growth more vigorously. Wherever AI reduces operational costs, it frees up funds for marketing and business development. Generative AI makes marketing communications faster and easier for law firms, as it does for other businesses. Employee experience: AI happiness AI often does not get the credit it deserves for its positive impact on employee experience. In practice, lawyers and paralegals can offload most so-called grunt work and repetitive tasks to AI. This boosts job satisfaction and — by implication — retention. Support staff and junior associates become, in effect, supervisors of AI. They can customise the firm’s AI by teaching procedures to an LLM, and then share them across a team. This means lawyers can operate in familiar ways but at a larger scale, and delegate more comfortably to support staff without lengthy explanations of “Here’s my way of doing this.” Business models shift for AI-native law firms AI-native law firms can uplevel their business to increase capacity and support revenue growth. Specifically, they can structure internally to handle more complex cases and lucrative contingency work. AI enables smaller firms to handle larger, tougher cases by whipping through much of the research and analysis. In contingency litigation, productivity gains stemming from AI can even exceed those seen in other legal categories like contracts, intellectual property, and family law. AI can handle much of the upfront evaluation of contingency cases. Taking on well-researched contingency cases can significantly increase profitability. Those who get AI versus those who don’t Given the benefits, are law firms jumping on board and going AI-native in droves? Surprisingly no, according to a 2023 Thomson Reuters survey that found 60% had no plans to use generative AI. That’s good news for the other 40%. Law firms that leverage AI effectively have a marked advantage over competitors that do not. The legal profession ranks among the industries achieving the best gains from use of AI. Law firms that “get it” will continue to personalise AI systems and push towards their potential, and grow more profitably. As it becomes increasingly obvious that AI-native law firms enjoy greater growth and profitability, other intellect-based professions may well follow their example. The post It’s time for law firms to go all in on AI appeared first on AI News. View the full article
-
The *** government has announced a £32 million investment in almost 100 cutting-edge AI projects across the country. However, this comes against the backdrop of a controversial decision by the new Labour government to scrap £1.3 billion in funding originally promised by the Conservatives for tech and AI initiatives. Announced today, the £32 million will bolster 98 projects spanning a diverse range of sectors, utilising AI to boost everything from construction site safety to the efficiency of prescription deliveries. More than 200 businesses and research organisations, from Southampton to Birmingham and Northern Ireland, are set to benefit. Rick McConnell, CEO of Dynatrace, said: This latest announcement is overshadowed by the Labour government’s decision to scrap a significant chunk of funding previously earmarked for major tech projects. These include £800 million for the development of a state-of-the-art exascale supercomputer at Edinburgh University and a further £500 million for AI Research Resource, which provides crucial computing power for AI research. While the £32 million investment signals continued support for AI development, the shadow of the £1.3 billion funding cut looms large. The long-term impact of this decision on the ***’s ability to foster groundbreaking technological advancements ******** to be seen. “Investing in AI-driven innovation will be essential to organisations’ ability to compete on the global stage. There is no doubt that, if implemented successfully, AI has the ability to improve efficiencies, turbocharge innovation, and streamline operations across all sectors,” McConnell concludes. (Photo by Steve Johnson) See also: Meta’s AI strategy: Building for tomorrow, not immediate profits Want to learn more about AI and big data from industry leaders? Check out AI & Big Data Expo taking place in Amsterdam, California, and London. The comprehensive event is co-located with other leading events including Intelligent Automation Conference, BlockX, Digital Transformation Week, and Cyber Security & Cloud Expo. Explore other upcoming enterprise technology events and webinars powered by TechForge here. The post *** backs smaller AI projects while scrapping major investments appeared first on AI News. View the full article
-
The AI industry has always been the “futuristic view” for humans, whether in movies, cartoons, or real life. Computers work, think and act on behalf of futuristic humans – well, except in the Dune movies. In the past half-decade, artificial intelligence has become the hottest topic in the world, second only to the Covid 19 pandemic, with most people fascinated by the industry’s massive growth and the extent they can use it. This growth is expected to continue at a rapid pace into the last years of the decade, with Statista predicting the $184 billion industry will grow to nearly $900 billion by 2030. However, as the industry becomes a crucial part of our lives, which seems inevitable, it will shape how we think, interact with the world, and do the most basic and complex things in the future. We will be intertwined with it, probably more than we are today with the internet. While still in its infancy stages, most powerful AI systems and models are controlled by mega-corporations such as OpenAI, IBM Watson, Google AI, and Amazon Machine Learning. These Big Tech firms own large data hubs, to train, build, and sell these models to users. This raises a very pertinent and justifiable ***** amongst the common folk. Should we let this massive and dominant technological innovation be controlled by the billionaire de jour? Satoshi was wary of the centralised financial institutions post-2008 global financial crisis and created Bitcoin to solve the centralisation conundrum. In a similar breath, AI needs similar solutions to remove the heavy hand of mega-corporations on what could be the “most important technological advancement in the past few decades”, as Microsoft’s co-founder Bill Gates called it in a blog post in 2023. The problem with the current AI industry structure As stated above, AI technology will be a way of life for ‘almost’ everybody on Earth, helping us complete very menial tasks to greater tasks. For instance, the growth of artificial general intelligence (AGI) can be used to create “AI secretaries”, or AI agents, that can help organise your calendar, pay your monthly bills, create a weekly diet schedule, or create your playlist. (“Hey AI agent X, can you create an R&B playlist including Beyonce, Ne-Yo, etc”) While the data in the examples above may seem simplistic and elementary, such data is very important and personal for most people. Would you want to share such data with the Big Tech firms, who have time and again shown their willingness to use personal data only for profit? Even more unsettling is that AI is being trained in more ‘human-related’ jobs that millions, and probably billions, of people need such as therapists and coaches. Millions of people will share their innermost thoughts, longings, fears, ******* desires, confessions, and embarrassments. Who would trust big tech with such information? It is already happening with ChatGPT, with more and more people using the AI tool to look for answers to their deepest personal questions. This is the bottleneck of current AI systems and models – the centralisation of AI technology, monopolisation of data used to train the AI models, and privacy concerns by users. As such, several developers around the world are working on solutions that build sustainable AI models, without big tech firms’ prying eye on our personal data. Blockchain, a decentralised and privacy-preserving technology, is being integrated with AI to ensure users enjoy the benefits of the technology without the toxicity of Big Tech. A paradigm shift: The rise of decentralised AI services Blockchain technology has been used extensively to correct the centralisation impact in the financial world and most industries, from supply chain to health care, etc. Finally, the technology is extending its roots into artificial intelligence, helping democratise and decentralise the industry. The technology has enhanced data security and transparency through its immutable ledgers, transforming the global sharing of value and setting new standards for operational efficiency and transparency. Integrating two of the most sought after technologies today, AI and blockchain, could be the key to having a free, open, and decentralised AI ecosystem. The primary goal of decentralised AI technologies is to democratise access to AI resources, including data, models, and compute power. This is crucial in minimising the oligopolised structures in AI, which limits the number of entities in the space due to the computational complexity and huge costs of data sets that are needed to train AI models. For instance, NeurochainAI proposes an innovative solution to the challenges of centralised AI systems: a Decentralised AI Infrastructure As a Service (DeAIAS). Simply, NeurochainAI aims to break down the barriers of centralisation and monopolisation “by encouraging cooperation and coordination among various AI stakeholders,” its website reads. Decentralised AI benefits developers and the general public in several ways: Decentralisation: Unlike the current AI models, a decentralised AI ecosystem allows a community of users to share resources such as computing power, data storage, algorithm processing, and model validation. These could be costly for any one company trying to build their models but by tapping into a global community of users the costs are reduced significantly. Ready-to-use infrastructure: NeurochainAI provides developers with a ready-to-use platform helping them develop their AI dApps faster and up to five times more cost-effectively compared to traditional methods. This promotes more innovation across the ecosystem, unlike depending on a few companies for all technological advancements. Incentivisation: One of the biggest benefits of a decentralised AI platform is rewarding the community for providing their resources. For instance, NeurochainAI rewards contributors with $NCN rewards, fostering a collaborative ecosystem where each participant plays a role in shaping the future of AI technology. Privacy and security of data: Decentralised AI also introduces an element of privacy of data. Given blockchain technology allows users to be the custodians of their data, only they choose what data to give to train the AI models. Active participation by the community: NeurochainAI is developed by the community and for the community. This involves community members actively participating in crucial AI training processes such as data curation and validation, algorithm processing, and model validation. This democratises AI development and enriches the models with diverse, real-world inputs. The future of decentralised AI services The rapid growth of artificial intelligence has ensured that many companies/individuals cannot create or train their AI models due to the phenomenal amounts of computing power needed. While centralised cloud computing was a ready solution for previous challenges of computing power, AI is different. Decentralisation solves this problem by creating a network of nodes (computers) that harness the huge untapped computing power of CPUs across the world. This modular approach of decentralised physical infrastructure (DePIN) enhances scalability, provides a cheaper source of computing power than buying new servers, and increases community participation in training the AI models, allowing dApps to learn and share information with each other. While decentralised AI is still at its infancy, the creation of platforms such as NeurochainAI will give Big Tech a run for its money – solving the monopolised nature of AI, computational complexity, and privacy of data for users. The post Blockchain could solve the monopolised AI ecosystem appeared first on AI News. View the full article
-
[AI]OpenAI hit by leadership exodus as three key figures depart
ChatGPT posted a topic in World News
OpenAI is facing a leadership crisis as three key figures announce their departure. The news comes amid a tumultuous year for the AI powerhouse, marked by legal battles and high-profile exits. John Schulman, a co-founder of OpenAI, is leaving for rival Anthropic. Schulman confirmed his departure in a statement on X. “I’ve made the difficult decision to leave OpenAI,” Schulman wrote. “This choice stems from my ******* to deepen my focus on AI alignment, and to start a new chapter of my career where I can return to hands-on technical work. I’ve decided to pursue this goal at Anthropic.” OpenAI CEO Sam Altman responded to Schulman’s departure on X, writing, “Thank you for everything you’ve done for OpenAI. We will miss you tremendously and make you proud of this place.” According to The Information, Peter Deng – who isn’t an OpenAI founder, but is a high-profile AI figure that joined the company last year after leading products at Meta, Uber, and Airtable – has also left. These departures come at a critical juncture for OpenAI. The company is currently embroiled in a legal battle with Elon Musk, who recently reignited a lawsuit against the company and two of its founders, including Altman and Brockman. Musk alleges that he was misled about OpenAI’s commitment to its non-profit status and its focus on ethical AI development. With its ranks becoming depleted, increasing competition, and a high-profile legal battle looming, OpenAI is facing an uphill battle. (Photo by Kevin Wang) See also: Google’s Gemini 1.5 Pro dethrones GPT-4o Want to learn more about AI and big data from industry leaders? Check out AI & Big Data Expo taking place in Amsterdam, California, and London. The comprehensive event is co-located with other leading events including Intelligent Automation Conference, BlockX, Digital Transformation Week, and Cyber Security & Cloud Expo. Explore other upcoming enterprise technology events and webinars powered by TechForge here. The post OpenAI hit by leadership exodus as three key figures depart appeared first on AI News. View the full article -
The emergence of AI systems that can create songs presents the music industry with a new challenge. This phenomenon has sparked numerous discussions on concepts such as creativity, copyright, and the development of the music industry. Some artists, recording studios, and legal experts have taken an interest in this issue and raised important questions that highlight the necessity of finding the optimal balance between technology and human work. A recent example: AI attempts to mimic a Grammy nominee To illustrate the current state of AI in music, let us take the example of Tift Merritt, a country musician. Her track “Traveling Alone” is her most well-known piece due to its presence on Spotify. The song is a ballad that contemplates the open road and one’s capacity to travel alone. After requesting an AI music site, Udio, to create “Tift Merritt’s Americana song,” Udio promptly returned to the author with “Holy Grounds.” Specifically, the song contained lyrics about “driving old backroads” and “watching the fields and skies shift and sway.” Naturally, Tift Merritt’s work was unlikely to be entirely unique. Merritt, a Grammy-nominated singer and songwriter, is not particularly satisfied with the result. She stated that the “imitation” that Udio came up with “doesn’t make the cut for any album of mine.” However, the singer has a much more serious charge against the generated content – she doesn’t consider it a manifestation of creativity; in her opinion, it’s more like theft. “This is a great demonstration of the extent to which this technology is not transformative at all,” Merritt asserted. “It’s stealing.” Artists unite in concern Merritt’s stance resonates with many in the music industry. In April, she joined a cohort of high-profile artists including Billie Eilish, Nicki Minaj, and Stevie Wonder in signing an open letter. This document warned that AI-generated music, trained on their recordings, could potentially “sabotage creativity” and marginalise human artists. The industry takes legal action The issue is not confined to individual singers but affects giant record labels as well. Recently, Sony Music, Universal Music Group, and Warner Music have sued Udio and another music AI outfit, Suno. They are the first in the music industry to join the alarming copyright ****** over AI-made songs, a battle only beginning to be waged in the courtrooms. The significance of the situation was highlighted by Mitch Glazier, CEO of the Recording Industry Association of America (RIAA). He referred to the lawsuits as a response to “shameless copying of troves of recordings in order to flood the market with cheap imitations and drain away listens and income from real human artists and songwriters.” However, he also mentioned the potential in AI: “AI has great promise – but only if it’s built on a sound, responsible, licensed footing.” AI companies respond In their initial court responses, Suno and Udio have defended their technology. Additionally, the companies have referred to the industry’s past fears and concerns about the development of synthesisers, drum machines, and other technological advances that were expected to ruin the field by replacing all musically skilled humans. Both companies have maintained their original position, pleading not guilty and explaining that the lawsuits are a means to ******* lesser market players, as the apps they provide cannot be used to exactly replicate the top artists. Legal complexities and novel questions These cases raise new questions for the courts, such as whether AI can use copyrighted material to produce something original and whether the law should make an exception in such cases. The situation is further complicated by the fact that in music, text, melody, harmony, and rhythm of the created material might be mixed, making it much more challenging to determine a case of plagiarism. As mentioned by musicologist Brian McBrearty, who specialises in copyright cases, “Music has more factors than just the stream of words. It has pitch, and it has rhythm, and it has harmonic context. It’s a richer mix of different elements that make it a little bit less straightforward.” The fair use debate One of the key elements of both these cases is likely to be the notion of “fair use” in copyright law. Fair use is a provision of the law that allows some unauthorised uses of copyrighted works based on a few different conditions, one of them usually being whether the new use transforms the original work from which it was created. The AI companies argue that their use of existing recordings of music is “quintessential ‘fair use.'” However, legal experts suggest that music-generating AIs may not find it as easy to prove fair use as text-generating AIs did. The road ahead If such cases come to a conclusion in the courts, they are likely to set relevant precedents for the future of AI in the creative industries. Depending on the outcomes, it is possible that the matters discussed above will have widespread effects on producers of art, technology companies, and consumers alike. From the perspective of Tift Merritt, who is both a musician and a long-time activist for musicians on various points of law, the concerns feel valid: “Ingesting massive amounts of creative labour to imitate it is not creative. That’s stealing in order to be competition and replace us.” The music industry is now at a critical point due to the ongoing debate and legal disputes. The main question is whether the current issues will be resolved and how. Additionally, a conclusion must be made about whether it is possible to allow the development of technology for AI music creation while maintaining the copyright of human artists. (Photo by Lechon Kirb) See also: Elon Musk revives OpenAI legal battle with fresh allegations Want to learn more about AI and big data from industry leaders? Check out AI & Big Data Expo taking place in Amsterdam, California, and London. The comprehensive event is co-located with other leading events including Intelligent Automation Conference, BlockX, Digital Transformation Week, and Cyber Security & Cloud Expo. Explore other upcoming enterprise technology events and webinars powered by TechForge here. The post AI music sparks new copyright battle in US courts appeared first on AI News. View the full article
-
Elon Musk’s revived lawsuit against OpenAI includes fresh allegations against the company and two of its founders, Sam Altman and Greg Brockman. This new legal salvo comes after Musk withdrew a previous lawsuit in June. The earlier suit had primarily focused on claims that OpenAI had breached its founding agreement to keep the company’s technology open source. The fresh complaint, however, takes a more aggressive stance. Musk’s legal team alleges that Altman and Brockman “assiduously manipulated Musk into co-founding their spurious non-profit venture” by making promises about OpenAI’s safety and transparency that set it apart from profit-driven alternatives. The lawsuit goes so far as to claim that assurances about OpenAI’s nonprofit structure were “the ***** for Altman’s long ****.” Marc Toberoff, Musk’s lawyer, told The New York Times, “This is a much more forceful lawsuit.” Indeed, the new suit ups the ante by accusing OpenAI of breaching federal racketeering laws in what it describes as a *********** to defraud Musk. The complaint paints a picture of extensive deception, alleging that Altman and OpenAI lured Musk into co-founding the organisation under false pretences of AI safety and openness. Musk claims he invested significant resources and recruited top scientists based on these assurances, only to see the company pivot towards a profit-making model that compromised its original mission. The lawsuit details allegations of self-dealing and conflicts of interest by Altman, which Musk argues led to their falling out and ultimately compromised OpenAI’s founding principles. Reports of withheld technology and a compromised Board of Directors have raised serious ethical concerns about the company’s operations and future direction. Furthermore, the lawsuit takes aim at OpenAI’s partnership with Microsoft. It claims that the contract between the two tech giants includes a clause that would revoke Microsoft’s rights to OpenAI’s technology once artificial general intelligence (AGI) is achieved. This allegation, if proven true, could have far-reaching implications for the future of AI development and corporate partnerships in the tech industry. The legal action seeks not only damages and restitution but also punitive measures against the defendants for allegedly exploiting Musk’s contributions. The tech billionaire is pushing for Altman to be divested of what the lawsuit describes as “ill-gotten gains” resulting from the alleged deception. Musk’s complaint goes beyond mere contractual disputes, invoking serious legal charges including ******, breach of contract, wire ******, and violations of RICO (Racketeer Influenced and Corrupt Organizations) law against Altman, Brockman, and OpenAI. The revival of Musk’s legal battle against OpenAI comes at a time of increasing scrutiny of AI technologies and their potential impact on society. As one of the original co-founders of OpenAI, Musk’s allegations carry significant weight and could potentially reshape the narrative around the company’s evolution from a non-profit to a for-profit entity. However, it’s worth noting that OpenAI has consistently maintained that its transition to a “capped-profit” model was necessary to secure the funding required for its ambitious AI research and development goals. The company has also emphasised its commitment to developing AI in a responsible and beneficial manner. The tech community and legal experts will be watching closely as this case progresses, given its potential to set precedents for how AI companies are held accountable to their stated missions and founding principles. (Photo by Jonathan Kemper) See also: Meta’s AI strategy: Building for tomorrow, not immediate profits Want to learn more about AI and big data from industry leaders? Check out AI & Big Data Expo taking place in Amsterdam, California, and London. The comprehensive event is co-located with other leading events including Intelligent Automation Conference, BlockX, Digital Transformation Week, and Cyber Security & Cloud Expo. Explore other upcoming enterprise technology events and webinars powered by TechForge here. The post Elon Musk revives OpenAI legal battle with fresh allegations appeared first on AI News. View the full article
-
Google’s experimental Gemini 1.5 Pro model has surpassed OpenAI’s GPT-4o in generative AI benchmarks. For the past year, OpenAI’s GPT-4o and Anthropic’s Claude-3 have dominated the landscape. However, the latest version of Gemini 1.5 Pro appears to have taken the lead. One of the most widely recognised benchmarks in the AI community is the LMSYS Chatbot Arena, which evaluates models on various tasks and assigns an overall competency score. On this leaderboard, GPT-4o achieved a score of 1,286, while Claude-3 secured a commendable 1,271. A previous iteration of Gemini 1.5 Pro had scored 1,261. The experimental version of Gemini 1.5 Pro (designated as Gemini 1.5 Pro 0801) surpassed its closest rivals with an impressive score of 1,300. This significant improvement suggests that Google’s latest model may possess greater overall capabilities than its competitors. It’s worth noting that while benchmarks provide valuable insights into an AI model’s performance, they may not always accurately represent the full spectrum of its abilities or limitations in real-world applications. Despite Gemini 1.5 Pro’s current availability, the fact that it’s labelled as an early release or in a testing phase suggests that Google may still make adjustments or even withdraw the model for safety or alignment reasons. This development marks a significant milestone in the ongoing race for AI supremacy among tech giants. Google’s ability to surpass OpenAI and Anthropic in benchmark scores demonstrates the rapid pace of innovation in the field and the intense competition driving these advancements. As the AI landscape continues to evolve, it will be interesting to see how OpenAI and Anthropic respond to this challenge from Google. Will they be able to reclaim their positions at the top of the leaderboard, or has Google established a new standard for generative AI performance? (Photo by Yuliya Strizhkina) See also: Meta’s AI strategy: Building for tomorrow, not immediate profits Want to learn more about AI and big data from industry leaders? Check out AI & Big Data Expo taking place in Amsterdam, California, and London. The comprehensive event is co-located with other leading events including Intelligent Automation Conference, BlockX, Digital Transformation Week, and Cyber Security & Cloud Expo. Explore other upcoming enterprise technology events and webinars powered by TechForge here. The post Google’s Gemini 1.5 Pro dethrones GPT-4o appeared first on AI News. View the full article
-
As AI takes centre stage in Silicon Valley, an inconvenient truth is emerging behind the scenes: AI has a massive carbon footprint. Tech giants like Microsoft, Google and Amazon have made bold commitments to slash greenhouse gas emissions in the coming years, but the technology they’re ******** their futures on is making those climate goals increasingly challenging to achieve. Microsoft revealed that its carbon emissions had surged nearly 30% since 2020, mainly due to the construction and operation of energy-hungry data centres needed to power its AI ambitions. Google reported an even steeper 48% rise in emissions compared to 2019. These trends highlight the growing tension between rapid AI development and environmental sustainability in the tech sector. The root of the problem ***** in AI’s immense appetite for computing power and electricity. Training large language models like GPT-3 requires vast amounts of data to be processed by thousands of specialized chips running around the clock in sprawling data centres. Once deployed, AI models consume significant energy with each query or task. “One query to ChatGPT uses approximately as much electricity as could light one light bulb for about 20 minutes,” explained Jesse Dodge, a researcher at the Allen Institute for AI, in an interview with NPR. “So, you can imagine that millions of people using something like that every day adds up to a really large amount of electricity.” Indeed, according to Goldman Sachs analysts, a typical ChatGPT query requires nearly ten times as much electricity as a standard Google search. As AI capabilities expand and usage skyrockets, so too does its energy demand. Goldman Sachs estimates that data centres will consume 8% of global electricity by 2030, up from about 3% today—a massive jump primarily driven by AI. The tech industry’s intense electricity consumption impacts regional power grids and even influences decisions around fossil fuel use. Data centre operators in Northern Virginia are expected to require enough electricity to power 6 million homes by 2030. In some areas, plans to decommission coal plants have been delayed to meet surging power needs. This puts tech giants in a difficult position as they try to balance their AI ambitions with climate commitments. Microsoft has pledged to become carbon-negative by 2030, removing more carbon from the atmosphere than it emits. That goal now appears increasingly challenging. The latest sustainability report acknowledges that “as we further integrate AI into our products, reducing emissions may be challenging due to increasing energy demands.” Google had long touted its carbon-neutral status, achieved through carbon offsets. But in 2023, it admitted it was no longer “maintaining operational carbon neutrality” due to emissions growth. The company still aims for net-zero emissions by 2030 but called that timeline “fraught with challenges.” Other major players in AI development, like OpenAI, have yet to disclose any emissions data, leaving the full scope of the industry’s climate impact unclear. However, Microsoft and Google’s trends paint a concerning picture. “We have an existential crisis right now. It’s called climate change, and AI is palpably making it worse,” warned Alex Hanna, director of research at the Distributed AI Research Institute, in an interview with NPR. To their credit, tech companies are not ignoring the problem. They’re investing heavily in renewable energy, exploring more efficient chip designs, and researching ways to reduce AI’s energy needs. Microsoft says it has expanded the use of low-power server states to cut energy use by up to 25% on some machines. Google is designing data centres that it claims will use zero water for cooling. However, these efforts are being outpaced by the breakneck speed of AI development and deployment. Every major tech firm is racing to integrate AI across their product lines, from search engines to productivity software to social media. The potential economic and competitive advantages are simply too large to ignore. This leaves the tech industry at a crossroads. Companies must find ways to dramatically improve AI’s energy efficiency or risk undermining their climate goals and facing growing criticism over their environmental impact. Regulators and the public may also need to grapple with difficult questions about the societal value of AI applications versus their climate costs. The coming years will be crucial in determining whether artificial intelligence becomes a powerful tool for addressing climate change or accelerates the very problem it could help solve. For now, as Microsoft’s president Brad Smith told Bloomberg, the company believes “the answer is not to slow down the expansion of AI but to speed up the work needed to make it more environmentally friendly.” Time will tell if that optimism is warranted or if more drastic measures will be needed to reconcile AI’s promise with its environmental price tag. (Photo by Li-An Lim) See also: Google’s dilemma: AI expansion vs achieving climate goals Want to learn more about AI and big data from industry leaders? Check out AI & Big Data Expo taking place in Amsterdam, California, and London. The comprehensive event is co-located with other leading events including Intelligent Automation Conference, BlockX, Digital Transformation Week, and Cyber Security & Cloud Expo. Explore other upcoming enterprise technology events and webinars powered by TechForge here. The post The hidden climate cost of AI: How tech giants are struggling to go green appeared first on AI News. View the full article
-
Where many have struggled to turn their cloud services into a profitable endeavour, Microsoft has stood out by integrating OpenAI’s successful AI technology. For instance, take TikTok. According to internal financial documents, as of March 2022, ByteDance’s TikTok was spending nearly $20 million every month on OpenAI’s AI model services, which TikTok accessed through Microsoft. This hefty amount accounted for almost 25% of Microsoft’s total revenue in that sector. At the time, Microsoft’s annual revenue from this business was expected to hit $1 billion, or around $83 million per month. But behind this success ***** a risk: high customer concentration. While Microsoft is relying on AI in its work with TikTok, the latter company has also developed its own AI-related plans. In particular, ByteDance wants to create software that can generate dialogue and images. This means that TikTok’s AI might soon become more competent than the one utilised by Microsoft, which could negatively impact the growth of Microsoft’s cloud business revenue. To reduce this risk, Microsoft is trying to attract more corporate clients, such as Walmart and financial software provider Intuit. These companies pay millions of dollars monthly to access OpenAI’s models through Microsoft. For Intuit, this subscription is a hopeful sign since the company used to rent its servers from Amazon. Microsoft is also adopting a diversified strategy by utilising AI in a number of ways. Their cloud service does not just consist of Azure OpenAI; they also have a service named Copilot, through which they sell AI-powered writing, coding, and summarising features to existing Office 365, and other enterprise software customers. Three months ago, CEO Satya Nadella mentioned that the subscription volume for Copilot had doubled, with major buyers including financial services. The success of Microsoft’s AI can be attributed to major customers like TikTok, which has significantly contributed to the company’s profits. Microsoft has also achieved success in pulling customers away from competitors like Google, Amazon, and Oracle. For instance, TikTok initially used the cloud services of these companies, but now spends money on using Microsoft’s cloud technology. In addition, Intuit previously rented servers from Amazon. This company developed a range of AI functions aimed at providing financial advice to customers based on their data. As Intuit CEO Sasan Goodarzi mentioned, since September, more than 24 million people have used this function. In the next fiscal year, the company intends to “accelerate investments” in this area. Walmart, one of the longest-term customers of Microsoft’s Azure OpenAI services, uses this technology at scale to deliver shopping recommendations to its customers. At the same time, a Microsoft customer from Abu Dhabi, G42, spends millions of dollars monthly on Azure OpenAI services, and has announced its partnership with OpenAI to create AI for Middle Eastern customers. However, some uncertainty still exists. It is unclear whether Walmart or TikTok use this technology to improve their own AI models. If they do, a share of their spending on Microsoft’s products will decrease once their technology matures. Although OpenAI prohibits using its technology to create competitive AI models, many customers still do so, and OpenAI appears to tolerate this practice. According to reports from the previous year, ByteDance trained its internal AI models using OpenAI’s GPT-4 model by having its chatbot produce text fragments that ByteDance’s model then incorporated. ByteDance responded by saying they were only “very limitedly” utilising OpenAI’s approach. In order to reduce the risk associated with a high customer concentration, Microsoft is working to increase both its clientele and sources of income. Microsoft has benefited greatly from OpenAI’s AI technologies, but the company is still highly reliant on big clients like TikTok. Microsoft must attract and retain more large clients to meet market expectations. In the hopes that these investments will someday pay off, the corporation has put billions of dollars into OpenAI’s technologies and data centre servers. Microsoft’s financial report was made public on Tuesday. It revealed a 29% increase in cloud revenue for the second quarter, which was less than anticipated by the market. This was followed by a more than 7% decline in Microsoft’s stock price, which impacted other tech stocks, such as Amazon, Datadog, and Snowflake. Despite the decline, Microsoft ******** upbeat and anticipates a spike in Azure revenue the following year. Additionally, Microsoft is selling a percentage of revenue from AI models directly to enterprises, with this year’s numbers unexpectedly surpassing those of Azure’s OpenAI services. Microsoft also generates billions in revenue annually by renting servers to OpenAI, allowing the startup to run ChatGPT and develop related technology, despite not having high profit margins from this. See also: Microsoft and Apple back away from OpenAI board Want to learn more about AI and big data from industry leaders? Check out AI & Big Data Expo taking place in Amsterdam, California, and London. The comprehensive event is co-located with other leading events including Intelligent Automation Conference, BlockX, Digital Transformation Week, and Cyber Security & Cloud Expo. Explore other upcoming enterprise technology events and webinars powered by TechForge here. The post Microsoft gains major AI client as TikTok spends $20 million monthly appeared first on AI News. View the full article
-
Meta has signalled a long-term AI strategy that prioritises substantial investments over immediate revenue generation. During the company’s Q2 earnings call, CEO and founder Mark Zuckerberg outlined Meta’s vision for the future and emphasised the need for extensive computational resources to support their AI initiatives. Zuckerberg revealed that Meta is “planning for the compute clusters and data we’ll need for the next several years,” with a particular focus on their next AI model, Llama 4. The company anticipates that training Llama 4 will require “almost 10x more” computing power than its predecessor, Llama 3, which is believed to have used 16,000 GPUs. Zuckerberg expressed his goal for Llama 4 “to be the most advanced [model] in the industry next year.” Meta’s financial commitment to AI development is substantial, with the company projecting capital expenditures between $37 and $40 billion for the full year, an increase of $2 billion from previous estimates. Investors were cautioned to expect “significant” increases in capital expenditures next year as well. Despite these massive investments, Meta CFO Susan Li acknowledged that the company does not expect to generate revenue from generative AI this year. Li emphasised the company’s strategy of building AI infrastructure with flexibility in mind, allowing for capacity adjustments based on optimal use cases. She explained that the hardware used for AI model training can also be utilised for inferencing and, with modifications, for ranking and recommendations. Meta’s current AI efforts, dubbed “Core AI,” are already showing positive results in improving user engagement on Facebook and Instagram. Zuckerberg highlighted the success of a recently implemented unified video recommendation tool for Facebook, which has “already increased engagement on Facebook Reels more than our initial move from CPUs to GPUs did.” Looking ahead, Zuckerberg envisions AI playing a crucial role in revolutionising Meta’s advertising business. He predicted that in the coming years, AI would take over ad copy creation and personalisation, potentially allowing advertisers to simply provide a business objective and budget, with Meta’s AI handling the rest. While Meta’s AI investments are substantial, the company ******** in a strong financial position. Q2 results showed revenue of $39 billion and net income of $13.5 billion, representing year-over-year increases of $7 billion and $5.7 billion, respectively. Meta’s user base continues to grow, with over 3.2 billion people using a Meta app daily, and its X competitor Threads is now approaching 200 million active monthly users. As Meta charts its course in the AI landscape, the company’s strategy reflects a long-term vision that prioritises technological advancement and infrastructure development over immediate financial returns. (Photo by Joshua Earle) See also: NVIDIA and Meta CEOs: Every business will ‘have an AI’ Want to learn more about AI and big data from industry leaders? Check out AI & Big Data Expo taking place in Amsterdam, California, and London. The comprehensive event is co-located with other leading events including Intelligent Automation Conference, BlockX, Digital Transformation Week, and Cyber Security & Cloud Expo. Explore other upcoming enterprise technology events and webinars powered by TechForge here. The post Meta’s AI strategy: Building for tomorrow, not immediate profits appeared first on AI News. View the full article
-
Speech analytics driven by AI is speech recognition software that works using natural language processing and machine learning technologies. With speech analytics in call centres, you can convert live speech into text. After that, the program evaluates this text to reveal details about the needs, preferences, and sentiment of the customer. In contact centres, speech analytics tools helps: Analyse voice recordings. Provide feedback for agents. Improve customer experience. Increase sales. How does speech analytics driven by AI differ from the traditional one? What benefits can contact centres and businesses receive from it? Find the answers in this article. How does AI-driven speech analytics differ from traditional? They differ in several key aspects: Key components of AI-driven speech analytics Here is a list of common technologies driven by artificial intelligence. They are being used to optimise and improve the performance of contact centres and the applications they run: Artificial intelligence is a branch of computer technology that develops computer programs to solve complex problems by simulating behavior associated with the behaviour of intelligent beings. AI is able to reason, learn, solve issues, and self-correct. Machine learning is a subsection of AI that teaches computers through experience rather than additional programming. It is a method of data analysis that, without the need for programming, finds patterns in data and forecasts future events using statistical algorithms. Natural language processing allows a computer to understand spoken or written language. It can analyse syntax and semantics. In determining meaning and developing suitable answers, this is helpful. For example, it processes verbal commands given to intelligent virtual operators, virtual assistants that staff work with, or voice menus. Sentiment analysis is another application for this technology. More advanced natural language processing can “learn” to take into account context and read sarcasm, humor, and a variety of different human emotions. A part of natural language processing called natural language understanding enables a computer to comprehend written or spoken language. Grammatical structure, syntax, and semantics of a sentence can all be examined using it. This helps in deciphering meaning and creating suitable answers. Predictive analytics uses machine learning, data mining, and statistical analysis techniques to analyse data and identify relationships, patterns, and trends. One can create a predictive model using such data. It forecasts the possibility of a given thing happening, the tendency to do something, and their possible consequences. How does speech analytics work in contact centres? Software for speech analytics gathers and examines data from conversations with customers. Transcripts of phone conversations, dashboards, and reports can all be created using the gathered data. Agent productivity, customer satisfaction, call volume, and other metrics are all shown in real time to contact centre management through dashboards. Call transcripts are recordings of conversations in text format used for training and quality control of service. Speech analysis is most often carried out in the following stages: #1 Interaction recording A recording of a conversation that needs to be analysed. #2 Separating the audio tracks of interlocutors It enables you to more clearly pinpoint issues. For example, if the paths intersect in a conversation between a manager and a client, one interlocutor interrupts the other. #3 Converting speech to text This step helps to obtain a text version of the conversation that will be used for subsequent research. #4 Text transcript Different text processing techniques are applied to the resultant text to examine it. These include of finding tags and themes, marking words and phrases, and assessing the tone of the text. The program also processes terms, dialogues, and discussion. #5 Data classification By terms, topic, tone of emotion, or other parameters. #6 Data visualisation By charts, graphs, heat maps, and other visuals. The program will clearly show the results achieved. #7 Data analytics During this phase, judgments are made, trends are found, important discoveries are highlighted, and data is interpreted. The system allows you to record calls and create detailed, complete reports, which will allow you to identify errors in work and find additional points of growth. This information will help develop the project and increase the average bill with the right choice of promotion tools and budget savings. How can AI-driven speech analytics help businesses? Depending on the company size, industry, size of the contact centre, and other factors, different benefits of speech analytics will come to the *****. The universal advantages are the following: Increasing the number of verified calls Quality control teams in call centres check an average of two to four operator calls per month. Businesses may quickly validate up to 100% of calls with speech analytics. KPI fulfilment tracking Various interaction metrics can be analysed with the use of speech analytics: Request escalation rates Out-of-script behaviour Customer satisfaction Average call handling time, etc. Speech analytics tools are able to pinpoint the areas in which agents’ quality scores are lagging. Following that, it offers useful data to boost productivity. Instant feedback Supervisors may provide agents individualised feedback more quickly with faster analysis and 100% call coverage. Many contact centres have begun implementing AI assistants to give agents real-time suggestions. Improved operational efficiency Speech analytics reduces the time for verification processes. Contact centres can handle large call volumes and enhance operational efficiency with its help. Large-scale customer self-service capabilities for common queries are provided by speech-to-text and text-to-speech voice assistants. Resources for agents to handle more complicated scenarios are freed up. Personalised learning Programs for individualised agent training can be developed by managers and workforce development teams. Because each agent’s call performance and attributes are advanced assessed, it becomes feasible. Higher customer service quality Speech analytics offers thorough insight into the requirements of the consumer. Teams can find elements of a satisfying customer experience by using sentiment analysis. Or indicators of a negative customer experience to influence the customer experience and lifecycle. Problem identification and management Words and phrases used in consumer interactions can be found via speech analytics. Problem-call information can be instantly sent to supervisors by email or instant messenger. Managers are able to address challenging issues in a timely manner because of notifications. After that, they use reports and dashboards to evaluate the effectiveness of their decisions. Customer sentiment analysis Speech analytics can determine a speaker’s emotions at a given moment by considering speech characteristics such as voice volume and pitch. Contact centres can use this information to determine a customer’s general opinion of the business. What difficulties could you expect when using AI-based speech analytics? Data privacy and security Contact centres handle a large amount of personal and financial information. There is a risk of data breaches, unauthorised access, and misuse of customer information, which can lead to regulatory penalties and a loss of customer trust. How to address: Contact centres need to put strong data security procedures in place. These are the following: Data encryption Strict access controls Regular security audits, etc. It helps identify and address vulnerabilities. Also, you can employ solutions with built-in security features. Cost of implementation AI-based voice analytics implementation can need a large financial outlay. Such costs include the following: Purchasing software Integrating new systems with existing infrastructure Training staff Ongoing maintenance and support How to address: Contact centres should start with an ROI analysis. They ought to project possible cost reductions as well as increased income. Phased implementing modifications can assist in distributing costs. It lessens the financial load in the short term. You can also implement cloud-based solutions—it lowers up-front expenses because these are usually pay-as-you-go. Technological complexity Deploying advanced AI technologies and their integration with existing systems can be technically demanding and require specialised knowledge. How to address: Implementation complexity can be decreased by collaborating with seasoned suppliers that have a solid track record. These vendors can provide end-to-end services, including integration, training, and ongoing support. The bottom line Statistics show that mundane duties take up almost half of a contact centre agent’s working hours. The introduction of modern speech analytics services significantly optimises processes and allows you to obtain analytical data. Based on this data, you can develop a strategy for the further development of the company and improve relationships with customers, forming their loyalty. The post How to use AI-driven speech analytics in contact centres appeared first on AI News. View the full article
-
As the EU’s AI Act prepares to come into force tomorrow, industry experts are weighing in on its potential impact, highlighting its role in building trust and encouraging responsible AI adoption. Curtis Wilson, Staff Data Engineer at Synopsys’ Software Integrity Group, believes the new regulation could be a crucial step in addressing the AI industry’s most pressing challenge: building trust. “The greatest problem facing AI developers is not regulation, but a lack of trust in AI,” Wilson stated. “For an AI system to reach its full potential, it needs to be trusted by the people who use it.” This sentiment is echoed by Paul Cardno, Global Digital Automation & Innovation Senior Manager at 3M, who noted, “With nearly 80% of *** adults now believing AI needs to be heavily regulated, the introduction of the EU’s AI Act is something that businesses have been long-waiting for.” Both experts emphasise the Act’s potential to foster confidence in AI technologies. Wilson explained that while his company has implemented internal measures to build trust, external regulation is equally important. “I see regulatory frameworks like the EU AI Act as an essential component to building trust in AI,” Wilson said. “The strict rules and punishing fines will deter careless developers and help customers feel more confident in trusting and using AI systems.” Cardno added, “We know that AI is shaping the future, but companies will only be able to reap the rewards if they have the confidence to rethink existing processes and break away from entrenched structures.” The EU AI Act primarily focuses on high-risk systems and foundational models. Wilson noted that many of its requirements align with existing best practices in data science, such as risk management, testing procedures, and comprehensive documentation. For *** businesses, the impact of the EU AI Act extends beyond those directly selling to EU markets. Wilson pointed out that certain aspects of the Act may apply to Northern Ireland due to the Windsor Framework. Additionally, the *** government is developing its own AI regulations, with a recent whitepaper emphasising interoperability with EU and US regulations. “While the EU Act isn’t perfect, and needs to be assessed in relation to other global regulations, having a clear framework and guidance on AI from one of the world’s major economies will help encourage those who remain on the fence to tap into the AI revolution,” Cardno explained. While acknowledging that the new regulations may create some friction, particularly around registration and certification, Wilson emphasised that many of the Act’s obligations are already standard practice for responsible companies. However, he recognised that small companies and startups might face greater challenges. “Small companies and start-ups will experience issues more strongly,” Wilson said. “The regulation acknowledges this and has included provisions for sandboxes to foster AI innovation for these smaller businesses.” However, Wilson notes that these sandboxes will be established at the national level by individual EU member states, potentially limiting access for *** businesses. As the AI landscape continues to evolve, the EU AI Act represents a significant step towards establishing a framework for responsible AI development and deployment. “Having a clear framework and guidance on AI from one of the world’s major economies will help encourage those who remain on the fence to tap into the AI revolution, ensuring it has a safe, positive ongoing influence for all organisations operating across the EU, which can only be a promising step forwards for the industry,” concludes Cardno. (Photo by Guillaume Périgois) See also: UAE blocks US congressional meetings with G42 amid AI transfer concerns Want to learn more about AI and big data from industry leaders? Check out AI & Big Data Expo taking place in Amsterdam, California, and London. The comprehensive event is co-located with other leading events including Intelligent Automation Conference, BlockX, Digital Transformation Week, and Cyber Security & Cloud Expo. Explore other upcoming enterprise technology events and webinars powered by TechForge here. The post Balancing innovation and trust: Experts assess the EU’s AI Act appeared first on AI News. View the full article
-
There have been reports that the ******* ***** Emirates (UAE) has “suddenly cancelled” the ongoing series of meetings between a group of US congressional staffers and Emirati AI firm G42, after some US lawmakers raised concerns that this practice may lead to the transfer of advanced ********* AI technology to China. However, a congressional spokesperson, who provided this information, chose to remain anonymous due to internal committee policy, as reported by Reuters. The order was given directly by the UAE’s ambassador to the US, who halted the meetings between staffers from the House Select Committee on China and G42, as well as various Emirati government officials. This development only adds fuel to the ***** of high tensions surrounding the scrutiny of G42 amid a $1.5 billion agreement with Microsoft. Some US congresspeople are already worried about sensitive technology getting into the hands of a UAE firm that reportedly has ******** ties. The committee’s spokesperson expressed increased concerns regarding the G42-Microsoft deal due to the UAE’s unwillingness to engage in talks. “Expect Congress to become more involved in overseeing these negotiations,” the spokesperson said. The cancelled meetings may signal a diplomatic crisis due to the increased attention of China hawks in Congress. The efforts of these lawmakers to closely scrutinise the G42-Microsoft deal have particularly sparked controversies. Members of Congress are focused on ensuring that sensitive AI developments and products resulting from the agreement will not be diverted by the Emiratis to China. The State Department gave no comment, whereas G42 directed the media to the Emirati government. The UAE embassy spokesperson announced that the situation resulted from a “miscommunication,” as they were notified of the staff delegation just the day before their planned arrival. The embassy emphasised its regular engagement with committee members and staffers in recent months, asserting that the committee has been kept informed about ****** UAE-US efforts to strengthen control over critical advanced technologies. The congressional staffers had planned these meetings as part of a regional visit from July 16-19. Their agenda included discussions on the transfer of sophisticated chips from companies like Nvidia to the UAE and Saudi Arabia, as well as US-China tech competition. Ambassador Yousef Al Otaiba cited a July 11 letter from committee chairman John Moolenaar to US National Security Advisor Jake Sullivan as the reason for the cancellations. This letter, co-signed by House Foreign Affairs chair Michael McCaul, requested a White House intelligence briefing on Microsoft’s investment in G42 before the deal could progress to its second phase, which would involve transferring export-restricted semiconductor chips from Nvidia and sophisticated AI model weights. The Biden administration has taken a positive view of the G42-Microsoft deal, stating that G42’s severance from China’s Huawei has been a major positive factor for the deal. However, last year, the administration also imposed sweeping curbs on AI chip exports, requiring licenses for shipments under a more restrictive policy than the previous Trump administration. Additionally, the policy of restricting exports to China requires licenses for exports to the UAE and some other Middle Eastern countries. It is noted that a regional visit by a congressional delegation took place, during which they met with Saudi officials who expressed a ******* to alleviate US companies’ concerns about the activities of the ******** government in Saudi Arabia. Their goal was to obtain permission to import advanced ********* chips. The level of interaction between US and other countries’ authorities illustrates the link between technological innovation, international political relationships, and national security issues. See also: UAE unveils new AI model to rival big tech giants Want to learn more about AI and big data from industry leaders? Check out AI & Big Data Expo taking place in Amsterdam, California, and London. The comprehensive event is co-located with other leading events including Intelligent Automation Conference, BlockX, Digital Transformation Week, and Cyber Security & Cloud Expo. Explore other upcoming enterprise technology events and webinars powered by TechForge here. The post UAE blocks US congressional meetings with G42 amid AI transfer concerns appeared first on AI News. View the full article
-
In a report published on Monday, it was disclosed that Apple sidestepped industry leader Nvidia in favour of chips designed by Google. Instead of employing Nvidia’s GPUs for its artificial intelligence software infrastructure, Apple will use Google chips as the cornerstone of AI-related features and tools set to launch soon. The fact that Apple chose to use Google’s cloud infrastructure is quite interesting because Nvidia has already captured this market, bringing Google, Amazon.com, and other cloud computing companies to 80% of the overall share. Therefore, it seems to be a smart strategic move by Apple to use an alternative source to minimise its hardware dependency. Apple has never revealed that it is avoiding Nvidia chips in any way. However, a statement in a research paper detailing the hardware and software infrastructure powering the company’s AI tools and features is raising eyebrows. This description conspicuously lacks any reference to Nvidia chips. When asked about this, Apple chose not to respond, leaving industry analysts to make educated guesses. According to the paper, Apple’s AI model training process leverages two distinct varieties of Google’s tensor processing units (TPUs), organised in expansive clusters of chips. When the tech giant Google prepared to build its new AI model, designed to operate on iPhones and other products offered by the company, it began deploying no fewer than 2,048 of its advanced TPUv5p chips. An even more significant part of developing the server-side for this model involved no fewer than 8,192 TPUv4 processors. Google’s decision to use TPUs contrasts with Nvidia’s focus on GPUs, which are now practically synonymous with machine learning development. Making the choice even more interesting, Google’s TPUs are not only different by design, but also by access. Unlike Nvidia’s products – which are available independently of other providers – Google only leases TPUs through the Google Cloud Platform, forcing customers to develop their software with the help of Google’s cloud to take advantage of them. Apple revealed this information just as it started rolling out its Apple Intelligence abilities to beta users. Currently, its developer beta program – which gives app creators an early preview of future software versions – incorporates a variety of AI-powered features. First and foremost, the use of its Writing Tools is now enhanced. Users may find an improved iteration of Siri, which now understands text as well as voice. AI alternatives can also be used for Mail and Smart Replies, thanks to email summarisation. As a bonus, beta testers can now transcribe their dictations with the assistance of AI. Based on real-world usage and feedback, Apple will be able to improve its AI features – such as ChatGPT integration – as well as expand the functionality of Siri to interact with third-party apps. Allowing users to familiarise themselves with the AI and provide feedback, this strategy will enable the corporation to make an optimal general release in the fall. Even though Reuters reported in June about Apple using TPU chips, the full scope of the use of Google hardware by the company would have remained unknown were it not for Monday’s detailed research. Furthermore, it is noteworthy that Apple’s engineers claimed to have the potential to develop even more significant and complex models with Google’s chips—suggesting that the current implementation is only the beginning of Apple’s AI projects. This news comes a week after Apple held its annual developer conference in June, where the firm revealed a range of new AI capabilities, including the incorporation of OpenAI’s ChatGPT technology into the Apple software ecosystem. There was widespread euphoria in the tech space, with some seeing it as proof positive of Apple’s commitment to the future, blending ambiance with the latest technological trends. Despite the significance of this news, Apple’s stock showed only a marginal reaction, ticking down 0.1% to $218.24 in regular trading on Monday. This muted response may indicate that investors are taking a wait-and-see approach to Apple’s AI strategy, particularly given the company’s historical tendency to enter new technological landscape deliberately, and with polished offerings. (Photo by Medhat Dawoud) See also: NVIDIA and Meta CEOs: Every business will ‘have an AI’ Want to learn more about AI and big data from industry leaders? Check out AI & Big Data Expo taking place in Amsterdam, California, and London. The comprehensive event is co-located with other leading events including Intelligent Automation Conference, BlockX, Digital Transformation Week, and Cyber Security & Cloud Expo. Explore other upcoming enterprise technology events and webinars powered by TechForge here. The post Apple opts for Google chips in AI infrastructure, sidestepping Nvidia appeared first on AI News. View the full article
-
In a fireside chat at SIGGRAPH 2024, NVIDIA founder and CEO Jensen Huang and Meta founder and CEO Mark Zuckerberg shared their insights on the potential of open source AI and virtual assistants. The conversation began with Zuckerberg announcing the launch of AI Studio, a new platform designed to democratise AI creation. This tool allows users to create, share, and discover AI characters, potentially opening up AI development to millions of creators and small businesses. Huang emphasised the ubiquity of AI in the future, stating, “Every single restaurant, every single website will probably, in the future, have these AIs …” Zuckerberg concurred, adding, “…just like every business has an email address and a website and a social media account, I think, in the future, every business is going to have an AI.” This vision aligns with NVIDIA’s recent developments showcased at SIGGRAPH. The company previewed “James,” an interactive digital human based on the NVIDIA ACE (Avatar Cloud Engine) reference design. James – a virtual assistant capable of providing contextually accurate responses – demonstrates the potential for businesses to create custom, hyperrealistic avatars for customer interactions. The discussion highlighted Meta’s significant contributions to AI development. Huang praised Meta’s work, saying, “You guys have done amazing AI work,” and cited advancements in computer vision, language models, and real-time translation. He also acknowledged the widespread use of PyTorch, an open-source machine learning framework developed by Meta. Both CEOs stressed the importance of open source in advancing AI. Meta has positioned itself as a leader in this field, implementing AI across its platforms and releasing open-source models like Llama 3.1. This latest model, with 405 billion parameters, required training on over 16,000 NVIDIA H100 GPUs, representing a substantial investment in resources. Zuckerberg shared his vision for more integrated AI models, saying, “I kind of dream of one day like you can almost imagine all of Facebook or Instagram being like a single AI model that has unified all these different content types and systems together.” He believes that collaboration is crucial for further advancements in AI. The conversation touched on the potential of AI to enhance human productivity. Huang described a future where AI could generate images in real-time as users type, allowing for fluid collaboration between humans and AI assistants. This concept is reflected in NVIDIA’s latest advancements to the NVIDIA Maxine AI platform, including Maxine 3D and Audio2Face-2D, which aim to create immersive telepresence experiences. Looking ahead, Zuckerberg expressed enthusiasm about combining AI with augmented reality eyewear, mentioning Meta’s collaboration with eyewear maker Luxottica. He envisions this technology transforming education, entertainment, and work. Huang discussed the evolution of AI interactions, moving beyond turn-based conversations to more complex, multi-option simulations. “Today’s AI is kind of turn-based. You say something, it says something back to you,” Huang explained. “In the future, AI could contemplate multiple options, or come up with a tree of options and simulate outcomes, making it much more powerful.” The importance of this evolution is evident in the adoption of NVIDIA’s technologies by companies across industries. HTC, Looking Glass, Reply, and UneeQ are among the latest firms using NVIDIA ACE and Maxine for applications ranging from customer service agents to telepresence experiences in entertainment, retail, and hospitality. As AI continues to evolve and integrate into various aspects of our lives, the insights shared by these industry leaders provide a glimpse into a future where AI assistants are as commonplace as websites and social media accounts. The developments showcased at SIGGRAPH 2024 by both NVIDIA and other companies demonstrate that this future is rapidly approaching, with digital humans becoming increasingly sophisticated and capable of natural, engaging interactions. See also: Amazon strives to outpace Nvidia with cheaper, faster AI chips Want to learn more about AI and big data from industry leaders? Check out AI & Big Data Expo taking place in Amsterdam, California, and London. The comprehensive event is co-located with other leading events including Intelligent Automation Conference, BlockX, Digital Transformation Week, and Cyber Security & Cloud Expo. Explore other upcoming enterprise technology events and webinars powered by TechForge here. The post NVIDIA and Meta CEOs: Every business will ‘have an AI’ appeared first on AI News. View the full article
-
In a move that underscores the growing influence of AI in the financial industry, JPMorgan Chase has unveiled a cutting-edge generative AI product. This new tool, LLM Suite, is being hailed as a game-changer and is capable of performing tasks traditionally assigned to research analysts. According to an internal memo obtained by the Financial Times, JPMorgan has granted employees in its asset and wealth management division access to this large language model platform. The memo, jointly signed by key executives Mary Erdoes, head of JPMorgan’s asset and wealth management business, Teresa Heitsenrether, the bank’s chief data and analytics officer, and Mike Urciuoli, the asset and wealth management unit’s chief information officer, describes LLM Suite as a “ChatGPT-like product” designed for “general purpose productivity.” The purpose of the platform is to change how employees work with their daily tasks, and it has various functions, including writing, helping to generate ideas, and document summarisation. The memo states, “Think of LLM Suite as a research analyst that can offer information, solutions, and advice on a topic.” Additionally, it is not an AI tool that performs functions by itself but an addition to the firm’s existing applications for handling financial information carefully—Connect Coach and SpectrumGPT. JPMorgan began the process of providing access to the LLM Suite to several of its departments earlier this year, representing a gradual implementation of the new system. At this point, an estimated 50,000 employees, or about 15% of the organisation’s workforce, can access the new platform. The number of research analysts who work for JPMorgan is not revealed, but the fact that the innovation affects so many employees from various departments raises the question of its relevance to traditional roles. It is worth mentioning that this is one of the most extensive implementations of large language models on Wall Street. For example, Morgan Stanley has started developing AI products built by OpenAI to improve their wealth management businesses. However, JPMorgan distinguishes itself from other financial organisations by developing its own AI tool, LLM Suite. A powerful reason to create the LLM Suite internally is the high level of regulation in the financial services domain. According to the strict regulations typical for any financial organisation, JPMorgan workers are not allowed to use any AI chatbots developed by other companies for consumers. These include Anthropic’s Claude, OpenAI’s GPT, and Google’s Gemini. Another reason is to ensure that customer information ******** on the bank’s servers safe and sound. As a company actively involved in the use of AI for enhancing services to its clients, JPMorgan Bank CEO Jamie Dimon made a statement to investors in May: “AI is going to change every job. It may eliminate some jobs. Some of it may create additional jobs.” He went further to state, “But you can’t envision one app, one database, or one job where it’s not going to help, aid, or abet.” Such a statement reflects the bank’s forward-looking attitude towards AI. Already, AI technologies contribute a significant amount of money to the bank. According to Daniel Pinto, the president of the bank, the value of AI technology currently in use is $1 to $1.5 billion. This fact shows that the effect of AI adoption is significant for the bank, which means it is also beneficial for society. Despite the fact that the release of LLM Suite is a breakthrough in AI implementation in the financial sphere, it is still possible to note that the technology has some drawbacks. Specifically, like any other AI model, LLM Suite may not work with sufficient accuracy, may “hallucinate,” or provide false data as correct information. Nevertheless, the memo does not discuss these issues or whether they exist. (Photo by IKECHUKWU JULIUS UGWU) See also: Meta advances open source AI with ‘frontier-level’ Llama 3.1 Want to learn more about AI and big data from industry leaders? Check out AI & Big Data Expo taking place in Amsterdam, California, and London. The comprehensive event is co-located with other leading events including Intelligent Automation Conference, BlockX, Digital Transformation Week, and Cyber Security & Cloud Expo. Explore other upcoming enterprise technology events and webinars powered by TechForge here. The post JPMorgan introduces in-house AI chatbot for research analysis appeared first on AI News. View the full article
-
Galileo, a leading developer of generative AI for enterprise applications, has released its latest Hallucination Index. The evaluation framework – which focuses on Retrieval Augmented Generation (RAG) – assessed 22 prominent Gen AI LLMs from major players including OpenAI, Anthropic, Google, and Meta. This year’s index expanded significantly, adding 11 new models to reflect the rapid growth in both open- and closed-source LLMs over the past eight months. Vikram Chatterji, CEO and Co-founder of Galileo, said: “In today’s rapidly evolving AI landscape, developers and enterprises face a critical challenge: how to harness the power of generative AI while balancing cost, accuracy, and reliability. Current benchmarks are often based on academic use-cases, rather than real-world applications.” The index employed Galileo’s proprietary evaluation metric, context adherence, to check for output inaccuracies across various input lengths, ranging from 1,000 to 100,000 tokens. This approach aims to help enterprises make informed decisions about balancing price and performance in their AI implementations. Key findings from the index include: Anthropic’s Claude 3.5 Sonnet emerged as the best overall performing model, consistently scoring near-perfect across short, medium, and long context scenarios. Google’s Gemini 1.5 Flash ranked as the best performing model in terms of cost-effectiveness, delivering strong performance across all tasks. Alibaba’s Qwen2-72B-Instruct stood out as the top open-source model, particularly excelling in short and medium context scenarios. The index also highlighted several trends in the LLM landscape: Open-source models are rapidly closing the gap with their closed-source counterparts, offering improved hallucination performance at lower costs. Current RAG LLMs demonstrate significant improvements in handling extended context lengths without sacrificing quality or accuracy. Smaller models sometimes outperform larger ones, suggesting that efficient design can be more crucial than scale. The emergence of strong performers from outside the US, such as Mistral’s Mistral-large and Alibaba’s qwen2-72b-instruct, indicates a growing global competition in LLM development. While closed-source models like Claude 3.5 Sonnet and Gemini 1.5 Flash maintain their lead due to proprietary training data, the index reveals that the landscape is evolving rapidly. Google’s performance was particularly noteworthy, with its open-source Gemma-7b model performing poorly while its closed-source Gemini 1.5 Flash consistently ranked near the top. As the AI industry continues to grapple with hallucinations as a major hurdle to production-ready Gen AI products, Galileo’s Hallucination Index provides valuable insights for enterprises looking to adopt the right model for their specific needs and budget constraints. See also: Senators probe OpenAI on safety and employment practices Want to learn more about AI and big data from industry leaders? Check out AI & Big Data Expo taking place in Amsterdam, California, and London. The comprehensive event is co-located with other leading events including Intelligent Automation Conference, BlockX, Digital Transformation Week, and Cyber Security & Cloud Expo. Explore other upcoming enterprise technology events and webinars powered by TechForge here. The post Anthropic to Google: Who’s winning against AI hallucinations? appeared first on AI News. View the full article
-
Amazon’s chip lab is churning out a constant stream of innovation in Austin, Texas. A new server design was put through its paces by a group of devoted engineers on July 26th. During a visit to the facility in Austin, Amazon executive Rami Sinno shed light on the server’s use of Amazon’s AI chips. This development is a bold step toward competing with Nvidia, the current leader in the field. The main reason Amazon is developing its own processor is this: it doesn’t want to rely on Nvidia and buy the company’s chips. The expensive Nvidia chips power a big part of the AI cloud business at Amazon Web Services. This business is the most significant growth engine of the company. Thus, the so-called “Nvidia tax” was pushing the company to look for a cheaper option. Amazon’s chip development program has a dual purpose. Firstly, the project is meant to provide customers with more affordable opportunities for complex calculations and large data volume processing. Secondly, the initiative was developed to preserve Amazon’s competitiveness in the volatile cloud computing and AI industry. This move was also supported by the directions of tech giants such as Microsoft and Alphabet, which are developing custom-made chips to maintain their leadership in the market. Rami Sinno, director of engineering for Amazon’s Annapurna Labs, a key element of the AWS ecosystem, emphasised that customer demand for more economical solutions to Nvidia’s products is growing. The acquisition of Annapurna Labs in 2015 was a savvy move by Amazon as it enabled the company to lay the groundwork to begin developing popular chips. Although Amazon’s chips for AI are in their early days, the company has been making and refining chips for other mainstream applications for nearly a decade, most notably its general-purpose chip, Graviton, which is now in its fourth generation. Amazon has announced that its Trainium and Inferentia chips, the company’s latest and strongest, are still in their early days and are specially designed processors. The impact is potentially huge because the impressive performance underscores the reports by David Brown, vice president of compute and networking at AWS. In this light, it should be acknowledged that Amazon’s in-house chips could deliver up to a 40-50% price-performance ratio improvement compared to Nvidia-based solutions. In turn, this potential improvement could mean considerable savings for AWS clientele deploying their AI workloads. AWS’ significance to Amazon’s overall business cannot be underestimated. In the first quarter of this year, AWS made up a little under a fifth of Amazon’s total revenue, as its sales soared by 17 per cent year over year to reach $25 billion. At the moment, AWS holds about a third of the global cloud computing market, and Microsoft’s Azure covers about a quarter, or 25%. Amazon’s commitment to its custom chip strategy was demonstrated during the recent Prime Day, a two-day sales event at Amazon.com. To handle the highly elevated level of shopping as well as streaming video, music, and other content, Amazon deployed an impressive 250,000 Graviton chips and 80,000 of its custom AI chips across its platforms. Adobe Analytics announced record Prime Day results of $14.2 billion in sales. It seems that as Amazon intensifies its work on the development of AI chips, the industry leader, Nvidia, is not going to remain at the same level. Nvidia’s CEO, Jensen Huang, has presented Nvidia’s latest Blackwell chips, which are scheduled for release later in the year. Their performance has increased significantly, and Huang promised that the new chips are twice as powerful for AI model training and five times faster for inference. Nvidia’s dominant position in the AI chip market is underscored by its impressive client list, which includes tech giants like Amazon, Google, Microsoft, OpenAI, and Meta. The company’s focus on AI has propelled its market value to a staggering $2 trillion, making it the third most valuable company globally, behind only Microsoft and Apple. As the AI chip race intensifies, Nvidia is also diversifying its offerings. The company has introduced new software tools to facilitate AI integration across various industries and is developing specialised chips for emerging applications such as in-car chatbots and humanoid robots. (Image by Gerd Altmann) See also: Nvidia: World’s most valuable company under French antitrust ***** Want to learn more about AI and big data from industry leaders? Check out AI & Big Data Expo taking place in Amsterdam, California, and London. The comprehensive event is co-located with other leading events including Intelligent Automation Conference, BlockX, Digital Transformation Week, and Cyber Security & Cloud Expo. Explore other upcoming enterprise technology events and webinars powered by TechForge here. The post Amazon strives to outpace Nvidia with cheaper, faster AI chips appeared first on AI News. View the full article
-
Tech giants like Microsoft, Alphabet, and Meta are riding high on a wave of revenue from AI-driven cloud services, yet simultaneously drowning in the substantial costs of pushing AI’s boundaries. Recent financial reports paint a picture of a double-edged sword: on one side, impressive gains; on the other, staggering expenses. This dichotomy has led Bloomberg to aptly dub AI development a “huge money pit,” highlighting the complex economic reality behind today’s AI revolution. At the heart of this financial problem ***** a relentless push for *******, more sophisticated AI models. The quest for artificial general intelligence (AGI) has led companies to develop increasingly complex systems, exemplified by large language models like GPT-4. These models require vast computational power, driving up hardware costs to unprecedented levels. To top it off, the demand for specialised AI chips, mainly graphics processing units (GPUs), has skyrocketed. Nvidia, the leading manufacturer in this space, has seen its market value soar as tech companies scramble to secure these essential components. Its H100 graphics chip, the gold standard for training AI models, has sold for an estimated $30,000 — with some resellers offering them for multiple times that amount. The global chip shortage has only exacerbated this issue, with some firms waiting months to acquire the necessary hardware. Meta Chief Executive Officer Zuckerberg previously said that his company planned to acquire 350,000 H100 chips by the end of this year to support its AI research efforts. Even if he gets a bulk-buying discount, that quickly adds to billions of dollars. On the other hand, the push for more advanced AI has also sparked an arms race in chip design. Companies like Google and Amazon invest heavily in developing their AI-specific processors, aiming to gain a competitive edge and reduce reliance on third-party suppliers. This trend towards custom silicon adds another layer of complexity and cost to the AI development process. But the hardware challenge extends beyond just procuring chips. The scale of modern AI models necessitates massive data centres, which come with their technological hurdles. These facilities must be designed to handle extreme computational loads while managing heat dissipation and energy consumption efficiently. As models grow larger, so do the power requirements, significantly increasing operational costs and environmental impact. In a podcast interview in early April, Dario Amodei, the chief executive officer of OpenAI-rival Anthropic, said the current crop of AI models on the market cost around $100 million to train. “The models that are in training now and that will come out at various times later this year or early next year are closer in cost to $1 billion,” he said. “And then I think in 2025 and 2026, we’ll get more towards $5 or $10 billion.” Then, there is data, the lifeblood of AI systems, presenting its own technological challenges. The need for vast, high-quality datasets has led companies to invest heavily in data collection, cleaning, and annotation technologies. Some firms are developing sophisticated synthetic data generation tools to supplement real-world data, further driving up research and development costs. The rapid pace of AI innovation also means that infrastructure and tools quickly become obsolete. Companies must continuously upgrade their systems and retrain their models to stay competitive, creating a constant cycle of investment and obsolescence. “On April 25, Microsoft said it spent $14 billion on capital expenditures in the most recent quarter and expects those costs to “increase materially,” driven partly by AI infrastructure investments. That was a 79% increase from the year-earlier quarter. Alphabet said it spent $12 billion during the quarter, a 91% increase from a year earlier, and expects the rest of the year to be “at or above” that level as it focuses on AI opportunities,” the article by Bloomberg reads. Bloomberg also noted that Meta, meanwhile, raised its estimates for investments for the year and now believes capital expenditures will be $35 billion to $40 billion, which would be a 42% increase at the high end of the range. “It cited aggressive investment in AI research and product development,” Bloomberg wrote. Interestingly, Bloomberg’s article also points out that despite these enormous costs, tech giants are proving that AI can be a real revenue driver. Microsoft and Alphabet reported significant growth in their cloud businesses, mainly attributed to increased demand for AI services. This suggests that while the initial investment in AI technology is staggering, the potential returns are compelling enough to justify the expense. However, the high costs of AI development raise concerns about market concentration. As noted in the article, the expenses associated with cutting-edge AI research may limit innovation to a handful of well-funded companies, potentially stifling competition and diversity in the field. Looking ahead, the industry is focusing on developing more efficient AI technologies to address these cost challenges. Research into techniques like few-shot learning, transfer learning, and more energy-efficient model architectures aims to reduce the computational resources required for AI development and deployment. Moreover, the push towards edge AI – running AI models on local devices rather than in the cloud – could help distribute computational loads and reduce the strain on centralised data centres. This shift, however, requires its own set of technological innovations in chip design and software optimisation. Overall, it is clear that the future of AI will be shaped not just by breakthroughs in algorithms and model design but also by our ability to overcome the immense technological and financial hurdles that come with scaling AI systems. Companies that can navigate these challenges effectively will likely emerge as the leaders in the next phase of the AI revolution. (Image by Igor Omilaev) Want to learn more about AI and big data from industry leaders? Check out AI & Big Data Expo taking place in Amsterdam, California, and London. The comprehensive event is co-located with other leading events including Intelligent Automation Conference, BlockX, Digital Transformation Week, and Cyber Security & Cloud Expo. Explore other upcoming enterprise technology events and webinars powered by TechForge here. The post The exponential expenses of AI development appeared first on AI News. View the full article
-
Mistral AI’s latest model, Mistral Large 2 (ML2), allegedly competes with large models from industry leaders like OpenAI, Meta, and Anthropic, despite being a fraction of their sizes. The timing of this release is noteworthy, arriving the same week as Meta’s launch of its behemoth 405-billion-parameter Llama 3.1 model. Both ML2 and Llama 3 boast impressive capabilities, including a 128,000 token context window for enhanced “memory” and support for multiple languages. Mistral AI has long differentiated itself through its focus on language diversity, and ML2 continues this tradition. The model supports “dozens” of languages and more than 80 coding languages, making it a versatile tool for developers and businesses worldwide. According to Mistral’s benchmarks, ML2 performs competitively against top-tier models like OpenAI’s GPT-4o, Anthropic’s Claude 3.5 Sonnet, and Meta’s Llama 3.1 405B across various language, coding, and mathematics tests. In the widely-recognised Massive Multitask Language Understanding (MMLU) benchmark, ML2 achieved a score of 84 percent. While slightly behind its competitors (GPT-4o at 88.7%, Claude 3.5 Sonnet at 88.3%, and Llama 3.1 405B at 88.6%), it’s worth noting that human domain experts are estimated to score around 89.8% on this test. Efficiency: A key advantage What sets ML2 apart is its ability to achieve high performance with significantly fewer resources than its rivals. At 123 billion parameters, ML2 is less than a third the size of Meta’s largest model and approximately one-fourteenth the size of GPT-4. This efficiency has major implications for deployment and commercial applications. At full 16-bit precision, ML2 requires about 246GB of memory. While this is still too large for a single GPU, it can be easily deployed on a server with four to eight GPUs without resorting to quantisation – a feat not necessarily achievable with larger models like GPT-4 or Llama 3.1 405B. Mistral emphasises that ML2’s smaller footprint translates to higher throughput, as LLM performance is largely dictated by memory bandwidth. In practical terms, this means ML2 can generate responses faster than larger models on the same hardware. Addressing key challenges Mistral has prioritised combating hallucinations – a common issue where AI models generate convincing but inaccurate information. The company claims ML2 has been fine-tuned to be more “cautious and discerning” in its responses and better at recognising when it lacks sufficient information to answer a query. Additionally, ML2 is designed to excel at following complex instructions, especially in longer conversations. This improvement in prompt-following capabilities could make the model more versatile and user-friendly across various applications. In a nod to practical business concerns, Mistral has optimised ML2 to generate concise responses where appropriate. While verbose outputs can lead to higher benchmark scores, they often result in increased compute time and operational costs – a consideration that could make ML2 more attractive for commercial use. Licensing and availability While ML2 is freely available on popular repositories like Hugging Face, its licensing terms are more restrictive than some of Mistral’s previous offerings. Unlike the open-source Apache 2 license used for the Mistral-NeMo-12B model, ML2 is released under the Mistral Research License. This allows for non-commercial and research use but requires a separate commercial license for business applications. As the AI race heats up, Mistral’s ML2 represents a significant step forward in balancing power, efficiency, and practicality. Whether it can truly challenge the dominance of tech giants ******** to be seen, but its release is certainly an exciting addition to the field of large language models. (Photo by Sean Robertson) See also: Senators probe OpenAI on safety and employment practices Want to learn more about AI and big data from industry leaders? Check out AI & Big Data Expo taking place in Amsterdam, California, and London. The comprehensive event is co-located with other leading events including Intelligent Automation Conference, BlockX, Digital Transformation Week, and Cyber Security & Cloud Expo. Explore other upcoming enterprise technology events and webinars powered by TechForge here. The post Mistral Large 2: The David to Big Tech’s Goliath(s) appeared first on AI News. View the full article
-
Meta has unveiled Llama 3.1, marking a significant milestone in the company’s commitment to open source AI. This release, which Meta CEO Mark Zuckerberg calls “the first frontier-level open source AI model,” aims to challenge the dominance of closed AI systems and democratise access to advanced AI technology. The Llama 3.1 release includes three models: 405B, 70B, and 8B. Zuckerberg asserts that the 405B model competes with the most advanced closed models while offering better cost-efficiency. The CEO views this release as a turning point, predicting that most developers will shift towards primarily using open source AI models. He invites the tech community to join Meta in “this journey to bring the benefits of AI to everyone in the world.” The Llama 3.1 models are now accessible at llama.meta.com. (Photo by Dima Solomin) See also: Meta joins Apple in withholding AI models from EU users [Hidden Content] Want to learn more about AI and big data from industry leaders? Check out AI & Big Data Expo taking place in Amsterdam, California, and London. The comprehensive event is co-located with other leading events including Intelligent Automation Conference, BlockX, Digital Transformation Week, and Cyber Security & Cloud Expo. Explore other upcoming enterprise technology events and webinars powered by TechForge here. The post Meta advances open source AI with ‘frontier-level’ Llama 3.1 appeared first on AI News. View the full article
-
If your business relies on web applications, you’re probably familiar with traditional network firewalls. And for good reason – they play an invaluable role filtering external threats looking to ******* your overall infrastructure. But as more and more of your essential operations shift online to intricate web apps and APIs, gaps have opened up that basic firewalls simply can’t see into. The new AI-powered threats of today demand a new approach to security. Without visibility into your custom application logic and data flows, major vulnerabilities can be exploited, allowing sensitive information theft, financial ******, and even operational disruption. While you still need perimeter firewall defenses, exclusively relying on them to safeguard increasingly powerful web properties leaves you playing a risky game of chance (with very real consequences). By adding specialised web application firewalls (WAFs) designed to analyse requests in the full context of your app environments – and enhanced by AI for even greater accuracy – you can lock things down and confidently build out advanced digital capabilities. With a layered defense-in-depth approach combining network and application-level protections, you can securely deliver the types of seamless, personalised digital experiences that form the foundation of lasting customer relationships and operational excellence in 2024. Gaps in traditional firewall defences The chances are you already have traditional firewall protection guarding your overall network (if you run any online services). These firewalls filter incoming traffic based on a set of predefined rules focused primarily around protocol, port number, IP address ranges, and basic connection state. For example, common firewall rules restrict outside access to private intranet resources, block unwanted traffic types like online gaming protocols, detect large-scale network scans, and mitigate distributed denial of service (DDoS) attacks. This perimeter protection works well for classic network-focused cyberthreats. But a traditional firewall lacks context about the application logic, user workflows, and data structures unique to custom web apps and APIs. It simply scans network packets as they arrive and attempts to allow or block them accordingly. This leaves it vulnerable to the evolving tactics of AI-powered attackers. Without insight into application internals, major vulnerabilities can sneak right past traditional firewall defences: SQL injection attacks: Inserting malicious code allowing remote access, data destruction, or information theft Broken authentication: Enabling unauthorised system access with stolen credentials Sensitive data exposure: Through improper encryption, backups, or logging Cross-site scripting (XSS): Injecting JavaScript or HTML to spread malware, ******* sessions, scrape data, or deface sites Hackers can also target configuration issues, flawed business logic flows, identity management gaps, and unsafe object level access once inside applications themselves. AI-powered attacks can exploit these vulnerabilities with alarming speed and precision—and your firewall wouldn’t see it coming. These exploitable application flaws allow attackers to steal sensitive business data and personal information, mine cryptocurrency illicitly on servers, hold systems ransom, take over client accounts, and both deny legitimate access and ******** backend resources. AI has only amplified these risks. Still, traditional firewalls remain extremely important as the first line of network perimeter defence. But for companies conducting operations online through modern web apps, additional safeguards tuned to application threats – and bolstered by AI’s threat detection capabilities – are essential. Why WAFs provide critical protection Web application firewalls address the application layer vulnerabilities and holes in logic that basic network firewalls miss. WAFs are designed specifically to protect web apps, APIs, microservices, and rich internet applications. AI further enhances their ability to identify and respond to these threats. A WAF will deeply inspect all traffic flowing to web properties using targeted rulesets and negative security models defining suspicious behaviour. From there, they analyse requests for indicators of common exploits and attacks seeking to ****** application behaviour and functionality. AI-powered analysis can detect subtle patterns that might otherwise go unnoticed. These might include: Extreme traffic spikes indicating possible DDoS events Suspicious geolocations of an IP addresses Repeated input submissions just below lockout thresholds Unusual HTTP headers, user agents, or protocols Known malicious payloads in POST requests Attempts to traverse directory structures in unpredictable ways Special characters and patterns indicating SQL injection or cross-site scripting Advanced WAFs combine this real-time threat detection with global threat intelligence to identify emerging exploits and bad actors as soon as new ******* patterns appear. AI and machine learning algorithms even allow some solutions to derive additional behavioral rules by examining your specific application traffic patterns over time. AI’s adaptability is crucial in this constantly shifting landscape. As traffic passes through, the WAF blocks dangerous requests while allowing legitimate users through with minimal latency impact. This protects the application itself, shielding both data and functionality from compromise. AI-powered WAFs can do this with remarkable speed and accuracy, keeping pace with the ever-changing threat landscape. Most WAF products also include capabilities like virtual patching, behavioral anomaly detection, automatic policy tuning, third-party integration, and positive security models for detecting verified use cases. Breaking down the key features of traditional firewalls vs WAFs FeatureTraditional FirewallWeb Application Firewall (WAF)Layer of operationNetwork (Layer 3/4)Application (Layer 7)Traffic analysisPackets, ports, IP addressesHTTP/HTTPS requests, content, parameters, headers******* protectionNetwork-level attacksWeb application-specific attacks (SQLi, XSS, CSRF, etc.)CustomisationLimitedExtensiveAdditional capabilitiesMay offer basic intrusion preventionOften include **** mitigation, DDoS protection, API securityAI integrationLimited or non-existentConsiderably more prevalent. Used to enhance threat detection and and incident response Creating an application security ladder Web applications underpin many essential business capabilities – internal operations management, customer experience, partner integration – the list goes on. As reliance on these application ecosystems grows, so does business risk exposure through underlying vulnerabilities. Strengthening application security closes major blindspots while allowing companies to pursue advanced digital transformation supporting key goals around: Improving self-service and convenience through customer portal expansion Accelerating development velocity using CI/CD pipelines and microservices Enabling real-time data exchanges through IoT integrations and open API ecosystems Increasing revenue with personalised interfaces and recommendation engines Combining network-layer perimeter defences from traditional firewalls with reinforced protections from specialised WAFs creates a security ladder effect. The traditional firewall filters allowed traffic at the network level based on IPs, protocols, and volume heuristics. This protects against basic attacks like worms, reconnaissance scans, and DDoS events. Then the WAF takes over at the application layer, scrutinising the full context of requests to identify attempts to exploit app logic and functionality itself using injection attacks, stolen credentials, unusual workflows, or other sneaky techniques security teams encounter daily. Together, this layered defence-in-depth approach secures both the overall network and the intricate web apps conducting an ever-larger percentage of essential business. Companies can then direct more development resources towards advancing capabilities rather than just patching vulnerabilities. Final word The costs of security incidents grow more severe year over year. And as companies rely increasingly on web apps to manage operations, serve customers, and drive revenue, application vulnerabilities present a serious (and immediate) business risk. Protecting systems with advanced application-aware defenses – powered by AI – means that your security supports rather than gets in the way of your key strategic initiatives With scalable and secure defenses guarding your web properties, you can confidently build capabilities supporting goals around better customer experience, smoother operations, increased sales growth, and expanded partner channels. In other words, you can focus on pushing your business forward with the peace of mind knowing that you’ve done your part in securing your perimeter and web apps in our ever AI-driven world. The post AI-powered WAFs vs traditional firewalls: Protecting your web applications appeared first on AI News. View the full article