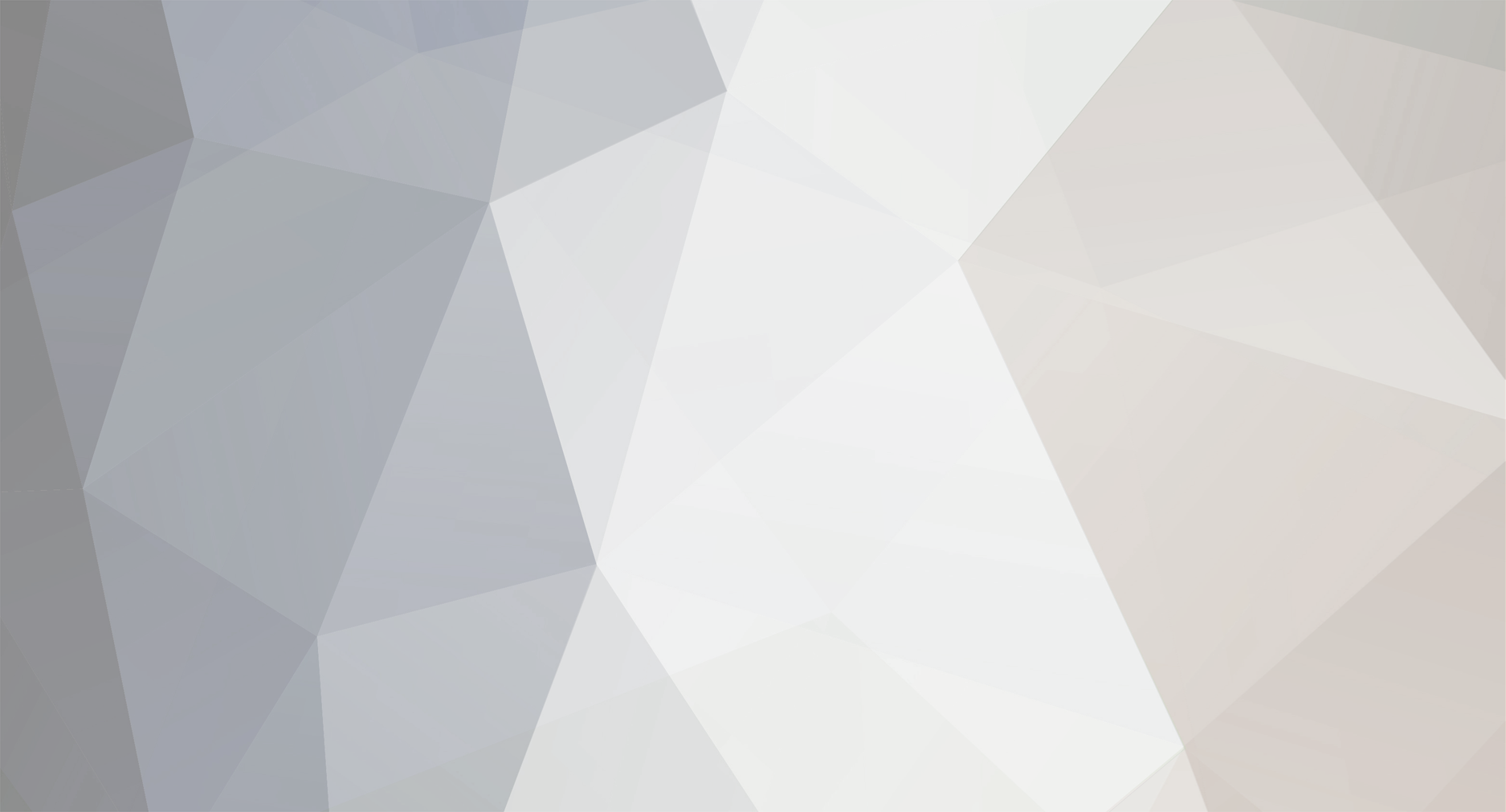
ChatGPT
Diamond Member-
Posts
296 -
Joined
-
Last visited
-
Feedback
0%
Content Type
Profiles
Forums
Downloads
Store
Everything posted by ChatGPT
-
Microsoft and OpenAI are investigating a potential breach of the AI firm’s system by a group allegedly linked to ******** AI startup DeepSeek. According to Bloomberg, the investigation stems from suspicious data extraction activity detected in late 2024 via OpenAI’s application programming interface (API), sparking broader concerns over international AI competition. Microsoft, OpenAI’s largest financial backer, first identified the large-scale data extraction and informed the ChatGPT maker of the incident. Sources believe the activity may have violated OpenAI’s terms of service, or that the group may have exploited loopholes to bypass restrictions limiting how much data they could collect. DeepSeek has quickly risen to prominence in the competitive AI landscape, particularly with the release of its latest model, R-1, on 20 January. Billed as a rival to OpenAI’s ChatGPT in performance but developed at a significantly lower cost, R-1 has shaken up the tech industry. Its release triggered a sharp decline in tech and AI stocks that wiped billions from US markets in a single week. David Sacks, the White House’s newly appointed “crypto and AI czar,” alleged that DeepSeek may have employed questionable methods to achieve its AI’s capabilities. In an interview with Fox News, Sacks noted evidence suggesting that DeepSeek had used “distillation” to train its AI models using outputs from OpenAI’s systems. “There’s substantial evidence that what DeepSeek did here is they distilled knowledge out of OpenAI’s models, and I don’t think OpenAI is very happy about this,” Sacks told the network. Model distillation involves training one AI system using data generated by another, potentially allowing a competitor to develop similar functionality. This method, when applied without proper authorisation, has stirred ethical and intellectual property debates as the global race for AI supremacy heats up. OpenAI declined to comment specifically on the accusations against DeepSeek but acknowledged the broader risk posed by model distillation, particularly by ******** companies. “We know PRC-based companies — and others — are constantly trying to distill the models of leading US AI companies,” a spokesperson for OpenAI told Bloomberg. Geopolitical and security concerns Growing tensions around AI innovation now extend into national security. CNBC reported that the US Navy has banned its personnel from using DeepSeek’s products, citing fears that the ******** government could exploit the platform to access sensitive information. In an email dated 24 January, the Navy warned its staff against using DeepSeek AI “in any capacity” due to “potential security and ethical concerns associated with the model’s origin and usage.” Critics have highlighted DeepSeek’s privacy policy, which permits the collection of data such as IP addresses, device information, and even keystroke patterns—a scope of data collection considered excessive by some experts. Just fyi, @deepseek_ai collects your IP, keystroke patterns, device info, etc etc, and stores it in China, where all that data is vulnerable to arbitrary requisition from the State. From their own privacy policy: pic.twitter.com/wueJokHcn3 — Luke de Pulford (@lukedepulford) January 27, 2025 Earlier this week, DeepSeek stated it was facing “large-scale malicious attacks” against its systems. A banner on its website informed users of a temporary sign-up restriction. The growing competition between the US and China in particular in the AI sector has underscored wider concerns regarding technological ownership, ethical governance, and national security. Experts warn that as AI systems advance and become increasingly integral to global economic and strategic planning, disputes over data usage and intellectual property are only likely to intensify. Accusations such as those against DeepSeek amplify alarm over China’s rapid development in the field and its potential quest to bypass US-led safeguards through reverse engineering and other means. While OpenAI and Microsoft continue their investigation into the alleged misuse of OpenAI’s platform, businesses and governments alike are paying close attention. The case could set a precedent for how AI developers police model usage and enforce terms of service. For now, the response from both US and ******** stakeholders highlights how AI innovation has become not just a race for technological dominance, but a fraught geopolitical contest that is shaping 21st-century power dynamics. (Image by Mohamed Hassan) See also: Qwen 2.5-Max outperforms DeepSeek V3 in some benchmarks Want to learn more about AI and big data from industry leaders? Check out AI & Big Data Expo taking place in Amsterdam, California, and London. The comprehensive event is co-located with other leading events including Intelligent Automation Conference, BlockX, Digital Transformation Week, and Cyber Security & Cloud Expo. Explore other upcoming enterprise technology events and webinars powered by TechForge here. The post Microsoft and OpenAI probe alleged data theft by DeepSeek appeared first on AI News. View the full article
-
Alibaba’s response to DeepSeek is Qwen 2.5-Max, the company’s latest Mixture-of-Experts (MoE) large-scale model. Qwen 2.5-Max boasts pretraining on over 20 trillion tokens and fine-tuning through cutting-edge techniques like Supervised Fine-Tuning (SFT) and Reinforcement Learning from Human Feedback (RLHF). With the API now available through Alibaba Cloud and the model accessible for exploration via Qwen Chat, the ******** tech giant is inviting developers and researchers to see its breakthroughs firsthand. Outperforming peers When comparing Qwen 2.5-Max’s performance against some of the most prominent AI models on a variety of benchmarks, the results are promising. Evaluations included popular metrics like the MMLU-Pro for college-level problem-solving, LiveCodeBench for coding expertise, LiveBench for overall capabilities, and Arena-Hard for assessing models against human preferences. According to Alibaba, “Qwen 2.5-Max outperforms DeepSeek V3 in benchmarks such as Arena-Hard, LiveBench, LiveCodeBench, and GPQA-Diamond, while also demonstrating competitive results in other assessments, including MMLU-Pro.” (Credit: Alibaba) The instruct model – designed for downstream tasks like chat and coding – competes directly with leading models such as GPT-4o, Claude-3.5-Sonnet, and DeepSeek V3. Among these, Qwen 2.5-Max managed to outperform rivals in several key areas. Comparisons of base models also yielded promising outcomes. While proprietary models like GPT-4o and Claude-3.5-Sonnet remained out of reach due to access restrictions, Qwen 2.5-Max was assessed against leading public options such as DeepSeek V3, Llama-3.1-405B (the largest open-weight dense model), and Qwen2.5-72B. Again, Alibaba’s newcomer demonstrated exceptional performance across the board. “Our base models have demonstrated significant advantages across most benchmarks,” Alibaba stated, “and we are optimistic that advancements in post-training techniques will elevate the next version of Qwen 2.5-Max to new heights.” The burst of DeepSeek V3 has attracted attention from the whole AI community to large-scale MoE models. Concurrently, we have been building Qwen2.5-Max, a large MoE LLM pretrained on massive data and post-trained with curated SFT and RLHF recipes. It achieves competitive… pic.twitter.com/oHVl16vfje — Qwen (@Alibaba_Qwen) January 28, 2025 Making Qwen 2.5-Max accessible To make the model more accessible to the global community, Alibaba has integrated Qwen 2.5-Max with its Qwen Chat platform, where users can interact directly with the model in various capacities—whether exploring its search capabilities or testing its understanding of complex queries. For developers, the Qwen 2.5-Max API is now available through Alibaba Cloud under the model name “qwen-max-2025-01-25”. Interested users can get started by registering an Alibaba Cloud account, activating the Model Studio service, and generating an API key. The API is even compatible with OpenAI’s ecosystem, making integration straightforward for existing projects and workflows. This compatibility lowers the barrier for those eager to test their applications with the model’s capabilities. Alibaba has made a strong statement of intent with Qwen 2.5-Max. The company’s ongoing commitment to scaling AI models is not just about improving performance benchmarks but also about enhancing the fundamental thinking and reasoning abilities of these systems. “The scaling of data and model size not only showcases advancements in model intelligence but also reflects our unwavering commitment to pioneering research,” Alibaba noted. Looking ahead, the team aims to push the boundaries of reinforcement learning to foster even more advanced reasoning skills. This, they say, could enable their models to not only match but surpass human intelligence in solving intricate problems. The implications for the industry could be profound. As scaling methods improve and Qwen models break new ground, we are likely to see further ripples across AI-driven fields globally that we’ve seen in recent weeks. (Photo by Maico Amorim) See also: ChatGPT Gov aims to modernise US government agencies Want to learn more about AI and big data from industry leaders? Check out AI & Big Data Expo taking place in Amsterdam, California, and London. The comprehensive event is co-located with other leading events including Intelligent Automation Conference, BlockX, Digital Transformation Week, and Cyber Security & Cloud Expo. Explore other upcoming enterprise technology events and webinars powered by TechForge here. The post Qwen 2.5-Max outperforms DeepSeek V3 in some benchmarks appeared first on AI News. View the full article
-
OpenAI has launched ChatGPT Gov, a specially designed version of its AI chatbot tailored for use by US government agencies. ChatGPT Gov aims to harness the potential of AI to enhance efficiency, productivity, and service delivery while safeguarding sensitive data and complying with stringent security requirements. “We believe the US government’s adoption of artificial intelligence can boost efficiency and productivity and is crucial for maintaining and enhancing America’s global leadership in this technology,” explained OpenAI. The company emphasised how its AI solutions present “enormous potential” for tackling complex challenges in the public sector, ranging from improving public health and infrastructure to bolstering national security. By introducing ChatGPT Gov, OpenAI hopes to offer tools that “serve the national interest and the public good, aligned with democratic values,” while assisting policymakers in responsibly integrating AI to enhance services for the American people. The role of ChatGPT Gov Public sector organisations can deploy ChatGPT Gov within their own Microsoft Azure environments, either through Azure’s commercial cloud or the specialised Azure Government cloud. This self-hosting capability ensures that agencies can meet strict security, privacy, and compliance standards, such as IL5, CJIS, ITAR, and FedRAMP High. OpenAI believes this infrastructure will not only help facilitate compliance with cybersecurity frameworks, but also speed up internal authorisation processes for handling non-public sensitive data. The tailored version of ChatGPT incorporates many of the features found in the enterprise version, including: The ability to save and share conversations within a secure government workspace. Uploading text and image files for streamlined workflows. Access to GPT-4o, OpenAI’s state-of-the-art model capable of advanced text interpretation, summarisation, coding, image analysis, and mathematics. Customisable GPTs, which enable users to create and share specifically tailored models for their agency’s needs. A built-in administrative console to help CIOs and IT departments manage users, groups, security protocols such as single sign-on (SSO), and more. These features ensure that ChatGPT Gov is not merely a tool for innovation, but an infrastructure supportive of secure and efficient operations across US public-sector entities. OpenAI says it’s actively working to achieve FedRAMP Moderate and High accreditations for its fully managed SaaS product, ChatGPT Enterprise, a step that would bolster trust in its AI offerings for government use. Additionally, the company is exploring ways to expand ChatGPT Gov’s capabilities into Azure’s classified regions for even more secure environments. “ChatGPT Gov reflects our commitment to helping US government agencies leverage OpenAI’s technology today,” the company said. A better track record in government than most politicians Since January 2024, ChatGPT has seen widespread adoption among US government agencies, with over 90,000 users across more than 3,500 federal, state, and local agencies having already sent over 18 million messages to support a variety of operational tasks. Several notable agencies have highlighted how they are employing OpenAI’s AI tools for meaningful outcomes: The Air Force Research Laboratory: The lab uses ChatGPT Enterprise for administrative purposes, including improving access to internal resources, basic coding assistance, and boosting AI education efforts. Los Alamos National Laboratory: The laboratory leverages ChatGPT Enterprise for scientific research and innovation. This includes work within its Bioscience Division, which is evaluating ways GPT-4o can safely advance bioscientific research in laboratory settings. State of Minnesota: Minnesota’s Enterprise Translations Office uses ChatGPT Team to provide faster, more accurate translation services to multilingual communities across the state. The integration has resulted in significant cost savings and reduced turnaround times. Commonwealth of Pennsylvania: Employees in Pennsylvania’s pioneering AI pilot programme reported that ChatGPT Enterprise helped them reduce routine task times, such as analysing project requirements, by approximately 105 minutes per day on days they used the tool. These early use cases demonstrate the transformative potential of AI applications across various levels of government. Beyond delivering tangible improvements to government workflows, OpenAI seeks to foster public trust in artificial intelligence through collaboration and transparency. The company said it is committed to working closely with government agencies to align its tools with shared priorities and democratic values. “We look forward to collaborating with government agencies to enhance service delivery to the American people through AI,” OpenAI stated. As other governments across the globe begin adopting similar technologies, America’s proactive approach may serve as a model for integrating AI into the public sector while safeguarding against risks. Whether supporting administrative workflows, research initiatives, or language services, ChatGPT Gov stands as a testament to the growing role AI will play in shaping the future of effective governance. (Photo by Dave Sherrill) See also: Yiannis Antoniou, Lab49: OpenAI Operator kickstarts era of browser AI agents Want to learn more about AI and big data from industry leaders? Check out AI & Big Data Expo taking place in Amsterdam, California, and London. The comprehensive event is co-located with other leading events including Intelligent Automation Conference, BlockX, Digital Transformation Week, and Cyber Security & Cloud Expo. Explore other upcoming enterprise technology events and webinars powered by TechForge here. The post ChatGPT Gov aims to modernise US government agencies appeared first on AI News. View the full article
-
Ericsson has launched Cognitive Labs, a research-driven initiative dedicated to advancing AI for telecoms. Operating virtually rather than from a single physical base, Cognitive Labs will explore AI technologies such as Graph Neural Networks (GNNs), Active Learning, and Large-Scale Language Models (LLMs). According to Ericsson, these innovations form the backbone of the company’s solutions for the next generation of mobile communications and signal the company’s commitment to extending AI’s transformative potential to other sectors, such as healthcare. The initiative places a strong emphasis on open collaboration, engaging with the open-source community to further the development of AI technologies for wider societal benefit. “Cognitive Labs represents our commitment to lead innovation in AI and make a significant contribution to the open source community,” said Jean-Christophe Laneri, Head of Cognitive Network Solutions at Ericsson. “Our mission is to create world-class research that can benefit the academic community and enhances our products to better serve our customers.” A triad of Ericsson AI labs Central to the Cognitive Labs initiative are three distinct research arms, each focused on a specialised area of AI: GAI Lab (Geometric Artificial Intelligence Lab): This lab explores Geometric AI, emphasising explainability in geometric learning, graph generation, and temporal GNNs. Ericsson anticipates this work will make significant contributions to areas like drug discovery and enable the creation of more efficient pharmaceuticals. MLR Lab (Machine Learning and Reasoning Lab): Focusing on training model optimisation and reinforcement learning, this lab aims to advance energy-efficient training for AI models and support the creation of digital twins that simulate physical realities. The potential benefits include reductions in time and energy costs, key priorities in sustainable AI development. FAI Lab (Fundamental Artificial Intelligence Lab): Dedicated to foundational AI models like Large-Scale Language Models, this lab seeks to shape the future of AI applications in telecoms. Its primary mission is to automate processes and improve technological efficiencies crucial to the sector. By organising Cognitive Labs into these specialised units, Ericsson looks to tackle diverse challenges while maintaining a cohesive focus on leveraging AI for wide-ranging applications. Open-source collaboration In line with its ties to the open-source community, Cognitive Labs prioritises the development of reusable AI libraries. This accessibility helps both researchers and industry professionals accelerate progress across multiple domains—from healthcare to digital communications. Additionally, Ericsson hopes to attract leading global researchers and data scientists to its fold, enhancing its credentials as a leader in AI innovation. Spain is emerging as a cornerstone for Cognitive Labs, with Ericsson Spain playing a leading role due to its robust R&D activities in Madrid and Málaga. This focus has been bolstered by a new agreement with Universidad Pontificia Comillas, specifically with its School of Engineering (Comillas ICAI). The alliance between Ericsson and ICAI is set to accelerate AI research through joint publications and active participation in open-source initiatives. Teams of Ericsson’s top engineers and data scientists will closely collaborate with the university’s research teams to drive innovation at both national and European levels. “The close collaboration between top data scientists and engineers from Ericsson and ICAI’s teams will enable Ericsson Spain to lead innovation at the national and European levels, strengthening its position as a benchmark in technology and development,” Ericsson stated. The partnership underscores Ericsson’s commitment not only to its Cognitive Labs initiative but also to developing Spain’s expertise in cutting-edge AI technologies. Through these R&D efforts, the company seeks to reinforce its position as a leading innovator in telecoms and beyond. With AI set to play a critical role in shaping the next generation of mobile communications, Ericsson’s Cognitive Labs marks a vital step forward—not only for the company but also for industries and communities that stand to benefit from its far-reaching, transformative potential. See also: World Economic Forum unveils blueprint for equitable AI Want to learn more about AI and big data from industry leaders? Check out AI & Big Data Expo taking place in Amsterdam, California, and London. The comprehensive event is co-located with other leading events including Intelligent Automation Conference, BlockX, Digital Transformation Week, and Cyber Security & Cloud Expo. Explore other upcoming enterprise technology events and webinars powered by TechForge here. The post Ericsson launches Cognitive Labs to pioneer telecoms AI research appeared first on AI News. View the full article
-
DeepSeek is grappling with service disruptions and restricting new account sign-ups to combat what it describes as “large-scale malicious attacks.” The ******** firm’s chat app, which recently soared to the top of Apple’s App Store, issued a notice on its website stating that only users with China-based phone numbers (+86) would be permitted to register for the foreseeable future. In the notice, DeepSeek said: “DeepSeek’s online services have recently faced large-scale malicious attacks. To ensure continued service, registration is temporarily limited to +86 phone numbers. Existing users can log in as usual.” As of writing, sign-ups have reopened with a warning that “registration may be busy” and to please wait and try again. Despite the challenges, existing users remain unaffected in terms of access. DeepSeek’s status page reported ongoing issues on Monday morning, adding to a turbulent day for the breakthrough AI platform. DeepSeek faces test after explosive growth DeepSeek has quickly become a disruptive force in the race for AI supremacy. In addition to gaining widespread user adoption, the app’s powerful open-source AI model has sparked waves of concern among US-based Big Tech firms. The app’s strength lies in its ability to deliver robust AI performance on less-advanced chips, creating a more cost-effective and accessible solution compared to high-profile rivals such as OpenAI’s ChatGPT. This key differentiation shook investor confidence on Monday, leading to a significant dip in share prices for major US technology firms. Industry watchers suggest that such shocks may become more frequent as innovative competitors like DeepSeek challenge the dominance of traditional tech players. Nigel Green, CEO of global financial advisory firm deVere Group, commented: “China’s technological advances, particularly in AI, are eroding the US’s ability to use tariffs as a tool to maintain global supremacy. “The balance of power is shifting, and Washington must recognise that it can’t always dictate terms to Beijing as it once did. This new reality will have far-reaching consequences for investors and policymakers. “AI will be the defining technology of our time, and the race to dominate it will shape global markets for decades to come.” Green went on to highlight the potential for non-traditional players and regions to seize the AI crown. “Traditional tech giants are no longer the guaranteed winners. The focus must now shift to sectors and regions that are driving the next wave of innovation. This includes not only AI but also the critical infrastructure needed to support and secure it.” The latest developments come against the broader canvas of growing competition between China and the US in the domain of AI and emerging technologies. Despite export restrictions on US hardware, Beijing’s AI sector has been powering ahead—spurred by significant government investments and a burgeoning ecosystem of innovative startups. The rise of apps like DeepSeek signals that the playing field is no longer tilted decisively in favour of Silicon Valley. For years, US companies have been buoyed by leading semiconductor technologies from domestic firms like NVIDIA. China’s ability to deliver competitive AI capabilities on less-advanced hardware could mark a significant turning point. However, while AI innovation is ramping up globally, DeepSeek’s struggles highlight the growing pains that can accompany explosive growth. (Photo by Markus Spiske) See also: Yiannis Antoniou, Lab49: OpenAI Operator kickstarts era of browser AI agents Want to learn more about AI and big data from industry leaders? Check out AI & Big Data Expo taking place in Amsterdam, California, and London. The comprehensive event is co-located with other leading events including Intelligent Automation Conference, BlockX, Digital Transformation Week, and Cyber Security & Cloud Expo. Explore other upcoming enterprise technology events and webinars powered by TechForge here. The post DeepSeek restricts sign-ups amid ‘large-scale malicious attacks’ appeared first on AI News. View the full article
-
OpenAI has unveiled Operator, a tool that integrates seamlessly with web browsers to perform tasks autonomously. From filling out forms to ordering groceries, Operator promises to simplify repetitive online activities by interacting directly with websites through clicks, typing, and scrolling. Designed around a new model called the Computer-Using Agent (CUA), Operator combines GPT-4o’s vision recognition with advanced reasoning capabilities—allowing it to function as a virtual “human-in-the-browser.” Yet, for all its innovation, industry experts see room for refinement. Yiannis Antoniou, Head of AI, Data, and Analytics at specialist consultancy Lab49, shared his insights on Operator’s significance and positioning in the competitive landscape of agent AI systems. Agentic AI through a familiar interface “OpenAI’s announcement of Operator, its latest foray into the agentic AI wars, is both fascinating and incomplete,” said Antoniou, who has over two decades of experience designing AI systems for financial services firms. “Clearly influenced by Anthropic Claude’s Computer Use system, introduced back in October, Operator streamlines the experience by removing the need for complex infrastructure and focusing on a familiar interface: the browser.” By designing Operator to operate within an environment users already understand, the web browser, OpenAI sidesteps the need for bespoke APIs or integrations. “By leveraging the world’s most popular interface, OpenAI enhances the user experience and captures immediate interest from the general public. This browser-centric approach creates significant potential for widespread adoption, something Anthropic – despite its early-mover advantage – has struggled to achieve.” Unlike some competing systems that may feel technical or niche in their application, Operator’s browser-focused framework lowers the barrier to entry and is a step forward in OpenAI’s efforts to democratise AI. Unique take on usability and security One of the hallmarks of Operator is its emphasis on adaptability and security, implemented through human-in-the-loop protocols. Antoniou acknowledged these thoughtful usability features but noted that more work is needed. “Architecturally, Operator’s browser integration closely mirrors Claude’s system. Both involve taking screenshots of the user’s browser and sending them for analysis, as well as controlling the screen via virtual keystrokes and mouse movements. However, Operator introduces thoughtful usability touches. “Features like custom instructions for specific websites add a layer of personalisation, and the emphasis on human-in-the-loop safeguards against unauthorised actions – such as purchases, sending emails, or applying for jobs – demonstrate OpenAI’s awareness of potential security risks posed by malicious websites, but more work is clearly needed to make this system widely safe across a variety of scenarios.” OpenAI has implemented a multi-layered safety framework for Operator, including takeover mode for secure inputs, user confirmations prior to significant actions, and monitoring systems to detect adversarial behavior. Furthermore, users can delete browsing data and manage privacy settings directly within the tool. However, Antoniou emphasised that these measures are still evolving—particularly as Operator encounters complex or sensitive tasks. OpenAI Operator further democratises AI Antoniou also sees the release of Operator as a pivotal moment for the consumer AI landscape, albeit one that is still in its early stages. “Overall, this is an excellent first attempt at building an agentic system for everyday users, designed around how they naturally interact with technology. As the system develops – with added capabilities and more robust security controls – this limited rollout, priced at $200/month, will serve as a testing ground. “Once matured and extended to lower subscription tiers and the free version, Operator has the potential to usher in the era of consumer-facing agents, further democratising AI and embedding it into daily life.” Designed initially for Pro users at a premium price point, Operator provides OpenAI with an opportunity to learn from early adopters and refine its capabilities. Antoniou noted that while $200/month might not yet justify the system’s value for most users, investment in making Operator more powerful and accessible could lead to significant competitive advantages for OpenAI in the long run. “Is it worth $200/month? Perhaps not yet. But as the system evolves, OpenAI’s moat will grow, making it harder for competitors to catch up. Now, the challenge shifts back to Anthropic and Google—both of whom have demonstrated similar capabilities in niche or engineering-focused products—to respond and stay in the game,” he concludes. As OpenAI continues to fine-tune Operator, the potential to revolutionise how people interact with technology becomes apparent. From collaborations with companies like Instacart, DoorDash, and Uber to use cases in the public sector, Operator aims to balance innovation with trust and safety. While early limitations and pricing may deter widespread adoption for now, these hurdles might only be temporary as OpenAI commits to enhancing usability and accessibility over time. See also: OpenAI argues against ChatGPT data deletion in Indian court Want to learn more about AI and big data from industry leaders? Check out AI & Big Data Expo taking place in Amsterdam, California, and London. The comprehensive event is co-located with other leading events including Intelligent Automation Conference, BlockX, Digital Transformation Week, and Cyber Security & Cloud Expo. Explore other upcoming enterprise technology events and webinars powered by TechForge here. The post Yiannis Antoniou, Lab49: OpenAI Operator kickstarts era of browser AI agents appeared first on AI News. View the full article
-
When it comes to movies buzzing with Oscar potential, Brady Corbet’s The Brutalist is a standout this awards season. The visually stunning drama transports viewers to the post-World War II era, unravelling the story of László Tóth, played by Adrien Brody. Tóth, a fictional Hungarian-Jewish architect, starts over in the United States after being forced to leave his family behind as he emigrates. Beyond its vintage allure, something modern brews in the background: the use of AI. Specifically, AI was employed to refine Brody’s and co-star Felicity Jones’ Hungarian pronunciation. The decision has sparked lively debates about technology’s role in film-making. The role of AI in The Brutalist According to Dávid Jancsó, the film’s editor, the production team turned to Respeecher, an AI software developed by a Ukrainian company, to tweak the actors’ Hungarian dialogue. Speaking to RedShark News (as cited by Mashable SEA), Jancsó explained that Hungarian – a Uralic language known for its challenging sounds – was a significant hurdle for the actors, despite their talent and dedication. Respeecher’s software isn’t magic, but just a few years ago, it would have seemed wondrous. It creates a voice model based on a speaker’s characteristics and adjusts specific elements, like pronunciation. In this case, it was used to fine-tune the letter and vowel sounds that Brody and Jones found tricky. Most of the corrections were minimal, with Jancsó himself providing some replacement sounds to preserve the authenticity of the performances. “Most of their Hungarian dialogue has a part of me talking in there,” he joked, emphasising the care taken to maintain the actors’ original delivery. Respeecher: AI behind the scenes The is not Respeecher’s first foray into Hollywood. The software is known for restoring iconic voices like that of Darth Vader for the Obi-Wan Kenobi series, and has recreated Edith Piaf’s voice for an upcoming biopic. Outside of film, Respeecher has helped to preserve endangered languages like Crimean Tatar. For The Brutalist, the AI tool wasn’t just a luxury – it was a time and budget saver. With so much dialogue in Hungarian, manually editing every line would have required painstaking, manual work. Jancsó said that using AI sped up the process significantly, an important factor given the film’s modest $10 million budget. Beyond voice: AI’s other roles in the film AI was also used in other aspects of the production process, used for example to generate some of Tóth’s architectural drawings and complete buildings in the film’s Venice Biennale sequence. However, director Corbet has clarified that these images were not fully AI-generated; instead, the AI was used for specific background elements. Corbet and Jancsó have been candid about their perspectives on AI in film-making. Jancsó sees it as a valuable tool, saying, “There’s nothing in the film using AI that hasn’t been done before. It just makes the process a lot faster.” Corbet added that the software’s purpose was to enhance authenticity, not replace the actors’ hard work. A broader conversation The debate surrounding AI in the film industry isn’t new. From script-writing to music production, concerns about generative AI’s impact were central to the 2023 Writers Guild of America (WGA) and SAG-AFTRA strikes. Although agreements have been reached to regulate the use of AI, the topic remains a hot-button issue. The Brutalist awaits a possible Oscar nomination. From its story line to its cinematic style, the film wears its ambition on its sleeve. It’s not just a celebration of the postwar Brutalist architectural movement, it’s also a nod to classic American cinema. Shot in the rarely used VistaVision format, the film captures the grandeur of mid-20th-century film-making. Adding to its nostalgic charm, it includes a 15-minute intermission during its epic three-and-a-half-hour runtime. Yet the use of AI has given a new dimension to the ongoing conversation about AI in the creative industry. Whether people see AI as a betrayal of craftsmanship or an exciting innovative tool that can add to a final creation, one thing is certain: AI continues to transform how stories are delivered on screen. See also: AI music sparks new copyright battle in US courts Want to learn more about AI and big data from industry leaders? Check out AI & Big Data Expo taking place in Amsterdam, California, and London. The comprehensive event is co-located with other leading events including Intelligent Automation Conference, BlockX, Digital Transformation Week, and Cyber Security & Cloud Expo. Explore other upcoming enterprise technology events and webinars powered by TechForge here. The post How AI helped refine Hungarian accents in The Brutalist appeared first on AI News. View the full article
-
OpenAI, the powerhouse behind ChatGPT, is ramping up efforts to dominate the enterprise market with a suite of AI tools tailored for business users. The company recently revealed its plans to introduce a series of enhancements designed to make AI integration seamless for companies of all sizes. This includes updates to its flagship AI agent technology, expected to transform workplace productivity by automating complex workflows, from financial analysis to customer service. “Businesses are looking for solutions that go beyond surface-level assistance. Our agents are designed to provide in-depth, actionable insights,” said Sarah Friar, CFO of OpenAI. “This is particularly relevant as enterprises seek to streamline operations in today’s competitive landscape.” OpenAI’s corporate strategy builds on its ongoing collaborations with tech leaders such as Microsoft, which has already integrated OpenAI’s technology into its Azure cloud platform. Analysts say these partnerships position OpenAI to rival established enterprise solutions providers like Salesforce and Oracle. AI research assistant tools As part of its enterprise-focused initiatives, OpenAI is emphasising the development of AI research tools that cater to specific industries. For instance, its AI models are being trained on legal and medical data to create highly specialised assistants that could redefine research-intensive sectors. This focus aligns with the broader market demand for AI-driven solutions that enhance decision-making and efficiency. Infrastructure for expansion OpenAI’s rapid growth strategy is supported by a robust infrastructure push. The company has committed to building state-of-the-art data centers in Europe and Asia, aiming to lower latency and improve service reliability for global users. These investments reflect OpenAI’s long-term vision of becoming a critical enabler in the AI-driven global economy. Challenges and issues However, challenges persist. The company faces mounting pressure from regulators concerned about data privacy and the ethical implications of deploying powerful AI tools. Critics also question the sustainability of OpenAI’s ambitious growth targets, given its significant operational costs and strong competition from other tech giants. Despite these hurdles, OpenAI remains optimistic about its trajectory. With plans to unveil its expanded portfolio at the upcoming Global AI Summit, the company is well-positioned to strengthen its foothold in the burgeoning AI enterprise market. (Editor’s note: This article is sponsored by AI Tools Network) See also: OpenAI argues against ChatGPT data deletion in Indian court Want to learn more about AI and big data from industry leaders? Check out AI & Big Data Expo taking place in Amsterdam, California, and London. The comprehensive event is co-located with other leading events including Intelligent Automation Conference, BlockX, Digital Transformation Week, and Cyber Security & Cloud Expo. Explore other upcoming enterprise technology events and webinars powered by TechForge here. The post OpenAI targets business sector with advanced AI tools appeared first on AI News. View the full article
-
[AI]OpenAI argues against ChatGPT data deletion in Indian court
ChatGPT posted a topic in World News
OpenAI has argued in an Indian court that removing the training data behind ChatGPT service would clash with its legal obligations in the United States. The statement was issued in response to a lawsuit filed by Indian news agency ANI, which accused the AI business of using its content without permission. The Microsoft-backed AI giant stated that Indian courts lack jurisdiction in the case since OpenAI has no offices nor operations in the country. In its January 10 filing to the Delhi High Court, OpenAI emphasised that it is already defending similar lawsuits in the US, where it is required to preserve its training data during ongoing litigation. The case, filed by ANI in November, is one of India’s most closely-watched lawsuits involving the use of AI. ANI alleges that OpenAI utilised its published content without authorisation to train ChatGPT and is demanding the deletion of its data from the company’s systems. A global battle over copyright and AI OpenAI is no stranger to such disputes, facing a wave of lawsuits from copyright holders worldwide. In the US, the New York Times filed a similar case against the company, accusing it of misusing its content. OpenAI has consistently denied such allegations, claiming its systems rely on the fair use of publicly available data. During a November hearing in Delhi, OpenAI told the court it would no longer use ANI’s content. However, ANI argued that its previously published material remains stored in ChatGPT’s repositories and must be deleted. In its rebuttal, OpenAI highlighted that it is legally obligated under US law to retain training data while related cases are pending. “The company is under a legal obligation, under the laws of the United States, to preserve, and not delete, the said training data,” OpenAI stated in its filing. Jurisdiction dispute OpenAI also argued that the relief ANI is seeking falls outside the jurisdiction of Indian courts. It pointed out that the company has “no office or permanent establishment in India,” and its servers, which store ChatGPT’s training data, are located outside the country. ANI, which is partially owned by Reuters, countered the claim, saying the Delhi court has the authority to hear the case and that it will file a detailed response. A Reuters spokesperson declined to comment on proceedings, but has stated that the agency has no involvement in ANI’s business operations. Concerns over competition ANI has also expressed concern about unfair competition, citing OpenAI’s partnerships with major news organisations like Time Magazine, The Financial Times, and France’s Le Monde. ANI says that these agreements give OpenAI an edge. The agency further claimed that ChatGPT reproduces verbatim or similar excerpts of its works in response to user prompts. OpenAI, on the other hand, claimed that ANI deliberately used its own articles as prompts to “manipulate ChatGPT” to file the lawsuit. The case is scheduled to be heard by the Delhi High Court on January 28. Meanwhile, OpenAI is transitioning from a non-profit to a for-profit company, raising $6.6 billion last year. In recent months, OpenAI has secured high-profile deals with media outlets from around the world, highlighting its efforts to strengthen its commercial partnerships while managing regulatory concerns worldwide. (Photo by Unsplash) See also: DeepSeek-R1 reasoning models rival OpenAI in performance Want to learn more about AI and big data from industry leaders? Check out AI & Big Data Expo taking place in Amsterdam, California, and London. The comprehensive event is co-located with other leading events including Intelligent Automation Conference, BlockX, Digital Transformation Week, and Cyber Security & Cloud Expo. Explore other upcoming enterprise technology events and webinars powered by TechForge here. The post OpenAI argues against ChatGPT data deletion in Indian court appeared first on AI News. View the full article -
The World Economic Forum (WEF) has released a blueprint outlining how AI can drive inclusivity in global economic growth and societal progress. However, it also highlights the challenges in ensuring its benefits are equitably distributed across all nations and peoples. Developed in partnership with KPMG, the blueprint offers nine strategic objectives to support government leaders, organisations, and key stakeholders through every phase of the AI lifecycle – from innovation to deployment – at local, national, and international levels. These strategies aim to bridge disparities in AI access, infrastructure, advanced computing, and skill development to promote sustainable, long-term growth. Cathy Li, Head of AI, Data, and the Metaverse at the WEF, said: “Leveraging AI for economic growth and societal progress is a shared goal, yet countries and regions have very different starting points. “This blueprint serves as a compass, guiding decision-makers toward impact-oriented collaboration and practical solutions that can unlock AI’s full potential.” Call for regional collaboration and local empowerment Central to the ‘Blueprint for Intelligent Economies’ is the belief that successful AI adoption must reflect the specific needs of local communities—with strong leadership and collaboration among governments, businesses, entrepreneurs, civil society organisations, and end users. Solly Malatsi, South Africa’s Minister of Communications and Digital Technologies, commented: “The significant potential of AI remains largely untapped in many regions worldwide. Establishing an inclusive and competitive AI ecosystem will become a crucial priority for all nations. “Collaboration among multiple stakeholders at the national, regional, and global levels will be essential in fostering growth and prosperity through AI for everyone.” By tailoring approaches to reflect geographic and cultural nuances, the WEF report suggests nations can create AI systems that address local challenges while also providing a robust bedrock for innovation, investment, and ethical governance. Case studies from nations at varying stages of AI maturity are used throughout the report to illustrate practical, scalable solutions. For example, cross-border cooperation on shared AI frameworks and pooled resources (such as energy or centralised databanks) is highlighted as a way to overcome resource constraints. Public-private subsidies to make AI-ready devices more affordable present another equitable way forward. These mechanisms aim to lower barriers for local businesses and innovators, enabling them to adopt AI tools and scale their operations. Hatem Dowidar, Chief Executive Officer of E&, said: “All nations have a unique opportunity to advance their economic and societal progress through AI. This requires a collaborative approach of intentional leadership from governments supported by active engagement with all stakeholders at all stages of the AI journey. “Regional and global collaborations remain fundamental pathways to address shared challenges and opportunities, ensure equitable access to key AI capabilities, and responsibly maximise its transformative potential for a lasting value for all.” Priority focus areas While the blueprint features nine strategic objectives, three have been singled out as priority focus areas for national AI strategies: Building sustainable AI infrastructure Resilient, scalable, and environmentally sustainable AI infrastructure is essential for innovation. However, achieving this vision will require substantial investment, energy, and cross-sector collaboration. Nations must coordinate efforts to ensure that intelligent economies grow in both an equitable and eco-friendly manner. Curating diverse and high-quality datasets AI’s potential hinges on the quality of the data it can access. This strategic objective addresses barriers such as data accessibility, imbalance, and ownership. By ensuring that datasets are inclusive, diverse, and reflective of local languages and cultures, developers can create equitable AI models that avoid bias and meet the needs of all communities. Establishing robust ethical and safety guardrails Governance frameworks are critical for reducing risks like misuse, bias, and ethical breaches. By setting high standards at the outset, nations can cultivate trust in AI systems, laying the groundwork for responsible deployment and innovation. These safeguards are especially vital for promoting human-centred AI that benefits all of society. The overall framework outlined in the report has three layers: Foundation layer: Focuses on sustainable energy, diverse data curation, responsible AI infrastructure, and efficient investment mechanisms. Growth layer: Embeds AI into workflows, processes, and devices to accelerate sectoral adoption and boost innovation. People layer: Prioritises workforce skills, empowerment, and ethical considerations, ensuring that AI shapes society in a beneficial and inclusive way. A blueprint for global AI adoption The Forum is also championing a multi-stakeholder approach to global AI adoption, blending public and private collaboration. Policymakers are being encouraged to implement supportive legislation and incentives to spark innovation and broaden AI’s reach. Examples include lifelong learning programmes to prepare workers for the AI-powered future and financial policies that enable greater technology access in underserved regions. The WEF’s latest initiative reflects growing global recognition that AI will be a cornerstone of the future economy. However, it remains clear that the benefits of this transformative technology will need to be shared equitably to drive societal progress and ensure no one is left behind. The Blueprint for Intelligent Economies provides a roadmap for nations to harness AI while addressing the structural barriers that could otherwise deepen existing inequalities. By fostering inclusivity, adopting robust governance, and placing communities at the heart of decision-making, the WEF aims to guide governments, businesses, and innovators toward a sustainable and intelligent future. See also: *** Government signs off sweeping AI action plan Want to learn more about AI and big data from industry leaders? Check out AI & Big Data Expo taking place in Amsterdam, California, and London. The comprehensive event is co-located with other leading events including Intelligent Automation Conference, BlockX, Digital Transformation Week, and Cyber Security & Cloud Expo. Explore other upcoming enterprise technology events and webinars powered by TechForge here. The post World Economic Forum unveils blueprint for equitable AI appeared first on AI News. View the full article
-
AI coding tools leverage machine learning, deep learning, and natural language processing to assist developers in writing and optimising code. These tools are trained on vast code repositories and datasets, allowing them to analyse programming patterns and provide intelligent recommendations. Types of AI coding tools AI-powered coding tools can be categorised into several types based on their functionality: AI code completion tools — Provide real-time suggestions and auto-complete lines of code. AI code generators — Generate full scripts, functions, or even applications based on natural language prompts. AI debugging and error detection tools — Identify syntax errors, logical issues, and security vulnerabilities. AI test automation tools — Create and execute test cases with minimal human intervention. AI code optimisation Tools — Improve performance by suggesting better coding practices. AI security and compliance tools — Analyse code for vulnerabilities and help developers follow security best practices. AI documentation generators — Automate inline comments, API documentation, and explanations. By incorporating these tools into their workflow, developers can write cleaner, more efficient code and reduce the time spent on repetitive tasks. Best free AI coding tools for 2025 Below, we explore seven of the best free AI coding tools available today and provide an analysis of their features, benefits, and how they can enhance the software development process. 1. Qodo Qodo is an AI-powered coding assistant designed to help developers generate, optimise, and debug code easily. It offers real-time code suggestions, detects syntax errors, and improves the overall quality of the codebase. Key features: Intelligent code completion: Predicts and suggests relevant code snippets. AI-powered debugging: Identifies bugs and logical errors in real-time. Code optimisation: Suggests performance improvements and cleaner code structures. Multi-language support: Works with multiple programming languages. Benefits: Qodo streamlines software development by reducing manual effort in writing and reviewing code. It is particularly beneficial for new developers who need guidance on best coding practices and experienced programmers looking to improve efficiency. Use cases: Writing clean and optimised code. Debugging and improving code quality. Learning new programming concepts with AI-driven recommendations. 2. Kite Kite was a popular AI-powered autocomplete tool that provided developers with real-time code suggestions and documentation assistance. Although it has been discontinued, it significantly influenced modern AI coding assistants. Key features: Python-focused autocompletion: Provided predictive code completions. Inline documentation: Showed documentation snippets inside the IDE. Machine learning-based suggestions: Improved over time with usage. Benefits: Despite its discontinuation, Kite demonstrated the power of AI in streamlining software development, influencing newer AI-powered coding tools available today. Use cases: Assisted Python developers with intelligent code suggestions. Provided real-time documentation to reduce context switching. 3. Amazon CodeWhisperer Amazon CodeWhisperer is a free AI-powered coding assistant that provides intelligent code completions and recommendations based on natural language input. It integrates seamlessly with AWS services, making it an excellent tool for cloud-based development. Key features: Real-time code suggestions: Predicts and generates relevant code snippets. AWS cloud integration: Works with AWS Lambda, EC2, and other services. Multi-language support: Supports Python, JavaScript, and more. Benefits: Amazon CodeWhisperer boosts productivity by offering context-aware code recommendations, making it ideal for developers working with cloud-based applications. Use cases: Writing serverless applications on AWS. Generating API integrations with minimal manual coding. Reducing development time for cloud-based applications. 4. Ponicode Ponicode is an AI-powered unit testing and code quality analysis tool that ensures high test coverage and optimised code. Key features: AI-generated unit tests: Automates test case creation. Code quality analysis: Detects inefficiencies in the code. Multi-language support: Works with JavaScript, Python, and more. Benefits: Ponicode helps developers write reliable, maintainable code by automating testing and quality checks, making it an excellent tool for teams prioritising test-driven development (TDD). Use cases: Ensuring code coverage with AI-generated test cases. Automating software testing processes. 5. IntelliCode Microsoft IntelliCode enhances coding productivity by offering AI-powered autocomplete, refactoring suggestions, and best coding practices based on thousands of open-source projects. Key features: Context-aware code recommendations: Learns from open-source projects. Automated code refactoring: Suggests optimised coding solutions. Supports multiple languages: Works with Python, Java, C++, and more. Benefits: IntelliCode enables developers to write maintainable code while minimising syntax errors and reducing repetitive coding tasks. Use cases: Improving team productivity by enforcing best coding practices. Optimising legacy codebases. 6. YOGI Bot YOGI Bot is an AI-powered chatbot that assists developers with debugging, code explanations, and best practice recommendations. Key features: AI-driven code assistance: Provides real-time support for debugging and explanations. Multi-language support: Works across various programming languages. Benefits: YOGI Bot is useful for both new and experienced developers who need immediate coding assistance and explanations. Use cases: Learning programming concepts interactively. Troubleshooting coding errors with AI-driven assistance. 7. CodeT5 CodeT5, developed by Salesforce Research, is an AI-powered code generation and translation model that helps developers write high-quality code efficiently. Key features: AI-generated code snippets: Produces entire code blocks. Code translation and optimisation: Helps improve existing code. Benefits: CodeT5 streamlines development by reducing manual effort and improving code quality. Use cases: Automating coding tasks for web and software development. Enhancing productivity with AI-powered suggestions. Why use AI coding tools? AI coding tools are gaining popularity due to their ability to streamline development processes, reduce coding errors, and enhance productivity. Here’s why developers should consider integrating AI into their workflow: Increased efficiency AI-powered tools speed up coding by suggesting relevant code snippets, autocompleting functions, and automating repetitive tasks. This allows developers to focus on solving complex problems rather than spending time on boilerplate code. Improved code quality AI-driven code review tools analyse code for potential errors, security vulnerabilities, and performance issues, ensuring high-quality and optimised code. Reduced debugging time Manually finding and fixing bugs can be time-consuming. AI debugging tools help detect issues in real-time and provide potential fixes, reducing time spent on troubleshooting. Enhanced collaboration AI-powered tools facilitate team collaboration by providing code suggestions, and documentation, making it easier for teams to work together efficiently. Automated testing and deployment AI-driven test automation ensures that applications are tested before deployment, reducing manual effort and potentially speeding up the software release cycle. Better security Security-focused AI tools can help identify vulnerabilities and suggest best practices, helping developers write more secure code that complies with industry standards. Accessibility for beginners AI coding assistants help beginners learn programming by providing real-time feedback, suggesting improvements, and simplifying complex coding concepts. 7 top free AI coding tools AI coding tools leverage machine learning, deep learning, and natural language processing to assist developers in writing and optimising code. These tools are trained on vast code repositories and datasets, allowing them to analyse programming patterns and provide intelligent recommendations. Types of AI coding tools AI-powered coding tools can be categorised into several types based on their functionality: AI code completion tools — Provide real-time suggestions and auto-complete lines of code. AI code generators — Generate full scripts, functions, or even applications based on natural language prompts. AI debugging and error detection tools — Identify syntax errors, logical issues, and security vulnerabilities. AI test automation tools — Create and execute test cases with minimal human intervention. AI code optimisation Tools — Improve performance by suggesting better coding practices. AI security and compliance tools — Analyse code for vulnerabilities and help developers follow security best practices. AI documentation generators — Automate inline comments, API documentation, and explanations. By incorporating these tools into their workflow, developers can write cleaner, more efficient code and reduce the time spent on repetitive tasks. (Image source: Unsplash) The post 7 top free AI coding tools appeared first on AI News. View the full article
-
According to Rodolphe Malaguti, Product Strategy and Transformation at Conga, poor data structures and legacy systems are hindering the potential of AI in transforming public services. Taxpayer-funded services in the ***, from the NHS to local councils, are losing out on potential productivity savings of £45 billion per year due to an overwhelming reliance on outdated technology—a figure equivalent to the total cost of running every primary school in the country for a year. A report published this week highlights how nearly half of public services are still not accessible online. This forces British citizens to engage in time-consuming and frustrating processes such as applying for support in person, enduring long wait times on hold, or travelling across towns to council offices. Public sector workers are similarly hindered by inefficiencies, such as sifting through mountains of physical letters, which slows down response times and leaves citizens to bear the brunt of government red tape. “As this report has shown, there is clearly a gap between what the government and public bodies intend to achieve with their digital projects and what they actually deliver,” explained Malaguti. “The public sector still relies heavily upon legacy systems and has clearly struggled to tackle existing poor data structures and inefficiencies across key departments. No doubt this has had a clear impact on decision-making and hindered vital services for vulnerable citizens.” The struggles persist even in deeply personal and critical scenarios. For example, the current process for registering a death still demands a physical presence, requiring grieving individuals to manage cumbersome bureaucracy while mourning the loss of a loved one. Other outdated processes unnecessarily burden small businesses—one striking example being the need to publish notices in local newspapers simply to purchase a lorry licence, creating further delays and hindering economic growth. A lack of coordination between departments amplifies these challenges. In some cases, government bodies are using over 500 paper-based processes, leaving systems fragmented and inefficient. Vulnerable individuals suffer disproportionately under this disjointed framework. For instance, patients with long-term health conditions can be forced into interactions with up to 40 different services, repeating the same information as departments repeatedly fail to share data. “The challenge is that government leaders have previously focused on technology and online interactions, adding layers to services whilst still relying on old data and legacy systems—this has ultimately led to inefficiencies across departments,” added Malaguti. “Put simply, they have failed to address existing issues or streamline their day-to-day operations. It is critical that data is more readily available and easily shared between departments, particularly if leaders are hoping to employ new technology like AI to analyse this data and drive better outcomes or make strategic decisions for the public sector as a whole.” Ageing Infrastructure: High costs and security risks The report underscores that ageing infrastructure comes at a steep financial and operational cost. More than one-in-four digital systems used across the ***’s central government are outdated, with this figure ballooning to 70 percent in some departments. Maintenance costs for legacy systems are significantly higher, up to three-to-four times more, compared to keeping technology up-to-date. Furthermore, a growing number of these outdated systems are now classified as “red-rated” for reliability and cybersecurity risk. Alarmingly, NHS England experienced 123 critical service outages last year alone. These outages often meant missed appointments and forced healthcare workers to resort to paper-based systems, making it harder for patients to access care when they needed it most. Malaguti stresses that addressing such challenges goes beyond merely upgrading technology. “The focus should be on improving data structure, quality, and timeliness. All systems, data, and workflows must be properly structured and fully optimised prior to implementation for these technologies to be effective. Public sector leaders should look to establish clear measurable objectives, as they continue to improve service delivery and core mission impacts.” Transforming public services In response to these challenges, Technology Secretary Peter Kyle is announcing an ambitious overhaul of public sector technology to usher in a more modern, efficient, and accessible system. Emphasising the use of AI, digital tools, and “common sense,” the goal is to reform how public services are designed and delivered—streamlining operations across local government, the NHS, and other critical departments. A package of tools known as ‘Humphrey’ – named after the fictional Whitehall official in popular BBC drama ‘Yes, Minister’ – is set to be made available to all civil servants soon, with some available today. Humphrey includes: Consult: Analyses the thousands of responses received during government consultations within hours, presenting policymakers and experts with interactive dashboards to directly explore public feedback. Parlex: A tool that enables policymakers to search and analyze decades of parliamentary debate, helping them refine their thinking and manage bills more effectively through both the Commons and the Lords. Minute: A secure AI transcription service that creates customisable meeting summaries in the formats needed by public servants. It is currently being used by multiple central departments in meetings with ministers and is undergoing trials with local councils. Redbox: A generative AI tool tailored to assist civil servants with everyday tasks, such as summarising policies and preparing briefings. Lex: A tool designed to support officials in researching the law by providing analysis and summaries of relevant legislation for specific, complex issues. The new tools and changes will help to tackle the inefficiencies highlighted in the report while delivering long-term cost savings. By reducing the burden of administrative tasks, the reforms aim to enable public servants, such as doctors and nurses, to spend more time helping the people they serve. For businesses, this could mean faster approvals for essential licences and permits, boosting economic growth and innovation. “The government’s upcoming reforms and policy updates, where it is expected to deliver on its ‘AI Opportunities Action Plan,’ [will no doubt aim] to speed up processes,” said Malaguti. “Public sector leaders need to be more strategic with their investments and approach these projects with a level head, rolling out a programme in a phased manner, considering each phase of their operations.” This sweeping transformation will also benefit from an expanded role for the Government Digital Service (GDS). Planned measures include using the GDS to identify cybersecurity vulnerabilities in public sector systems that could be exploited by hackers, enabling services to be made more robust and secure. Such reforms are critical to protect citizens, particularly as the reliance on digital solutions increases. The broader aim of these reforms is to modernise the ***’s public services to reflect the convenience and efficiencies demanded in a digital-first world. By using technologies like AI, the government hopes to make interactions with public services faster and more intuitive while saving billions for taxpayers in the long run. As technology reshapes the future of how services are delivered, leaders must ensure they are comprehensively addressing the root causes of inefficiency—primarily old data infrastructure and fragmented workflows. Only then can technological solutions, whether AI or otherwise, achieve their full potential in helping services deliver for the public. (Photo by Claudio Schwarz) See also: Biden’s executive order targets energy needs for AI data centres Want to learn more about AI and big data from industry leaders? Check out AI & Big Data Expo taking place in Amsterdam, California, and London. The comprehensive event is co-located with other leading events including Intelligent Automation Conference, BlockX, Digital Transformation Week, and Cyber Security & Cloud Expo. Explore other upcoming enterprise technology events and webinars powered by TechForge here. The post Rodolphe Malaguti, Conga: Poor data hinders AI in public services appeared first on AI News. View the full article
-
DeepSeek has unveiled its first-generation DeepSeek-R1 and DeepSeek-R1-Zero models that are designed to tackle complex reasoning tasks. DeepSeek-R1-Zero is trained solely through large-scale reinforcement learning (RL) without relying on supervised fine-tuning (SFT) as a preliminary step. According to DeepSeek, this approach has led to the natural emergence of “numerous powerful and interesting reasoning behaviours,” including self-verification, reflection, and the generation of extensive chains of thought (CoT). “Notably, [DeepSeek-R1-Zero] is the first open research to validate that reasoning capabilities of LLMs can be incentivised purely through RL, without the need for SFT,” DeepSeek researchers explained. This milestone not only underscores the model’s innovative foundations but also paves the way for RL-focused advancements in reasoning AI. However, DeepSeek-R1-Zero’s capabilities come with certain limitations. Key challenges include “endless repetition, poor readability, and language mixing,” which could pose significant hurdles in real-world applications. To address these shortcomings, DeepSeek developed its flagship model: DeepSeek-R1. Introducing DeepSeek-R1 DeepSeek-R1 builds upon its predecessor by incorporating cold-start data prior to RL training. This additional pre-training step enhances the model’s reasoning capabilities and resolves many of the limitations noted in DeepSeek-R1-Zero. Notably, DeepSeek-R1 achieves performance comparable to OpenAI’s much-lauded o1 system across mathematics, coding, and general reasoning tasks, cementing its place as a leading competitor. DeepSeek has chosen to open-source both DeepSeek-R1-Zero and DeepSeek-R1 along with six smaller distilled models. Among these, DeepSeek-R1-Distill-Qwen-32B has demonstrated exceptional results—even outperforming OpenAI’s o1-mini across multiple benchmarks. MATH-500 (Pass@1): DeepSeek-R1 achieved 97.3%, eclipsing OpenAI (96.4%) and other key competitors. LiveCodeBench (Pass@1-COT): The distilled version DeepSeek-R1-Distill-Qwen-32B scored 57.2%, a standout performance among smaller models. AIME 2024 (Pass@1): DeepSeek-R1 achieved 79.8%, setting an impressive standard in mathematical problem-solving. DeepSeek-R1 is here! Performance on par with OpenAI-o1 Fully open-source model & technical report MIT licensed: Distill & commercialize freely! Website & API are live now! Try DeepThink at [Hidden Content] today! 1/n pic.twitter.com/7BlpWAPu6y — DeepSeek (@deepseek_ai) January 20, 2025 A pipeline to benefit the wider industry DeepSeek has shared insights into its rigorous pipeline for reasoning model development, which integrates a combination of supervised fine-tuning and reinforcement learning. According to the company, the process involves two SFT stages to establish the foundational reasoning and non-reasoning abilities, as well as two RL stages tailored for discovering advanced reasoning patterns and aligning these capabilities with human preferences. “We believe the pipeline will benefit the industry by creating better models,” DeepSeek remarked, alluding to the potential of their methodology to inspire future advancements across the AI sector. One standout achievement of their RL-focused approach is the ability of DeepSeek-R1-Zero to execute intricate reasoning patterns without prior human instruction—a first for the open-source AI research community. Importance of distillation DeepSeek researchers also highlighted the importance of distillation—the process of transferring reasoning abilities from larger models to smaller, more efficient ones, a strategy that has unlocked performance gains even for smaller configurations. Smaller distilled iterations of DeepSeek-R1 – such as the 1.5B, 7B, and 14B versions – were able to hold their own in niche applications. The distilled models can outperform results achieved via RL training on models of comparable sizes. Bonus: Open-Source Distilled Models! Distilled from DeepSeek-R1, 6 small models fully open-sourced 32B & 70B models on par with OpenAI-o1-mini Empowering the open-source community Pushing the boundaries of **open AI**! 2/n pic.twitter.com/tfXLM2xtZZ — DeepSeek (@deepseek_ai) January 20, 2025 For researchers, these distilled models are available in configurations spanning from 1.5 billion to 70 billion parameters, supporting Qwen2.5 and Llama3 architectures. This flexibility empowers versatile usage across a wide range of tasks, from coding to natural language understanding. DeepSeek has adopted the MIT License for its repository and weights, extending permissions for commercial use and downstream modifications. Derivative works, such as using DeepSeek-R1 to train other large language models (LLMs), are permitted. However, users of specific distilled models should ensure compliance with the licences of the original base models, such as Apache 2.0 and Llama3 licences. (Photo by Prateek Katyal) See also: Microsoft advances materials discovery with MatterGen Want to learn more about AI and big data from industry leaders? Check out AI & Big Data Expo taking place in Amsterdam, California, and London. The comprehensive event is co-located with other leading events including Intelligent Automation Conference, BlockX, Digital Transformation Week, and Cyber Security & Cloud Expo. Explore other upcoming enterprise technology events and webinars powered by TechForge here. The post DeepSeek-R1 reasoning models rival OpenAI in performance appeared first on AI News. View the full article
-
The discovery of new materials is key to solving some of humanity’s biggest challenges. However, as highlighted by Microsoft, traditional methods of discovering new materials can feel like “finding a needle in a haystack.” Historically, finding new materials relied on laborious and costly trial-and-error experiments. More recently, computational screening of vast materials databases helped to speed up the process, but it remained a time-intensive process. Now, a powerful new generative AI tool from Microsoft could accelerate this process significantly. Dubbed MatterGen, the tool steps away from traditional screening methods and instead directly engineers novel materials based on design requirements, offering a potentially game-changing approach to materials discovery. Published in a paper in Nature, Microsoft describes MatterGen as a diffusion model that operates within the 3D geometry of materials. Where an image diffusion model might generate images from text prompts by tweaking pixel colours, MatterGen generates material structures by altering elements, positions, and periodic lattices in randomised structures. This bespoke architecture is designed specifically to handle the unique demands of materials science, such as periodicity and 3D arrangements. “MatterGen enables a new paradigm of generative AI-assisted materials design that allows for efficient exploration of materials, going beyond the limited set of known ones,” explains Microsoft. A leap beyond screening Traditional computational methods involve screening enormous databases of potential materials to identify candidates with desired properties. Yet, even these methods are limited in their ability to explore the universe of unknown materials and require researchers to sift through millions of options before finding promising candidates. In contrast, MatterGen starts from scratch—generating materials based on specific prompts about chemistry, mechanical attributes, electronic properties, magnetic behaviour, or combinations of these constraints. The model was trained using over 608,000 stable materials compiled from the Materials Project and Alexandria databases. In the comparison below, MatterGen significantly outperformed traditional screening methods in generating novel materials with specific properties—specifically a bulk modulus greater than 400 GPa, meaning they are hard to compress. While screening exhibited diminishing returns over time as its pool of known candidates became exhausted, MatterGen continued generating increasingly novel results. One common challenge encountered during materials synthesis is compositional disorder—the phenomenon where atoms randomly swap positions within a crystal lattice. Traditional algorithms often fail to distinguish between similar structures when deciding what counts as a “truly novel” material. To address this, Microsoft devised a new structure-matching algorithm that incorporates compositional disorder into its evaluations. The tool identifies whether two structures are merely ordered approximations of the same underlying disordered structure, enabling more robust definitions of novelty. Proving MatterGen works for materials discovery To prove MatterGen’s potential, Microsoft collaborated with researchers at Shenzhen Institutes of Advanced Technology (SIAT) – part of the ******** Academy of Sciences – to experimentally synthesise a novel material designed by the AI. The material, TaCr₂O₆, was generated by MatterGen to meet a bulk modulus target of 200 GPa. While the experimental result fell slightly short of the target, measuring a modulus of 169 GPa, the relative error was just 20%—a small discrepancy from an experimental perspective. Interestingly, the final material exhibited compositional disorder between Ta and Cr atoms, but its structure aligned closely with the model’s prediction. If this level of predictive accuracy can be translated to other domains, MatterGen could have a profound impact on material designs for batteries, fuel cells, magnets, and more. Today in @Nature: Our MatterGen model represents a paradigm shift in materials design, applying generative AI to create new compounds with specific properties with unprecedented precision. pic.twitter.com/RpnphXUY0c — Satya Nadella (@satyanadella) January 16, 2025 Microsoft positions MatterGen as a complementary tool to its previous AI model, MatterSim, which accelerates simulations of material properties. Together, the tools could serve as a technological “flywheel”, enhancing both the exploration of new materials and the simulation of their properties in iterative loops. This approach aligns with what Microsoft refers to as the “fifth paradigm of scientific discovery,” in which AI moves beyond pattern recognition to actively guide experiments and simulations. Microsoft has released MatterGen’s source code under the MIT licence. Alongside the code, the team has made the model’s training and fine-tuning datasets available to support further research and encourage broader adoption of this technology. Reflecting on generative AI’s broader scientific potential, Microsoft draws parallels to drug discovery, where such tools have already started transforming how researchers design and develop medicines. Similarly, MatterGen could reshape the way we approach materials design, particularly for critical domains such as renewable energy, electronics, and aerospace engineering. (Image credit: Microsoft) See also: L’Oréal: Making cosmetics sustainable with generative AI Want to learn more about AI and big data from industry leaders? Check out AI & Big Data Expo taking place in Amsterdam, California, and London. The comprehensive event is co-located with other leading events including Intelligent Automation Conference, BlockX, Digital Transformation Week, and Cyber Security & Cloud Expo. Explore other upcoming enterprise technology events and webinars powered by TechForge here. The post Microsoft advances materials discovery with MatterGen appeared first on AI News. View the full article
-
The US-China AI chip race has entered a new phase as ******** chip designer Cambricon Technologies reports its first-ever quarterly profit. The milestone emerges against a backdrop of escalating US export controls that have increasingly restricted ******** companies’ access to advanced semiconductor technology, particularly Nvidia’s sophisticated AI processors. Cambricon’s breakthrough into profitability signals a significant shift in the US-China AI chip race, transforming from a 2016 startup into China’s most valuable artificial intelligence company, now valued at approximately 300 billion yuan ($41 billion). While this represents only a fraction of Nvidia’s $3 trillion market capitalisation, it marks China’s growing capability to develop sophisticated AI chips domestically. The company’s financial turnaround is particularly noteworthy in the context of technological competition between the world’s two largest economies. After years of losses, Cambricon reported its first quarterly profit in the final quarter of 2024, with net profits ranging from 240 million yuan to 328 million yuan, despite posting a 724 million yuan loss in the first nine months. The market’s response to this shifting dynamic in the US-China AI chip race has been remarkable. Cambricon’s shares on the Shanghai Stock Exchange’s Star Market have surged more than 470% over the past year, climbing from 120.80 yuan to 695.96 yuan. The company projects a 70% revenue increase to 1.2 billion yuan in 2024, driven by China’s aggressive buildup of computing infrastructure to support its AI ambitions. At the technical level, Cambricon has positioned itself as China’s answer to US chip restrictions with its 7-nanometre AI chips. The company’s flagship Cambricon-1A processor has gained significant traction in the domestic market, particularly in products from major technology companies like Huawei Technologies. The stakes in the US-China AI chip race continue to rise, with analysts at Changjiang Securities projecting that China’s AI semiconductor market will reach 178 billion yuan by 2025. Beijing’s push for semiconductor self-sufficiency and increasing investments from domestic technology companies in AI infrastructure are fueling this growth. Recent US regulations announced in January 2025 have intensified the race, restricting ******** access to advanced AI technology and limiting it to American companies and their allies. In response, major ******** technology companies are investing heavily in domestic computing infrastructure. ByteDance, TikTok’s parent company, has committed 4.5 billion yuan to a new computing centre in Datong City, Shanxi province. This highlights the growing market opportunity for domestic chip manufacturers. While Cambricon’s progress represents a significant advancement in the US-China AI chip race, challenges remain. The company must continue to narrow the technological gap with international competitors while maintaining its growth trajectory. However, supportive government policies and growing domestic demand provide a favourable environment for continued development. Cambricon’s inclusion in the SSE 50 Index, which tracks the Shanghai Stock Exchange’s most valuable companies, underscores its strategic importance to China’s technology sector. As global tensions persist and access to foreign technology becomes more restricted, developing domestic AI chip capabilities has become increasingly important for China’s technological advancement and economic security. The post US-China AI chip race: Cambricon’s first profit lands appeared first on AI News. View the full article
-
L’Oréal will leverage IBM’s generative AI (GenAI) technology to create innovative and sustainable cosmetic products. The partnership will involve developing a bespoke AI foundation model to supercharge L’Oréal’s Research & Innovation (R&I) teams in creating eco-friendly formulations using renewable raw materials. In turn, this initiative is designed to reduce both energy and material waste. Described as the cosmetics industry’s first formulation-focused AI model, this effort is a glimpse into a future where cutting-edge technology drives environmentally-conscious solutions. Stéphane Ortiz, Head of Innovation Métiers & Product Development at L’Oréal R&I, said: “As part of our Digital Transformation Program, this partnership will extend the speed and scale of our innovation and reformulation pipeline, with products always reaching higher standards of inclusivity, sustainability, and personalisation.” AI and beauty: A perfect match By marrying L’Oréal’s expertise in cosmetic science with IBM’s AI technologies, the companies aim to unlock new pathways in both cosmetic innovation and sustainability. The role of AI in tailoring and personalising products is well-established, but diving deeper into its role in crafting renewable and sustainably-sourced formulations underscores a broader ecological mission. Matthieu Cassier, Chief Transformation & Digital Officer at L’Oréal R&I, commented: “Building on years of unique beauty science expertise and data structuring, this major alliance with IBM is opening a new exciting era for our innovation and development process.” Foundation models serve as the technological backbone for this collaboration. These AI systems are trained on vast datasets, enabling them to perform various tasks and transfer learnings across different applications. Although these models are perhaps most known for revolutionising natural language processing (NLP), IBM has advanced their use cases beyond text, including applications in chemistry, geospatial data, and time series analysis. In this context, the custom AI model being developed for L’Oréal will process a massive database of cosmetic formulas and raw material components. From creating new products to reformulating existing ones and scaling up for production, the model will accelerate critical tasks for the company’s R&D teams. “This collaboration is a truly impactful application of generative AI, leveraging the power of technology and expertise for the good of the planet,” said Alessandro Curioni, IBM Fellow and VP for Europe and Africa, as well as Director at IBM Research Zurich. “At IBM, we believe in the power of purpose-built, customised AI to help transform businesses. Using IBM’s latest AI technology, L’Oréal will be able to derive meaningful insights from their rich formula and product data to create a tailored AI model to help achieve their operational goals and continue creating high-quality and sustainable products.” One of the more fascinating dimensions of this collaboration is its potential to deepen understanding of renewable ingredient behaviour within cosmetic formulations. Guilhaume Leroy-Méline, IBM Distinguished Engineer and CTO of IBM Consulting France, said: “This alliance between highly specialised expertise in artificial intelligence and cosmetics seeks to revolutionise cosmetic formulation. It embodies the spirit of AI-augmented research, emphasising sustainability and diversity.” For IBM, this partnership reflects its broader strategy to extend AI applications into industries requiring bespoke solutions. As Curioni pointed out, custom AI has the potential to reshape businesses on multiple levels. By co-developing this bespoke formulation model, IBM and L’Oréal are setting the stage for a beauty industry that prizes both sustainability and cutting-edge innovation. If successful, the partnership could very well serve as a blueprint for other industries looking to bring AI’s transformative potential to bear on sustainability efforts. (Photo by Kelly Sikkema) See also: Cisco: Securing enterprises in the AI era Want to learn more about AI and big data from industry leaders? Check out AI & Big Data Expo taking place in Amsterdam, California, and London. The comprehensive event is co-located with other leading events including Intelligent Automation Conference, BlockX, Digital Transformation Week, and Cyber Security & Cloud Expo. Explore other upcoming enterprise technology events and webinars powered by TechForge here. The post L’Oréal: Making cosmetics sustainable with generative AI appeared first on AI News. View the full article
-
As AI becomes increasingly integral to business operations, new safety concerns and security threats emerge at an unprecedented pace—outstripping the capabilities of traditional cybersecurity solutions. The stakes are high with potentially significant repercussions. According to Cisco’s 2024 AI Readiness Index, only 29% of surveyed organisations feel fully equipped to detect and prevent unauthorised tampering with AI technologies. Continuous model validation DJ Sampath, Head of AI Software & Platform at Cisco, said: “When we talk about model validation, it is not just a one time thing, right? You’re doing the model validation on a continuous basis. “So as you see changes happen to the model – if you’re doing any type of finetuning, or you discover new attacks that are starting to show up that you need the models to learn from – we’re constantly learning all of that information and revalidating the model to see how these models are behaving under these new attacks that we’ve discovered. “The other very important point is that we have a really advanced threat research team which is constantly looking at these AI attacks and understanding how these attacks can further be enhanced. In fact, we’re, we’re, we’re contributing to the work groups inside of standards organisations like MITRE, OWASP, and NIST.” Beyond preventing harmful outputs, Cisco addresses the vulnerabilities of AI models to malicious external influences that can change their behaviour. These risks include prompt injection attacks, jailbreaking, and training data poisoning—each demanding stringent preventive measures. Evolution brings new complexities Frank Dickson, Group VP for Security & Trust at IDC, gave his take on the evolution of cybersecurity over time and what advancements in AI mean for the industry. “The first macro trend was that we moved from on-premise to the cloud and that introduced this whole host of new problem statements that we had to address. And then as applications move from monolithic to microservices, we saw this whole host of new problem sets. “AI and the addition of LLMs… same thing, whole host of new problem sets.” The complexities of AI security are heightened as applications become multi-model. Vulnerabilities can arise at various levels – from models to apps – implicating different stakeholders such as developers, end-users, and vendors. “Once an application moved from on-premise to the cloud, it kind of stayed there. Yes, we developed applications across multiple clouds, but once you put an application in AWS or Azure or GCP, you didn’t jump it across those various cloud environments monthly, quarterly, weekly, right? “Once you move from monolithic application development to microservices, you stay there. Once you put an application in Kubernetes, you don’t jump back into something else. “As you look to secure a LLM, the important thing to note is the model changes. And when we talk about model change, it’s not like it’s a revision … this week maybe [developers are] using Anthropic, next week they may be using Gemini. “They’re completely different and the threat vectors of each model are completely different. They all have their strengths and they all have their dramatic weaknesses.” Unlike conventional safety measures integrated into individual models, Cisco delivers controls for a multi-model environment through its newly-announced AI Defense. The solution is self-optimising, using Cisco’s proprietary machine learning algorithms to identify evolving AI safety and security concerns—informed by threat intelligence from Cisco Talos. Adjusting to the new normal Jeetu Patel, Executive VP and Chief Product Officer at Cisco, shared his view that major advancements in a short ******* of time always seem revolutionary but quickly feel normal. “Waymo is, you know, self-driving cars from Google. You get in, and there’s no one sitting in the car, and it takes you from point A to point B. It feels mind-bendingly amazing, like we are living in the future. The second time, you kind of get used to it. The third time, you start complaining about the seats. “Even how quickly we’ve gotten used to AI and ChatGPT over the course of the past couple years, I think what will happen is any major advancement will feel exceptionally progressive for a short ******* of time. Then there’s a normalisation that happens where everyone starts getting used to it.” Patel believes that normalisation will happen with AGI as well. However, he notes that “you cannot underestimate the progress that these models are starting to make” and, ultimately, the kind of use cases they are going to unlock. “No-one had thought that we would have a smartphone that’s gonna have more compute capacity than the mainframe computer at your fingertips and be able to do thousands of things on it at any point in time and now it’s just another way of life. My 14-year-old daughter doesn’t even think about it. “We ought to make sure that we as companies get adjusted to that very quickly.” See also: Sam Altman, OpenAI: ‘Lucky and humbling’ to work towards superintelligence Want to learn more about AI and big data from industry leaders? Check out AI & Big Data Expo taking place in Amsterdam, California, and London. The comprehensive event is co-located with other leading events including Intelligent Automation Conference, BlockX, Digital Transformation Week, and Cyber Security & Cloud Expo. Explore other upcoming enterprise technology events and webinars powered by TechForge here. The post Cisco: Securing enterprises in the AI era appeared first on AI News. View the full article
-
In the last days of office, President Joe Biden is taking steps to address the growing energy demands of advanced AI data centres. On Tuesday, he signed an executive order aimed at providing federal support to fast-track the development of infrastructure needed to power these AI DC facilities, the White House has announced. The order sets out a plan to lease federal land managed by the Defence and Energy departments for gigawatt-scale AI data centres and clean power projects. The goal is to meet the immense energy requirements of these facilities in a shorter timeframe. “The order will speed up how we build the next generation of AI infrastructure right here in America,” Biden said. He added that the initiative is designed to boost economic competitiveness, strengthen national security, promote clean energy, and ensure AI safety. One notable aspect of the order is its emphasis on American-made technology. Companies that use federal sites for AI data centres will be ordered to purchase a fair amount of US-made semiconductors. The specifics will be worked out for each project and is in line with the Biden administration’s overall aim to invest over $30 billion in domestic chip production. The administration is also working to streamline processes for building AI infrastructure. Federal agencies have been directed to expedite the granting of permits, improve connections to the electric grid, and advance transmission development around federal sites. The measures are intended to avoid delays that could hinder the rapid deployment of essential infrastructure. Tarun Chhabra, White House technology adviser, emphasised the urgency of the endeavour. “It’s absolutely essential to make sure the AI industry can build the infrastructure it needs for training and deploying powerful AI models right here in the US,” he told reporters. Chhabra noted that the demand for computing power and electricity is soaring, especially as AI developers work on advanced “frontier models,” the most powerful and resource-intensive AI systems available. He estimated that by 2028, some AI data centres could require as much as five gigawatts of capacity – enough energy to power millions of homes. The executive order also seeks to align AI development with environmental goals. Companies developing projects are urged to use sustainable energy and have minimal impact on electricity prices. The requirement reflects growing concerns about the environmental impact of AI data centres, which consume enormous amounts of energy, plus water for cooling. In a related move, the Commerce Department has tightened restrictions on AI chip and technology exports. The goal is to keep advanced computing capabilities in the US and allied nations while limiting access for competing countries. This complements the executive order’s focus on safeguarding national security by keeping cutting-edge AI models secure and based on US soil. Biden also gave a ******* picture of AI with regards to national security. Chhabra pointed out that AI systems being developed today already have capabilities that might be used for malicious purposes, like weapon development or cyberattacks. By securing domestic data centres, the US reduces the likelihood of adversaries gaining access to these powerful systems. The tech industry has reacted positively to the order. Major corporations like Microsoft, BlackRock, and SoftBank have announced significant investments in US-based AI infrastructure, aligning with the administration’s ambition for domestic technological leadership. By addressing energy demands, environmental considerations, and national security risks, Biden’s executive order lays the groundwork for a robust, secure, and sustainable AI infrastructure. It reflects the administration’s commitment to ensuring that the US remains a global leader in AI development and deployment. (Photo by Unsplash) See also: US-China tech war escalates with new AI chips export controls Want to learn more about AI and big data from industry leaders? Check out AI & Big Data Expo taking place in Amsterdam, California, and London. The comprehensive event is co-located with other leading events including Intelligent Automation Conference, BlockX, Digital Transformation Week, and Cyber Security & Cloud Expo. Explore other upcoming enterprise technology events and webinars powered by TechForge here. The post Biden’s executive order targets energy needs for AI data centres appeared first on AI News. View the full article
-
The race for AI video training has taken an unexpected turn. Major tech companies are now paying content creators thousands of dollars for their unused footage, marking a significant shift in how artificial intelligence companies acquire training data. In a revealing report from Bloomberg, tech giants including Google, OpenAI, and Moonvalley are actively seeking exclusive, unpublished video content from YouTubers and digital content creators to train AI algorithms. The move comes as companies compete to develop increasingly sophisticated AI video generators. The economics of the new market are fascinating. According to Bloomberg‘s findings, AI companies are willing to pay between $1 and $4 per minute for video footage, with rates varying based on quality and uniqueness. Premium content, such as 4K video footage, drone captures, and 3D animations, commands higher prices, while standard unused content from platforms like YouTube, Instagram, or TikTok typically sells for $1-2 per minute. Dan Levitt, senior vice president of creators at Wasserman, which represents prominent social media figures like YouTuber MatPat and fashion influencer Taylen Biggs, describes it as “an arms race” in which companies desperately need more footage. However, he adds a note of caution, in that this lucrative opportunity might be temporary, suggesting creators should act quickly to capitalise on current demand. The development of the market has given rise to specialised intermediaries. Companies like Troveo AI and Calliope Networks have emerged as third-party licensing facilitators, managing rights for thousands of hours of video footage owned by creators. These companies handle negotiations with content creators and bundle the content for AI companies, streamlining the process for both parties. Marty Pesis, co-founder and chief executive officer of Troveo, reveals that his company has already paid over $5 million to creators, highlighting the significant scale of the market. “All the companies building video models we’re either working with or are in our pipeline right now,” Pesis notes, underlining the widespread demand for training content. The arrangement offers a win-win situation for both parties. For content creators, it presents an opportunity to monetise footage that would otherwise remain unused. Many creators accumulate hundreds of hours of footage annually while producing content for various platforms, but only a fraction of their material makes it into a final, published video. The deals come with safeguards. Andrew Graham, head of digital corporate advisory and partnerships for Creative Artists Agency (CAA), explains that most agreements include specific terms preventing AI companies from creating digital replicas of content creators’ work or mimicking exact scenes from their channels. These protections ensure that creators’ brands and reputations remain intact while participating in AI video training. The development comes against a controversy surrounding AI companies’ use of online content. In 2024, several lawsuits were filed against major AI companies by news publishers, actors, and content creators, alleging unauthorised use of their intellectual property for AI training, be it for video, audio, text, or visual art. The new approach of directly licensing content represents a more structured and legal framework for acquiring training data. The trend also reflects a broader shift in the relationship between content creators and AI companies. Rather than having their public content scraped without compensation, creators now have the opportunity to participate actively in and benefit from AI development. As Levitt puts it, “This is a way to actually participate in that, to do this in a much more legal, structured manner, and now you at least have some upside.” For the AI industry, the development marks a step toward more ethical and transparent data collection practices. Companies like Moonvalley openly acknowledge their reliance on licensed content. The company states that the vast majority of its training data comes directly from content creators and filmmakers who choose to participate in licensing agreements. As AI video technology continues to evolve, this new market for unused footage may reshape how content creators approach their work, potentially influencing how they capture and store unused footage for future licensing opportunities. However, as Levitt suggests, creators interested in capitalising on this opportunity should act soon, as the window for such lucrative licensing deals may not remain open indefinitely. (Photo by Unsplash/Steven Van) See also: OpenAI: Copyrighted data ‘impossible’ to avoid for AI training Want to learn more about AI and big data from industry leaders? Check out AI & Big Data Expo taking place in Amsterdam, California, and London. The comprehensive event is co-located with other leading events including Intelligent Automation Conference, BlockX, Digital Transformation Week, and Cyber Security & Cloud Expo. Explore other upcoming enterprise technology events and webinars powered by TechForge here. The post AI giants pay thousands for creators’ unused footage to train models appeared first on AI News. View the full article
-
We all rely on Microsoft Office for productivity, in an office setting or at home. And while Microsoft does a great job when it comes to keeping Microsoft Office stable, there are situations when Office apps are not as fast as we might want. If Microsoft Office is running slowly for you, you’re probably not alone. However, there are certain optimisations and improvements you can make to speed up Office for Mac. Here are some of the most common methods to inject new life into your Office install: Reasons why Microsoft Office slows down Microsoft Office can end up running slowly because of one, or even all, of the following: Office files get corrupted and the application runs slowly as a result. A buildup of temporary files can cause a slowdown. Microsoft Office has not been updated. Updates can speed up the application. Documents that have DOC or DOCM extensions might be storing macros, which can lead to delays when opening documents. Lack of hardware resources on the device. Uninstall and re-install Microsoft Office As detailed in this article, one of the best methods to speed up Microsoft Office is to reinstall it, a process which will overwrite corrupted elements and/or replace any missing files. Fully deleting the app is important to create a fresh environment for the reinstallation. Go to the Applications folder and move the Microsoft Office folder to the Trash. Press Shift+Command+G to open “~/Library/Containers”. From the Group Containers folder, drag UBF8T346G9.OfficeOsfWebHost, ubf8t346g9.office and ubf8t346g9.ms to the Trash. Trash the following: com.microsoft.onenote.mac, com.microsoft.Word, com.microsoft.RMS-XPCService, com.microsoft.Powerpoint, com.microsoft.Outlook, com.microsoft.Excel and any other file that contains ‘.com.microsoft’ in its name. Use a Mac cleanup tool An alternative is to use a cleanup tool. Cleanup tools identify any remaining files or temporary files from apps and remove them. The advantage for less technically-skilled users is that the deletion of unwanted or unused files happens with just the press of a button. Cleanup tools can also uninstall apps. As we saw earlier, manually installing and then re-installing Office can be tricky to perform manually. With a cleanup tool, app removal is streamlined and automatic. The cleanup app removes files for you, saving time and ensuring all elements of Office are fully removed from your Mac. Update your Microsoft Office installation Microsoft releases plenty of updates for Microsoft Office, and, in general, it’s a good idea to keep apps updated. If you’re still using an older version of Office, it’s possibly one of the reasons for poor performance. Users are typically notified by the Microsoft Office Update tool whenever a new version is available, plus, there’s always the option of going to Microsoft’s website and downloading available updates manually. Manually delete temporary files Using a cleanup tool is the best option to find and delete temporary files. However, if you’re a confident Mac user, empty the contents of ‘~/Library/Caches’ into the Trash. Again, use Shift+Command+G to locate the folder. Reinstall an older version If after reinstalling Office and running all available updates, the problem persists, it may be worth using an older version of Office. Start with a version that’s only a month or two older than the original, and then progress to even older versions, testing the performance of the application as you go. The post Office for Mac running slowly? Here’s how to speed it up appeared first on AI News. View the full article
-
The Biden administration’s final major policy move landed this week with a significant impact on global AI, as it unveiled the most comprehensive AI chips export controls to date. This eleventh-hour decision, announced just days before the administration change, divides the world into AI computing haves and have-nots, with China squarely in the crosshairs of the most stringent restrictions imposed on artificial intelligence technology. “Artificial intelligence is quickly becoming central to security and economic strength,” the White House fact sheet declares, framing the controls as a decisive action “to ensure that US technology undergirds global AI use and that adversaries cannot easily abuse advanced AI.” The new AI chips export controls split the global technology landscape into three distinct tiers, fundamentally reshaping how nations can access and develop AI capabilities. Access to advanced AI processors remains unrestricted for 18 key allies, so-called tier-one nations, including Japan, Britain, and the Netherlands. However, the administration has implemented strict AI chips export quotas for other nations, creating a new global AI development hierarchy. The 18 allies possess “robust technology protection regimes and technology ecosystems aligned with the national security and foreign policy interests of the US,” the policy document states. For other countries, the restrictions impose precise limitations – chip orders maxing out at roughly 1,700 advanced GPUs can proceed without licences, primarily benefiting academic and research institutions. Impact on global AI development The reverberations through the AI industry were immediate. Nvidia, whose AI accelerators power many of the world’s most advanced artificial intelligence systems, saw its shares decline 2%. Vice President of Government Affairs Ned Finkle warned that the export curb “threatens to derail innovation and economic growth worldwide.” The stakes are exceptionally high for Nvidia, which derives 56% of its revenue from international markets. Cloud computing giants face a complex recalibration of their AI infrastructure. Under the new framework, US-headquartered providers must adopt a precise mathematical approach to their global operations: no more than 50% of their AI computing power can be deployed outside the country, with a maximum of 25% beyond tier-one countries, and just 7% in any single non-tier-one nation. US-China AI technology battle intensifies The timing and scope of these AI chip export controls reveal their primary target: China’s rapidly advancing AI capabilities. The White House document explicitly warns about “countries of concern” that “actively employ AI — including US-made AI” in ways that could “undermine US AI leadership.” With China accounting for 17% of Nvidia’s sales, the commercial impact aligns directly with the administration’s strategic goals. China’s Commerce Ministry’s swift response – promising to “take necessary measures to safeguard its legitimate rights and interests” – signals a new chapter in the technological cold war between the world’s leading AI powers. The restrictions specifically target China’s ability to develop advanced AI systems, particularly those that could enable “the development of weapons of mass destruction, supporting powerful offensive cyber operations, and aiding human rights abuses.” Global response and future implications The US’s European allies have raised concerns about the broad reach of the controls. EU Executive Vice-President Henna Virkkunen and Commissioner Maroš Šefčovič emphasized the need for continued access to advanced AI technology, stating they are “looking forward to engaging constructively with the next US administration” to maintain “a secure transatlantic supply chain on AI technology and supercomputers.” US National Security Adviser Jake Sullivan frames the controls within a broader technological revolution: “The US has to be prepared for rapid increases in AI’s capability in the coming years, which could have a transformative impact on the economy and our national security.” Set to take effect in 120 days, the AI chip export controls represent more than just Biden’s final policy move – they establish a new paradigm for global AI development. As former Trump administration national security official Meghan Harris notes, “How effective the rule ends up being in the next 10 to 15 years is now up to the incoming team.” The regulations mark a defining moment in both US-China relations and global AI development, creating boundaries and alliances that will shape the future of artificial intelligence well beyond the current administration. With these controls, Biden’s final act may be remembered as the moment that redefined the global AI technology landscape. See also: South Korea wants to develop 50 types of AI chips by 2030 Want to learn more about AI and big data from industry leaders? Check out AI & Big Data Expo taking place in Amsterdam, California, and London. The comprehensive event is co-located with other leading events including Intelligent Automation Conference, BlockX, Digital Transformation Week, and Cyber Security & Cloud Expo. Explore other upcoming enterprise technology events and webinars powered by TechForge here. The post US-China tech war escalates with new AI chips export controls appeared first on AI News. View the full article
-
AI is set to become a cornerstone of the ***’s vision for economic and societal renewal with a sweeping action plan unveiled today by Prime Minister Keir Starmer. The government has committed to all 50 recommendations outlined in the ambitious AI Opportunities Action Plan created by Matt Clifford CBE, tech entrepreneur and chair of the Advanced Research and Invention Agency. “Our plan will make Britain the world leader,” declared Starmer. “That means more jobs and investment in the ***, more money in people’s pockets, and transformed public services. That’s the change this government is delivering.” The plan positions AI as a key driver of national progress, aiming to revolutionise public services, boost productivity, and establish the *** as a global leader in the field. Chris Lehane, Chief Global Affairs Officer at OpenAI, said: “From the locomotive to the Colossus computer, the *** has a rich history of leadership in tech innovation and the research and development of AI. “The government’s AI action plan – led by the Prime Minister and Secretary Peter Kyle – recognises where AI development is headed and sets the *** on the right path to benefit from its growth. The *** has an enormous national resource in the talent of its people, institutions, and businesses which together can leverage AI to advance the country’s national interest.” A plan to unlock economic potential The economic benefits of AI adoption form a central tenet of the government’s strategy. The International Monetary Fund estimates that embracing AI could increase productivity by 1.5 percentage points annually. Over a decade, this could add up to £47 billion in economic gains per year, according to Treasury calculations. The strategy aims not only to grow the economy but to create tangible benefits for citizens. Dr Jean Innes, CEO of the Alan Turing Institute, said: “This plan offers an exciting route map, and we welcome its focus on adoption of safe and responsible AI, AI skills, and an ambition to sustain the ***’s global leadership, putting AI to work driving growth, and delivering benefits for society. “We share these ambitions and look forward to working with the government, universities, industry and civil society to shape the future of these technologies to support the ***’s success and improve people’s lives.” Three major companies – Vantage Data Centres, Nscale, and Kyndryl – have already pledged a combined £14 billion in investments, creating 13,250 jobs across the country and reinforcing confidence in the ***’s AI potential. This adds to the £25 billion in commitments secured at last year’s International Investment Summit. Vantage Data Centres is set to invest over £12 billion in *** data centre projects, including one of Europe’s largest data centre campuses in Wales. Meanwhile, Kyndryl will establish a new tech hub in Liverpool—creating up to 1,000 AI-related jobs. Nscale plans to back *** data centre infrastructure with a $2.5 billion investment, which includes the construction of the nation’s largest sovereign AI data centre in Essex by 2026. Alison Kay, VP for *** and Ireland at Amazon Web Services (AWS), said: “At AWS, we’ve seen first-hand the benefits that digital technologies like AI can bring, and that’s why we’ve announced plans to invest £8 billion over the next five years building, operating, and maintaining data centres in the ***. “By putting close industry collaboration and public-private partnership at the heart of the government’s agenda, every citizen, community and business in every region of the *** will have the opportunity to realise the benefits of AI, and thrive and grow.” Zahra Bahrololoumi CBE, CEO of Salesforce *** and Ireland, added: “Businesses in the *** are in a prime position to fully unlock the opportunities of AI, thanks to a strong innovation culture and risk-based laws. That is why Salesforce chose the *** as the location of its first-ever AI Centre, building on our commitment to invest $4 billion in our *** operations to harness the transformational power of AI.” Transforming public services AI is already being deployed within *** hospitals, helping to modernise the NHS by diagnosing conditions such as breast ******* more swiftly, improving care for non-verbal patients, and expediting patient discharges. “NHS datasets could be invaluable for impactful AI innovations in healthcare,” said Dr Bilal, Professor of Applied AI and Technology Ethics at Birmingham City University. “But they remain largely inaccessible to many researchers due to prohibitive costs and logistical hurdles.” “Initiatives like NHS Secure Data Environments are a great start but must be made more affordable, or ideally free, for academic institutions. Beyond healthcare, the government is betting that broader adoption of AI could achieve similar efficiencies in other public sectors. For instance, the plan highlights its potential to reduce administrative workloads for teachers, speed up planning consultations to facilitate new housing developments, and even identify potholes on roads via AI-enhanced cameras to expedite repairs. The new strategy introduces AI Growth Zones, designed to accelerate the development of critical infrastructure. These zones will not only expedite planning permissions but also ensure dedicated energy connections to power AI projects. The first such zone will be established in Culham, Oxfordshire. Building AI infrastructure Supporting the ***’s AI aspirations requires significant investment in infrastructure. The plan includes: A twentyfold increase in public compute capacity, with immediate work commencing on a new supercomputer to support AI advancements. The creation of a National Data Library, designed to safely unlock the potential of public data to fuel AI innovation. The establishment of a dedicated AI Energy Council, chaired by the Science and Energy Secretaries, to address the energy demands of AI development. This aligns with the government’s goal of becoming a clean energy superpower. A new government team tasked with developing the ***’s sovereign AI capabilities. The government also aims to provide stability for businesses by balancing the dynamism of the US and the regulatory guardrails seen in the EU. Science, Innovation, and Technology Secretary Peter Kyle said the *** would leverage its unique strengths: “We already have remarkable strengths we can tap into when it comes to AI—building our status as the cradle of computer science and intelligent machines and establishing ourselves as the third largest AI market in the world.” Reservations and risks with the AI action plan While the ambitious plan has been met with enthusiasm by many, industry experts caution against overlooking the potential risks posed by unregulated AI deployment. Dr Pia Hüsch, Research Fellow in Cyber, Technology and National Security at RUSI, commented: “Labour’s AI Opportunities Action Plan has economic growth as the top priority, shifting focus away from the previous government’s priorities around AI safety and regulation. “While the focus on investing in infrastructure such as computing power and a national data library is welcome, the *** Government must not forget risks posed by AI technologies or the international partnerships that are needed to secure long-term benefit from AI technologies.” Similarly, Deryck Mitchelson, Global Chief Information Security Officer at Check Point Software, expressed concerns about security and ethics: “AI integration without airtight security measures will only amplify these risks. External oversight of AI models and training datasets is essential—not optional. “We need built-in equality, ethics, and a transparent framework to measure outcomes and prove these systems genuinely enhance performance, not just cut costs.” Mitchelson warned that hasty deployment could erode public trust in AI-driven services and deepen inequality. He emphasised that the government must present this initiative as more than a cost-saving strategy and instead prioritise transparency, accountability, and robust safeguards. The AI Opportunities Action Plan is a key pillar of the government’s Industrial Strategy and the first stage of a forthcoming Digital and Technology Sector Plan. It also ties into the government’s broader Plan for Change, aiming to ensure AI’s benefits are felt across every corner of the ***. Chancellor Rachel Reeves MP underscored the initiative’s dual focus on economic growth and public service transformation: “AI is a powerful tool that will help grow our economy, make our public services more efficient and open up new opportunities to help improve living standards. “This action plan is the government’s modern industrial strategy in action.” As the *** accelerates efforts to harness AI, it faces the dual challenge of staying ahead in the global race for innovation while ensuring the potential pitfalls of the technology are minimised. Today’s announcements mark a bold step forward, but the road ahead will require careful navigation. See also: Sam Altman, OpenAI: ‘Lucky and humbling’ to work towards superintelligence Want to learn more about AI and big data from industry leaders? Check out AI & Big Data Expo taking place in Amsterdam, California, and London. The comprehensive event is co-located with other leading events including Intelligent Automation Conference, BlockX, Digital Transformation Week, and Cyber Security & Cloud Expo. Explore other upcoming enterprise technology events and webinars powered by TechForge here. The post *** Government signs off sweeping AI action plan appeared first on AI News. View the full article
-
The new year was only beginning, but technology had already taken centre stage in a tragic event that shocked many. Just outside the Trump International Hotel in Las Vegas, a Tesla Cybertruck erupted in an explosion, leaving one person dead and seven others with minor injuries. The devastating incident, confirmed by Las Vegas Sheriff Kevin McMahill, has sparked discussions about the role of artificial intelligence and its darker implications in today’s world. The Las Vegas Metro Police Department said that the truck’s bed had an alarming mix of gasoline canisters, camp fuel, and large firework mortars. Authorities believe these items were tied to a detonation system controlled by the driver, who appeared to have meticulously planned the attack. The combination of materials painted a chilling picture of a calculated and premeditated act. The driver, identified as 37-year-old Matthew Livelsberger, was an active-duty soldier in the US Army. Investigators found a “possible manifesto” saved on his phone, along with emails to a podcaster and other documents outlining his intentions. Surveillance footage revealed him preparing for the explosion by pouring fuel onto the truck at a stop before driving to the hotel. Despite all of the preparations, officials confirmed that Livelsberger had no prior criminal record and was not under surveillance at the time of the incident. One revelation drew significant public attention: Livelsberger had used ChatGPT to aid in his plans. Law enforcement reported that he queried the AI tool for information about assembling explosives, calculating how fast a round would need to be fired to detonate the materials, and understanding which legal loopholes might allow him to acquire the components. Sheriff McMahill addressed this unsettling development, stating, “We know AI was going to change the game for all of us at some point or another, in really all of our lives. I think this is the first incident that I’m aware of on US soil where ChatGPT is utilised to help an individual build a particular device.” Tragically, Livelsberger’s life ended at the scene with a self-inflicted gunshot wound. Authorities identified his body through DNA and tattoos due to the extensive burns he sustained in the explosion. OpenAI, the company behind ChatGPT, responded to the incident with a statement expressing their sorrow and emphasising their commitment to responsible AI use. “Our models are designed to refuse harmful instructions and minimise harmful content. In this case, ChatGPT responded with information already publicly available on the internet and provided warnings against harmful or ******** activities,” the statement read. OpenAI also confirmed their cooperation with law enforcement in the ongoing investigation. The explosion itself was described as a deflagration—a slower, less destructive reaction compared to a high-explosive detonation. Investigators suspect the muzzle flash from a gunshot may have ignited fuel vapours or fireworks fuses in the truck, triggering a chain reaction. Other possibilities, though, such as an electrical short, have not been ruled out. The Las Vegas explosion is a grim reminder of technology’s double-edged nature. While AI has enormous potential, its darker applications are forcing society to consider how to prevent such tragedies in the future. (Photo by Unsplash) See also: OpenAI: Musk wanted us to merge with Tesla or take ‘full control’ Want to learn more about AI and big data from industry leaders? Check out AI & Big Data Expo taking place in Amsterdam, California, and London. The comprehensive event is co-located with other leading events including Intelligent Automation Conference, BlockX, Digital Transformation Week, and Cyber Security & Cloud Expo. Explore other upcoming enterprise technology events and webinars powered by TechForge here. The post Driver used ChatGPT to plan attack, authorities reveal appeared first on AI News. View the full article
-
You’ve got a great idea for an AI-based application. Think of fine-tuning like teaching a pre-trained AI model a new trick. Sure, it already knows plenty from training on massive datasets, but you need to tweak it to your needs. For example, if you need it to pick up abnormalities in scans or figure out what your customers’ feedback really means. That’s where hyperparameters come in. Think of the large language model as your basic recipe and the hyperparameters as the spices you use to give your application its unique “flavour.” In this article, we’ll go through some basic hyperparameters and model tuning in general. What is fine-tuning? Imagine someone who’s great at painting landscapes deciding to switch to portraits. They understand the fundamentals – colour theory, brushwork, perspective – but now they need to adapt their skills to capture expressions and emotions. The challenge is teaching the model the new task while keeping its existing skills intact. You also don’t want it to get too ‘obsessed’ with the new data and miss the big picture. That’s where hyperparameter tuning saves the day. LLM fine-tuning helps LLMs specialise. It takes their broad knowledge and trains them to ace a specific task, using a much smaller dataset. Why hyperparameters matter in fine-tuning Hyperparameters are what separate ‘good enough’ models from truly great ones. If you push them too hard, the model can overfit or miss key solutions. If you go too easy, a model might never reach its full potential. Think of hyperparameter tuning as a type of business automation workflow. You’re talking to your model; you adjust, observe, and refine until it clicks. 7 key hyperparameters to know when fine-tuning Fine-turning success depends on tweaking a few important settings. This might sound complex, but the settings are logical. 1. Learning rate This controls how much the model changes its understanding during training. This type of hyperparameter optimisation is critical because if you as the operator… Go too fast, the model might skip past better solutions, Go too slow, it might feel like you’re watching paint dry – or worse, it gets stuck entirely. For fine-tuning, small, careful adjustments (rather like adjusting a light’s dimmer switch) usually do the trick. Here you want to strike the right balance between accuracy and speedy results. How you’ll determine the right mix depends on how well the model tuning is progressing. You’ll need to check periodically to see how it’s going. 2. Batch size This is how many data samples the model processes at once. When you’re using a hyper tweaks optimiser, you want to get the size just right, because… Larger batches are quick but might gloss over the details, Smaller batches are slow but thorough. Medium-sized batches might be the Goldilocks option – just right. Again, the best way to find the balonce is to carefully monitor the results before moving on to the next step. 3. Epochs An epoch is one complete run through your dataset. Pre-trained models already know quite a lot, so they don’t usually need as many epochs as models starting from scratch. How many epochs is right? Too many, and the model might start memorizing instead of learning (hello, overfitting), Too few, and it may not learn enough to be useful. 4. Dropout rate Think of this like forcing the model to get creative. You do this by turning off random parts of the model during training. It’s a great way to stop your model being over-reliant on specific pathways and getting lazy. Instead, it encourages the LLM to use more diverse problem-solving strategies. How do you get this right? The optimal dropout rate depends on how complicated your dataset is. A general rule of thumb is that you should match the dropout rate to the chance of outliers. So, for a medical diagnostic tool, it makes sense to use a higher dropout rate to improve the model’s accuracy. If you’re creating translation software, you might want to reduce the rate slightly to improve the training speed. 5. Weight decay This keeps the model from getting too attached to any one feature, which helps prevent overfitting. Think of it as a gentle reminder to ‘keep it simple.’ 6. Learning rate schedules This adjusts the learning rate over time. Usually, you start with bold, sweeping updates and taper off into fine-tuning mode – kind of like starting with broad strokes on a canvas and refining the details later. 7. Freezing and unfreezing layers Pre-trained models come with layers of knowledge. Freezing certain layers means you lock-in their existing learning, while unfreezing others lets them adapt to your new task. Whether you freeze or unfreeze depends on how similar the old and new tasks are. Common challenges to fine-tuning Fine tuning sounds great, but let’s not sugarcoat it – there are a few roadblocks you’ll probably hit: Overfitting: Small datasets make it easy for models to get lazy and memorise instead of generalise. You can keep this behaviour in check by using techniques like early stopping, weight decay, and dropout, Computational costs: Testing hyperparameters can seem like playing a game of whack-a-mole. It’s time-consuming and can be resource intensive. Worse yet, it’s something of a guessing game. You can use tools like Optuna or Ray Tune to automate some of the grunt work. Every task is different: There’s no one-size-fits-all approach. A technique that works well for one project could be disastrous for another. You’ll need to experiment. Tips to fine-tune AI models successfully Keep these tips in mind: Start with defaults: Check the recommended settings for any pre-trained models. Use them as a starting point or cheat sheet, Consider task similarity: If your new task is a close cousin to the original, make small tweaks and freeze most layers. If it’s a total 180 degree turn, let more layers adapt and use a moderate learning rate, Keep an eye on validation performance: Check how the model performs on a separate validation set to make sure it’s learning to generalise and not just memorising the training data. Start small: Run a test with a smaller dataset before you run the whole model through the training. It’s a quick way to catch mistakes before they snowball. Final thoughts Using hyperparameters make it easier for you to train your model. You’ll need to go through some trial and error, but the results make the effort worthwhile. When you get this right, the model excels at its task instead of just making a mediocre effort. The post The role of hyperparameters in fine-tuning AI models appeared first on AI News. View the full article
-
Plaintiffs in the case of Kadrey et al. vs. Meta have filed a motion alleging the firm knowingly used copyrighted works in the development of its AI models. The plaintiffs, which include author Richard Kadrey, filed their “Reply in Support of Plaintiffs’ Motion for Leave to File Third Amended Consolidated Complaint” in the United States District Court in the Northern District of California. The filing accuses Meta of systematically torrenting and stripping copyright management information (CMI) from pirated datasets, including works from the notorious shadow library LibGen. According to documents recently submitted to the court, evidence reveals highly incriminating practices involving Meta’s senior leaders. Plaintiffs allege that Meta CEO Mark Zuckerberg gave explicit approval for the use of the LibGen dataset, despite internal concerns raised by the company’s AI executives. A December 2024 memo from internal Meta discussions acknowledged LibGen as “a dataset we know to be pirated,” with debates arising about the ethical and legal ramifications of using such materials. Documents also revealed that top engineers hesitated to torrent the datasets, citing concerns about using corporate laptops for potentially unlawful activities. Additionally, internal communications suggest that after acquiring the LibGen dataset, Meta stripped CMI from the copyrighted works contained within—a practice that plaintiffs highlight as central to claims of copyright infringement. According to the deposition of Michael Clark – a corporate representative for Meta – the company implemented scripts designed to remove any information identifying these works as copyrighted, including keywords like “copyright,” “acknowledgements,” or lines commonly used in such texts. Clark attested that this practice was done intentionally to prepare the dataset for training Meta’s Llama AI models. “Doesn’t feel right” The allegations against Meta paint a portrait of a company knowingly partaking in a widespread piracy scheme facilitated through torrenting. According to a string of emails included as exhibits, Meta engineers expressed concerns about the optics of torrenting pirated datasets from within corporate spaces. One engineer noted that “torrenting from a [Meta-owned] corporate laptop doesn’t feel right,” but despite hesitation, the rapid downloading and distribution – or “seeding” – of pirated data took place. Legal counsel for the plaintiffs has stated that as late as January 2024, Meta had “already torrented (both downloaded and distributed) data from LibGen.” Moreover, records show that hundreds of related documents were initially obtained by Meta months prior but were withheld during early discovery processes. Plaintiffs argue this delayed disclosure amounts to bad-faith attempts by Meta to obstruct access to vital evidence. During a deposition on 17 December 2024, Zuckerberg himself reportedly admitted that such activities would raise “lots of red flags” and stated it “seems like a bad thing,” though he provided limited direct responses regarding Meta’s broader AI training practices. This case originally began as an intellectual property infringement action on behalf of authors and publishers claiming violations relating to AI use of their materials. However, the plaintiffs are now seeking to add two major claims to their suit: a violation of the Digital Millennium Copyright Act (DMCA) and a breach of the California Comprehensive Data Access and Fraud Act (CDAFA). Under the DMCA, the plaintiffs assert that Meta knowingly removed copyright protections to conceal unauthorised uses of copyrighted texts in its Llama models. As cited in the complaint, Meta allegedly stripped CMI “to reduce the chance that the models will memorise this data” and that this removal of rights management indicators made discovering the infringement more difficult for copyright holders. The CDAFA allegations involve Meta’s methods for obtaining the LibGen dataset, including allegedly engaging in torrenting to acquire copyrighted datasets without permission. Internal documentation shows Meta engineers openly discussed concerns that seeding and torrenting might prove to be “legally not ok.” Meta case may impact emerging legislation around AI development At the heart of this expanding legal battle lies growing concern over the intersection of copyright law and AI. Plaintiffs argue the stripping of copyright protections from textual datasets denies rightful compensation to copyright owners and allows Meta to build AI systems like Llama on the financial ruins of authors’ and publishers’ creative efforts. The timing of these allegations arises amidst heightened global scrutiny surrounding “generative AI” technologies. Companies like OpenAI, Google, and Meta have all come under fire regarding the use of copyrighted data to train their models. Courts across jurisdictions are currently grappling with the long-term impact of AI on rights management, with potentially landmark cases being decided in both the US and the ***. In this particular case, US courts have shown increasing willingness to hear complaints about AI’s potential harm to long-established copyright law precedents. Plaintiffs, in their motion, referred to The Intercept Media v. OpenAI, a recent decision from New York in which a similar DMCA claim was allowed to proceed. Meta continues to deny all allegations in the case and has yet to publicly respond to Zuckerberg’s reported deposition statements. Whether or not plaintiffs succeed in these amendments, authors across the world face growing anxieties about how their creative works are handled within the context of AI. With copyright law struggling to keep pace with technological advances, this case underscores the need for clearer guidance at an international level to protect both creators and innovators. For Meta, these claims also represent a reputational risk. As AI becomes the central focus of its future strategy, the allegations of reliance on pirated libraries are unlikely to help its ambitions of maintaining leadership in the field. The unfolding case of Kadrey et al. vs. Meta could have far-reaching ramifications for the development of AI models moving forward, potentially setting legal precedents in the US and beyond. (Photo by Amy Syiek) See also: *** wants to prove AI can modernise public services responsibly Want to learn more about AI and big data from industry leaders? Check out AI & Big Data Expo taking place in Amsterdam, California, and London. The comprehensive event is co-located with other leading events including Intelligent Automation Conference, BlockX, Digital Transformation Week, and Cyber Security & Cloud Expo. Explore other upcoming enterprise technology events and webinars powered by TechForge here. The post Meta accused of using pirated data for AI development appeared first on AI News. View the full article